def generate(self, obs, all=False): good_pts = [] good_scores = [] pts = [] scores = [] dir_set = [(1, 0), (1, -1), (0, -1), (-1, -1), (-1, 0), (-1, 1), (0, 1), (1, 1)] if all: indices = np.where(obs) check_list = [(indices[0][i], indices[1][i]) for i in range(len(indices[0]))] else: if len(self._last_move_list) > 7: check_list = self._last_move_list[-7:] else: check_list = self._last_move_list for x0, y0 in check_list: for dir in dir_set: if x0 + dir[0] in range(0, 15) and y0 + dir[1] in range(0, 15): pos = (x0 + dir[0], y0 + dir[1]) if obs[pos[0]][pos[1]] == 0 and pos not in pts: obs[pos[0]][pos[1]] = self.color score_atk = self.evaluate_point(obs, pos) obs[pos[0]][pos[1]] = -self.color score_def = self.evaluate_point(obs, pos) score = max(score_atk, score_def) if score >= score_3_live: good_pts.append(pos) good_scores.append(score) if score_atk == score_5: break pts.append(pos) scores.append(score) obs[pos[0]][pos[1]] = 0 if len(good_pts) > 0 and max(good_scores) >= score_4: # print('good') pts = good_pts scores = good_scores lst = np.array([pts, scores]) pts = lst[:, lst[1].argsort()][0] pos_list = list(pts) pos_list.reverse() return pos_list
时间: 2023-06-18 11:04:07 浏览: 150
这段代码是一个五子棋AI的 generate 函数实现。该函数根据当前的观察数据 obs,生成AI下一步应该下的位置 pos_list。
首先,该函数会生成一个方向集合 dir_set,包含了八个方向。然后,如果参数 all 为 True,则遍历整个棋盘;否则,只遍历最近的七个落子位置。
接下来,对于每个遍历到的位置,都会向八个方向扩展,找到空位 pos。然后,分别计算在 pos 位置下子后的攻击分数 score_atk 和防御分数 score_def,取两者中的最大值作为该位置的得分 score。如果 score 大于等于特定的分数 score_3_live,则将该位置添加到 pts 和 scores 列表中。
如果存在得分大于等于特定值 score_4 的位置,则将这些位置作为好的位置 good_pts,并将它们的得分添加到 good_scores 列表中。如果存在得分为特定值 score_5 的位置,则直接选取这些位置。
最后,将 pts 和 scores 的元素按照 scores 从小到大进行排序,返回 pos_list。
相关问题
def fit(self, obs): self.obs = obs self.n_features = self.obs.shape[1] startprob = np.random.uniform(self.lb, self.ub, size=self.n_states) startprob /= np.sum(startprob) self.model = GaussianHMM(n_components=self.n_states, startprob_prior=startprob,covariance_type='spherical', n_iter=1000) self.model.fit(obs) self.sparrows = [self.generate_random_params() for _ in range(self.n_sparrows)] self.sparrows /= np.sum(self.sparrows) self.scores = [self.calculate_score(p) for p in self.sparrows] for i in range(self.n_iter): for j in range(self.n_sparrows): # 移动 params = self.sparrows[j] params += np.random.uniform(self.lb, self.ub, size=params.shape) params = np.clip(params, self.lb, self.ub) # 变异 params = self.mutate(params) # 计算分数 score = self.calculate_score(params) score = int(score) # 更新最优解 if score > self.best_score: self.best_score = score self.best_params = params # 更新麻雀群体 if score > self.scores[j]: self.sparrows[j] = params self.scores[j] = score
这段代码中出现了一些术语,可以帮我理解一下吗?
- GaussianHMM:高斯隐马尔可夫模型,是一种用于处理时间序列数据的统计模型,通常用于识别和预测序列中的模式和趋势。
- n_components:表示隐状态的数量,即模型中的状态数。
- startprob_prior:表示每个隐状态的先验概率。
- covariance_type:表示协方差矩阵的类型,可以是对角矩阵、球状协方差矩阵或完整协方差矩阵。
- n_iter:表示训练模型时迭代的次数。
- sparrows:表示麻雀群体,是一种基于鸟群行为的优化算法。
- mutate:表示变异操作,是优化算法中的一种操作,包括对参数进行随机扰动或基于其他参数进行变换,以便生成新的解。
- best_score:表示最优解的得分,即当前已发现的最好的参数组合的分数。
- best_params:表示最优解的参数组合,即当前已发现的最好的参数组合。
- lb和ub:表示参数的下限和上限,用于约束参数的取值范围。
阅读全文
相关推荐
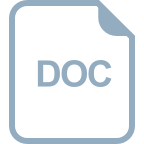
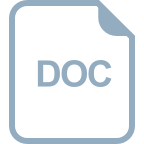
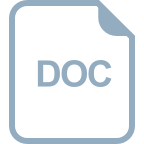
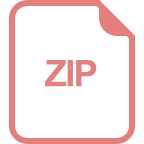
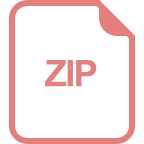
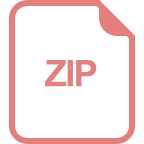
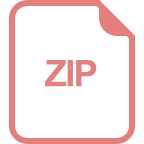
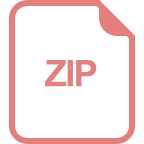
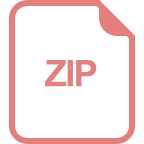
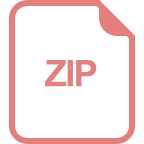
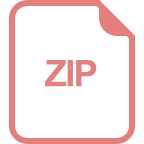
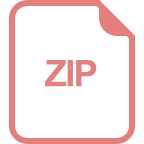
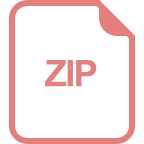
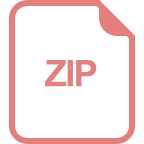
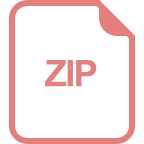
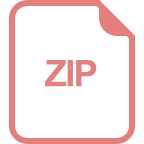
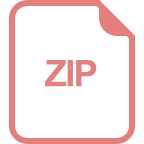
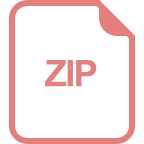