fastapi部署pytorch模型
时间: 2024-09-26 07:00:56 浏览: 7
FastAPI是一种用于构建Web API的高效Python框架,而PyTorch是一个流行的机器学习库,常用于深度学习。将PyTorch模型部署到FastAPI中主要是为了让训练好的模型能够通过RESTful API的形式提供服务。以下是基本步骤:
1. **保存模型**: 首先,在训练完成后,你需要将PyTorch模型保存为文件,通常是使用`torch.save()`函数,并指定`.pt`或`.pth`等格式。
```python
model = ... # your PyTorch model
torch.save(model.state_dict(), "model.pth")
```
2. **加载模型**: 创建一个简单的FastAPI应用,然后在启动时加载模型。你可以使用`torch.load()`在运行时从文件加载模型。
```python
from fastapi import FastAPI
import torch
app = FastAPI()
@app.on_event("startup")
async def load_model():
model = torch.load("model.pth", map_location=torch.device('cuda' if torch.cuda.is_available() else 'cpu'))
app.state.model = model
```
3. **定义API端点**: 定义一个接收输入数据并使用模型预测的API。例如,如果你的模型接受图像作为输入,你可以创建一个处理POST请求的端点。
```python
from PIL import Image
import io
@app.post("/predict")
async def predict(image: bytes):
img = Image.open(io.BytesIO(image))
input_tensor = preprocess_image(img) # Your preprocessing function
output = app.state.model(input_tensor)
return {"prediction": output.tolist()}
```
4. **运行服务器**: 使用FastAPI的内置服务器或外部WSGI服务器(如Uvicorn、gunicorn)运行应用程序。
```shell
uvicorn main:app --reload
```
**注意事项:**
- 您需要预先编写预处理和后处理函数(preprocess_image 和 postprocess_output),它们负责将原始数据转换成模型期望的格式,并将模型输出转回用户可以理解的形式。
- 根据需求调整设备定位(map_location),如果是CPU部署,则不需要设置cuda。
相关推荐
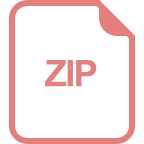
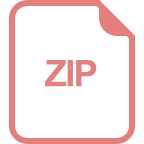
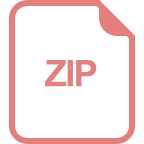














