详细解释这段代码 if status == 'train': if exploration: if args.action_enforcebound: normal = Normal(act_mean, act_std) x_t = normal.rsample() y_t = th.tanh(x_t) log_prob = normal.log_prob(x_t) # Enforcing Action Bound log_prob -= th.log(1 - y_t.pow(2) + 1e-6) actions = y_t return actions, log_prob
时间: 2024-02-10 07:20:36 浏览: 144
这段代码是一个条件语句,如果状态变量 `status` 的值等于字符串 `'train'`,那么会执行条件语句的内部代码块。
在内部代码块中,又有一个条件语句,如果变量 `exploration` 的值为真(即为 `True`),则会执行这个条件语句的内部代码块。
在内部代码块中,首先使用 `Normal` 函数生成一个服从正态分布的随机变量,并将这个随机变量的均值和标准差分别设置为变量 `act_mean` 和 `act_std` 的值。然后,使用 `rsample` 方法从这个分布中采样一个值,并将采样得到的值通过 `tanh` 函数进行激活。这个激活后的值被称为动作值,保存在变量 `y_t` 中。
接下来,计算这个随机变量的对数概率,即采样得到的值在这个分布中的概率的对数。这个对数概率值保存在变量 `log_prob` 中。
最后,为了确保生成的动作值在一定的范围内,使用 `tanh` 函数的性质,将其映射到区间 $[-1, 1]$ 上,并通过计算动作值的对数概率值来强制约束动作值的范围。强制约束后的动作值保存在变量 `actions` 中,并将其与对数概率值 `log_prob` 一起返回。
相关问题
class QLearningAgent: def __init__(self, state_size, action_size, learning_rate, discount_rate, exploration_rate): self.state_size = state_size self.action_size = action_size self.learning_rate = learning_rate self.discount_rate = discount_rate self.exploration_rate = exploration_rate self.q_table = np.zeros((state_size, action_size)) def act(self, state): if np.random.rand() < self.exploration_rate: return random.randrange(self.action_size) q_values = self.q_table[state] return np.argmax(q_values) def learn(self, state, action, reward, next_state, done): old_value = self.q_table[state, action] if done: td_target = reward else: next_max = np.max(self.q_table[next_state]) td_target = reward + self.discount_rate * next_max new_value = (1 - self.learning_rate) * old_value + self.learning_rate * td_target self.q_table[state, action] = new_value def set_exploration_rate(self, exploration_rate): self.exploration_rate = exploration_rate
这段代码是一个Q-learning智能体类,用于实现Q-learning算法。其中包括了初始化智能体、执行动作、学习过程等方法。在执行动作时,根据当前状态和探索率选择进行探索或者利用当前Q表中已有的知识进行动作选择。在学习过程中,根据当前状态、执行的动作、获得的奖励、下一个状态和是否结束来更新Q表中对应状态和动作的值。探索率和学习率都是可调节的超参数。
def train_model(stock_df, agent, num_episodes): for episode in range(num_episodes): obs = stock_df.iloc[0] state = get_state(obs) done = False total_reward = 0 while not done: action = agent.act(state) next_obs = stock_df.iloc[agent.current_step + 1] next_state = get_state(next_obs) reward = get_reward(action, obs, next_obs) total_reward += reward done = agent.current_step == len(stock_df) - 2 agent.learn(state, action, reward, next_state, done) state = next_state obs = next_obs # 输出每个episode的总奖励 print('Episode:', episode, 'Total Reward:', total_reward) # 逐渐降低探索率 agent.set_exploration_rate(agent.exploration_rate * 0.99)修改代码
这段代码中,训练模型时出现了错误,是因为 QLearningAgent 对象没有 current_step 属性,需要在 QLearningAgent 类中实现该属性。你可以在 QLearningAgent 类中添加 current_step 属性,并在 act 方法中更新该属性。例如:
```
class QLearningAgent:
def __init__(self, state_size, action_size):
self.state_size = state_size
self.action_size = action_size
self.q_table = np.zeros((state_size, action_size))
self.exploration_rate = 1.0
self.current_step = 0 # 新增 current_step 属性
def act(self, state):
if np.random.rand() < self.exploration_rate:
return np.random.choice(self.action_size)
q_values = self.q_table[state]
return np.argmax(q_values)
def learn(self, state, action, reward, next_state, done):
# 更新 Q 表
q_next = self.q_table[next_state]
if done:
q_next = np.zeros(self.action_size)
td_target = reward + GAMMA * np.max(q_next)
td_error = td_target - self.q_table[state, action]
self.q_table[state, action] += ALPHA * td_error
# 更新探索率和当前步数
self.current_step += 1
self.exploration_rate *= EXPLORATION_DECAY
self.exploration_rate = max(EXPLORATION_MIN, self.exploration_rate)
```
在修改后的代码中,我们在 QLearningAgent 类中新增了 current_step 属性,并在 act 方法和 learn 方法中更新该属性。最后,在训练模型时,我们可以使用 QLearningAgent 对象的 current_step 属性来获取当前步数,而不会再出现属性错误。
阅读全文
相关推荐




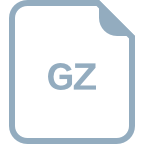








