使用Python语言写出遗传算法解决tsp问题
时间: 2024-11-04 13:14:59 浏览: 43
遗传算法是一种模拟自然选择过程的优化搜索技术,常用于求解复杂问题的全局最优解。针对旅行商问题(Traveling Salesman Problem, TSP),我们可以用遗传算法来寻找最短路径。以下是使用Python编写的一个简单的遗传算法解决方案:
```python
import random
import numpy as np
# 定义TSP问题的数据结构
def create_tsp_graph(graph_data):
nodes = list(range(len(graph_data)))
tsp = dict()
for node in nodes:
tsp[node] = [graph_data[node][other_node] for other_node in nodes if other_node != node]
return tsp
# 初始化种群
def initialize_population(population_size, graph):
population = []
for _ in range(population_size):
chromosome = [random.randint(0, len(graph) - 1) for _ in range(len(graph))]
population.append(chromosome)
return population
# 计算适应度(总距离)
def calculate_fitness(chromosome, graph):
total_distance = 0
for i in range(len(chromosome)):
total_distance += graph[chromosome[i]][chromosome[(i + 1) % len(chromosome)]]
return total_distance
# 交叉操作
def crossover(parent1, parent2):
point = random.randint(1, len(parent1) - 1)
offspring = parent1[:point] + parent2[point:]
return offspring
# 变异操作
def mutation(chromosome, mutation_rate):
for _ in range(len(chromosome)):
if random.random() < mutation_rate:
swap_index = random.randint(0, len(chromosome) - 1)
chromosome[swap_index], chromosome[swap_index + 1] = chromosome[swap_index + 1], chromosome[swap_index]
return chromosome
# 主遗传算法循环
def genetic_algorithm(graph, pop_size, num_generations, mutation_rate):
tsp_graph = create_tsp_graph(graph)
population = initialize_population(pop_size, tsp_graph)
for _ in range(num_generations):
fitness_scores = [calculate_fitness(c, tsp_graph) for c in population]
sorted_population = [p for _, p in sorted(zip(fitness_scores, population), key=lambda x: x[0])]
# 遗传操作
elite = sorted_population[:pop_size // 4] # 保留一部分精英个体
selected = random.sample(sorted_population, pop_size // 2) # 随机选择另一半
offspring = [crossover(p1, p2) for p1, p2 in zip(selected, selected)] # 交叉生成新个体
offspring = [mutation(o, mutation_rate) for o in offspring] # 变异操作
population = elite + offspring # 更新种群
best_solution = min(population, key=calculate_fitness)
return best_solution, calculate_fitness(best_solution, tsp_graph)
# 示例用法
if __name__ == "__main__":
graph_data = [[0, 10, 15, 20],
[10, 0, 35, 25],
[15, 35, 0, 30],
[20, 25, 30, 0]]
best_route, shortest_distance = genetic_algorithm(graph_data, 50, 100, 0.01)
print("Best route:", best_route)
print("Shortest distance:", shortest_distance)
阅读全文
相关推荐
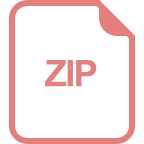
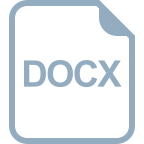
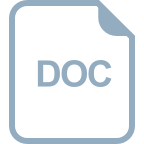
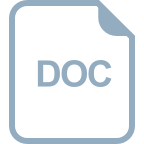

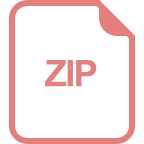
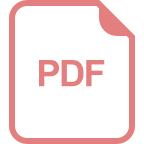
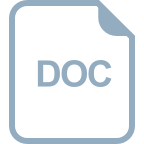
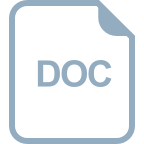
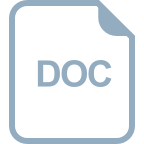
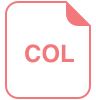
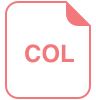
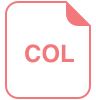
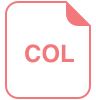
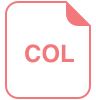
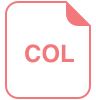
