解释代码 blob = cv2.dnn.blobFromImage(img_input, 1 / 255.0, (input_width, input_height), crop=False)
时间: 2023-06-11 18:05:42 浏览: 89
这行代码是使用OpenCV中的深度学习模块(dnn)从输入图片(img_input)中生成一个blob对象。Blob对象是多维数组,通常用于神经网络的数据输入。在这个函数中,我们将图片大小缩放为指定的输入宽度(input_width)和输入高度(input_height),并将像素值归一化到0到1的范围内。crop参数指定是否进行裁剪,如果为True,则会将图像中心裁剪成指定的尺寸,如果为False,则会按比例调整图像大小以适应指定的输入尺寸。
相关问题
解释代码 blob = cv2.dnn.blobFromImage(img_input, 1 / 255.0, (input_width, input_height), crop=False)
这段代码是使用 OpenCV 中的深度学习模块 dnn,用于将输入的图像 img_input 转换为深度学习模型可接受的输入格式。其中,1 / 255.0 表示对图像进行归一化处理,(input_width, input_height) 是所需的图像大小,crop=False 表示输入图像不需要进行裁剪。最终,生成的 blob 对象会被输入到深度学习模型中进行识别任务。
c程序求sn=a+aa+aaa+...+的值 循环结构写
使用现成的yolo8模型可以通过以下步骤实现:
1. 下载并安装yolo8模型,可以从官方网站或Github上获取。
2. 准备测试数据集,包括图片或视频。
3. 在终端或命令行中输入命令,加载yolo8模型并对测试数据集进行测试。
4. 可以根据需要进行参数调整和优化,以获得更好的结果。
以下是一个简单的Python代码示例,用于加载yolo8模型并进行测试:
```
import cv2
import numpy as np
# 加载yolo8模型
net = cv2.dnn.readNet("yolo8.weights", "yolo8.cfg")
# 设置类别名称和颜色
classes = []
with open("coco.names", "r") as f:
classes = [line.strip() for line in f.readlines()]
colors = np.random.uniform(0, 255, size=(len(classes), 3))
# 加载测试数据集
img = cv2.imread("test.jpg")
height, width, channels = img.shape
# 预处理图像
blob = cv2.dnn.blobFromImage(img, 1/255.0, (416, 416), swapRB=True, crop=False)
# 设置输入和输出层
net.setInput(blob)
output_layers = net.getUnconnectedOutLayersNames()
# 运行模型
outputs = net.forward(output_layers)
# 解析输出
boxes = []
confidences = []
class_ids = []
for output in outputs:
for detection in output:
scores = detection[5:]
class_id = np.argmax(scores)
confidence = scores[class_id]
if confidence > 0.5:
center_x = int(detection[0] * width)
center_y = int(detection[1] * height)
w = int(detection[2] * width)
h = int(detection[3] * height)
x = center_x - w // 2
y = center_y - h // 2
boxes.append([x, y, w, h])
confidences.append(float(confidence))
class_ids.append(class_id)
# 非极大值抑制
indexes = cv2.dnn.NMSBoxes(boxes, confidences, 0.5, 0.4)
# 绘制边界框和标签
for i in range(len(boxes)):
if i in indexes:
x, y, w, h = boxes[i]
label = str(classes[class_ids[i]])
color = colors[class_ids[i]]
cv2.rectangle(img, (x, y), (x + w, y + h), color, 2)
cv2.putText(img, label, (x, y - 10), cv2.FONT_HERSHEY_SIMPLEX, 0.5, color, 2)
# 显示结果
cv2.imshow("Image", img)
cv2.waitKey(0)
cv2.destroyAllWindows()
```
在以上示例中,我们首先加载了yolo8模型和类别名称,然后读取测试数据集(这里是一张图片),并将其预处理成模型需要的格式。接着,我们将预处理后的图像输入到模型中,并获取输出层。然后,我们通过解析输出,得到预测的边界框、置信度和类别信息,并进行非极大值抑制处理,最后将结果绘制到原始图像上并显示出来。
注意:以上示例仅供参考,具体实现需要根据实际情况进行调整和优化。
相关推荐
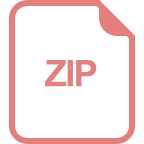
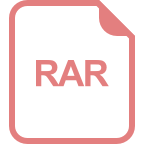













