没有标签的样本怎么送进1d-CNN回归神经网络进行测试,请写出pytorch代码
时间: 2024-02-24 07:53:46 浏览: 29
如果没有标签的样本,我们无法进行模型的监督学习和测试。因此,需要先准备一些有标签的样本用于模型的训练和测试,再使用模型对没有标签的样本进行预测。
以下是使用 PyTorch 实现 1D-CNN 回归神经网络进行测试的代码:
```python
import torch
import torch.nn as nn
# 定义 1D-CNN 回归神经网络模型
class Net(nn.Module):
def __init__(self):
super(Net, self).__init__()
self.conv1 = nn.Conv1d(in_channels=1, out_channels=16, kernel_size=3, padding=1)
self.pool1 = nn.MaxPool1d(kernel_size=2)
self.conv2 = nn.Conv1d(in_channels=16, out_channels=32, kernel_size=3, padding=1)
self.pool2 = nn.MaxPool1d(kernel_size=2)
self.fc1 = nn.Linear(32 * 25, 128)
self.fc2 = nn.Linear(128, 1)
def forward(self, x):
x = self.pool1(torch.relu(self.conv1(x)))
x = self.pool2(torch.relu(self.conv2(x)))
x = x.view(-1, 32 * 25)
x = torch.relu(self.fc1(x))
x = self.fc2(x)
return x
# 加载模型
model = Net()
model.load_state_dict(torch.load('model.pth'))
# 加载数据集
test_data = torch.Tensor(test_data) # test_data 是没有标签的样本数据,需先准备好
test_loader = torch.utils.data.DataLoader(test_data, batch_size=64, shuffle=False)
# 使用模型进行测试
model.eval()
predictions = []
with torch.no_grad():
for data in test_loader:
output = model(data.unsqueeze(1))
predictions.append(output.numpy())
# 打印预测结果
print(predictions)
```
其中,`test_data` 是没有标签的样本数据,需要先准备好。`model.pth` 是已经训练好的模型参数文件。使用 `model.eval()` 将模型设置为测试模式,使用 `torch.no_grad()` 禁用梯度计算,可以加快推理速度。最后,将预测结果 `predictions` 打印出来。
相关推荐
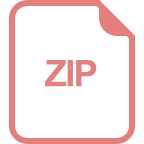
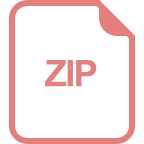
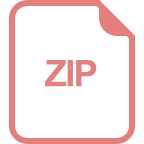














