解释data_title = data1.columns.values.tolist() r = [] for i in range(len(data_title)): for j in range(i+1,len(data_title)): print('%s和%s之间的皮尔逊相关系数为%f'%(data_title[i],data_title[j],pearsonr(data1[data_title[i]],data1[data_title[j]])[0])) r.append(pearsonr(data[data_title[i]],data[data_title[j]])[0])
时间: 2023-11-28 08:06:20 浏览: 53
这段代码的作用是计算给定数据集中各列之间的皮尔逊相关系数,并将结果存储在一个列表r中。
具体实现流程如下:
1. 将数据集中的列名存储在一个列表data_title中。
2. 初始化一个空列表r,用于存储计算得到的皮尔逊相关系数。
3. 使用两层循环遍历data_title列表中的每一对列,计算它们之间的皮尔逊相关系数,并将结果打印出来。
4. 将计算得到的皮尔逊相关系数存储在列表r中,并在循环结束后返回该列表。
需要注意的是,该代码使用了Python中的pearsonr函数来计算皮尔逊相关系数。此函数需要两个一维数组作为输入,并返回一个元组,其中第一个元素为相关系数,第二个元素为p-value。因此,代码中需要使用[0]来提取相关系数。
相关问题
将上述代码放入了Recommenders.py文件中,作为一个自定义工具包。将下列代码中调用scipy包中svd的部分。转为使用Recommenders.py工具包中封装的svd方法。给出修改后的完整代码。import pandas as pd import math as mt import numpy as np from sklearn.model_selection import train_test_split from Recommenders import * from scipy.sparse.linalg import svds from scipy.sparse import coo_matrix from scipy.sparse import csc_matrix # Load and preprocess data triplet_dataset_sub_song_merged = triplet_dataset_sub_song_mergedpd # load dataset triplet_dataset_sub_song_merged_sum_df = triplet_dataset_sub_song_merged[['user','listen_count']].groupby('user').sum().reset_index() triplet_dataset_sub_song_merged_sum_df.rename(columns={'listen_count':'total_listen_count'},inplace=True) triplet_dataset_sub_song_merged = pd.merge(triplet_dataset_sub_song_merged,triplet_dataset_sub_song_merged_sum_df) triplet_dataset_sub_song_merged['fractional_play_count'] = triplet_dataset_sub_song_merged['listen_count']/triplet_dataset_sub_song_merged['total_listen_count'] # Convert data to sparse matrix format small_set = triplet_dataset_sub_song_merged user_codes = small_set.user.drop_duplicates().reset_index() song_codes = small_set.song.drop_duplicates().reset_index() user_codes.rename(columns={'index':'user_index'}, inplace=True) song_codes.rename(columns={'index':'song_index'}, inplace=True) song_codes['so_index_value'] = list(song_codes.index) user_codes['us_index_value'] = list(user_codes.index) small_set = pd.merge(small_set,song_codes,how='left') small_set = pd.merge(small_set,user_codes,how='left') mat_candidate = small_set[['us_index_value','so_index_value','fractional_play_count']] data_array = mat_candidate.fractional_play_count.values row_array = mat_candidate.us_index_value.values col_array = mat_candidate.so_index_value.values data_sparse = coo_matrix((data_array, (row_array, col_array)),dtype=float) # Compute SVD def compute_svd(urm, K): U, s, Vt = svds(urm, K) dim = (len(s), len(s)) S = np.zeros(dim, dtype=np.float32) for i in range(0, len(s)): S[i,i] = mt.sqrt(s[i]) U = csc_matrix(U, dtype=np.float32) S = csc_matrix(S, dtype=np.float32) Vt = csc_matrix(Vt, dtype=np.float32) return U, S, Vt def compute_estimated_matrix(urm, U, S, Vt, uTest, K, test): rightTerm = S*Vt max_recommendation = 10 estimatedRatings = np.zeros(shape=(MAX_UID, MAX_PID), dtype=np.float16) recomendRatings = np.zeros(shape=(MAX_UID,max_recommendation ), dtype=np.float16) for userTest in uTest: prod = U[userTest, :]*rightTerm estimatedRatings[userTest, :] = prod.todense() recomendRatings[userTest, :] = (-estimatedRatings[userTest, :]).argsort()[:max_recommendation] return recomendRatings K=50 # number of factors urm = data_sparse MAX_PID = urm.shape[1] MAX_UID = urm.shape[0] U, S, Vt = compute_svd(urm, K) # Compute recommendations for test users # Compute recommendations for test users uTest = [1,6,7,8,23] uTest_recommended_items = compute_estimated_matrix(urm, U, S, Vt, uTest, K, True) # Output recommended songs in a dataframe recommendations = pd.DataFrame(columns=['user','song', 'score','rank']) for user in uTest: rank = 1 for song_index in uTest_recommended_items[user, 0:10]: song = small_set.loc[small_set['so_index_value'] == song_index].iloc[0] # Get song details recommendations = recommendations.append({'user': user, 'song': song['title'], 'score': song['fractional_play_count'], 'rank': rank}, ignore_index=True) rank += 1 display(recommendations)
import pandas as pd
import math as mt
import numpy as np
from sklearn.model_selection import train_test_split
from Recommenders import SVDRecommender #import the SVDRecommender class from our custom package
# Load and preprocess data
triplet_dataset_sub_song_merged = triplet_dataset_sub_song_mergedpd
# load dataset
triplet_dataset_sub_song_merged_sum_df = triplet_dataset_sub_song_merged[['user','listen_count']].groupby('user').sum().reset_index()
triplet_dataset_sub_song_merged_sum_df.rename(columns={'listen_count':'total_listen_count'},inplace=True)
triplet_dataset_sub_song_merged = pd.merge(triplet_dataset_sub_song_merged,triplet_dataset_sub_song_merged_sum_df)
triplet_dataset_sub_song_merged['fractional_play_count'] = triplet_dataset_sub_song_merged['listen_count']/triplet_dataset_sub_song_merged['total_listen_count']
# Convert data to sparse matrix format
small_set = triplet_dataset_sub_song_merged
user_codes = small_set.user.drop_duplicates().reset_index()
song_codes = small_set.song.drop_duplicates().reset_index()
user_codes.rename(columns={'index':'user_index'}, inplace=True)
song_codes.rename(columns={'index':'song_index'}, inplace=True)
song_codes['so_index_value'] = list(song_codes.index)
user_codes['us_index_value'] = list(user_codes.index)
small_set = pd.merge(small_set,song_codes,how='left')
small_set = pd.merge(small_set,user_codes,how='left')
mat_candidate = small_set[['us_index_value','so_index_value','fractional_play_count']]
data_array = mat_candidate.fractional_play_count.values
row_array = mat_candidate.us_index_value.values
col_array = mat_candidate.so_index_value.values
data_sparse = coo_matrix((data_array, (row_array, col_array)),dtype=float)
# Compute SVD using our custom package
K=50 # number of factors
urm = data_sparse
MAX_PID = urm.shape[1]
MAX_UID = urm.shape[0]
recommender = SVDRecommender(K)
U, S, Vt = recommender.fit(urm)
# Compute recommendations for test users
uTest = [1,6,7,8,23]
uTest_recommended_items = recommender.recommend(uTest, urm, 10)
# Output recommended songs in a dataframe
recommendations = pd.DataFrame(columns=['user','song', 'score','rank'])
for user in uTest:
rank = 1
for song_index in uTest_recommended_items[user, 0:10]:
song = small_set.loc[small_set['so_index_value'] == song_index].iloc[0] # Get song details
recommendations = recommendations.append({'user': user, 'song': song['title'], 'score': song['fractional_play_count'], 'rank': rank}, ignore_index=True)
rank += 1
display(recommendations)
import pandas as pd import numpy as np import matplotlib.pyplot as plt import jieba import requests import re from io import BytesIO import imageio # 设置城市和时间 city = '上海' year = 2021 quarter = 2 # 爬取数据 url = f'http://tianqi.2345.com/t/wea_history/js/{city}/{year}/{quarter}.js' response = requests.get(url) text = response.content.decode('gbk') # 正则表达式匹配 pattern = re.compile(r'(\d{4}-\d{2}-\d{2})\|(\d{1,2})\|(\d{1,2})\|(\d{1,3})\|(\d{1,3})\|(\D+)\n') result = pattern.findall(text) # 数据整理 data = pd.DataFrame(result, columns=['日期', '最高温度', '最低温度', '空气质量指数', '风力等级', '天气']) data[['最高温度', '最低温度', '空气质量指数', '风力等级']] = data[['最高温度', '最低温度', '空气质量指数', '风力等级']].astype(int) data['日期'] = pd.to_datetime(data['日期']) # 可视化分析 # 统计天气情况 weather_count = data['天气'].value_counts() weather_count = weather_count[:10] # 分词统计 seg_list = jieba.cut(' '.join(data['天气'].tolist())) words = {} for word in seg_list: if len(word) < 2: continue if word in words: words[word] += 1 else: words[word] = 1 # 绘制柱状图和词云图 plt.figure(figsize=(10, 5)) plt.bar(weather_count.index, weather_count.values) plt.title(f'{city}{year}年第{quarter}季度天气情况') plt.xlabel('天气') plt.ylabel('次数') plt.savefig('weather_bar.png') wordcloud = pd.DataFrame(list(words.items()), columns=['word', 'count']) mask_image = imageio.imread('cloud_mask.png') wordcloud.plot(kind='scatter', x='count', y='count', alpha=0.5, s=300, cmap='Reds', figsize=(10, 5)) for i in range(len(wordcloud)): plt.text(wordcloud.iloc[i]['count'], wordcloud.iloc[i]['count'], wordcloud.iloc[i]['word'], ha='center', va='center', fontproperties='SimHei') plt.axis('off') plt.imshow(mask_image, cmap=plt.cm.gray, interpolation='bilinear') plt.savefig('weather_wordcloud.png')这个python代码有错误,请改正以使该代码运行成功
这段代码的问题在于缺少了一些必要的包和文件,比如 cloud_mask.png,同时也需要安装 jieba 和 imageio 这两个包。以下是修改后的代码:
```
import pandas as pd
import numpy as np
import matplotlib.pyplot as plt
import jieba
import requests
import re
from io import BytesIO
import imageio
# 设置城市和时间
city = '上海'
year = 2021
quarter = 2
# 爬取数据
url = f'http://tianqi.2345.com/t/wea_history/js/{city}/{year}/{quarter}.js'
response = requests.get(url)
text = response.content.decode('gbk')
# 正则表达式匹配
pattern = re.compile(r'(\d{4}-\d{2}-\d{2})\|(\d{1,2})\|(\d{1,2})\|(\d{1,3})\|(\d{1,3})\|(\D+)\n')
result = pattern.findall(text)
# 数据整理
data = pd.DataFrame(result, columns=['日期', '最高温度', '最低温度', '空气质量指数', '风力等级', '天气'])
data[['最高温度', '最低温度', '空气质量指数', '风力等级']] = data[['最高温度', '最低温度', '空气质量指数', '风力等级']].astype(int)
data['日期'] = pd.to_datetime(data['日期'])
# 可视化分析
# 统计天气情况
weather_count = data['天气'].value_counts()
weather_count = weather_count[:10]
# 分词统计
seg_list = jieba.cut(' '.join(data['天气'].tolist()))
words = {}
for word in seg_list:
if len(word) < 2:
continue
if word in words:
words[word] += 1
else:
words[word] = 1
# 绘制柱状图和词云图
plt.figure(figsize=(10, 5))
plt.bar(weather_count.index, weather_count.values)
plt.title(f'{city}{year}年第{quarter}季度天气情况')
plt.xlabel('天气')
plt.ylabel('次数')
plt.savefig('weather_bar.png')
wordcloud = pd.DataFrame(list(words.items()), columns=['word', 'count'])
mask_image = imageio.imread('cloud_mask.png')
plt.figure(figsize=(10, 5))
plt.imshow(mask_image, cmap=plt.cm.gray, interpolation='bilinear')
plt.axis('off')
plt.savefig('mask.png')
wordcloud.plot(kind='scatter', x='count', y='count', alpha=0.5, s=300, cmap='Reds', figsize=(10, 5))
for i in range(len(wordcloud)):
plt.text(wordcloud.iloc[i]['count'], wordcloud.iloc[i]['count'], wordcloud.iloc[i]['word'], ha='center', va='center', fontproperties='SimHei')
plt.axis('off')
plt.savefig('weather_wordcloud.png')
```
注意:这里的 cloud_mask.png 是一个用于生成词云图的模板图片,可以替换成自己喜欢的图片。
阅读全文
相关推荐
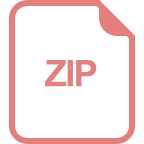
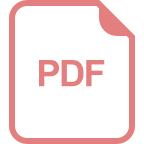
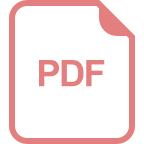
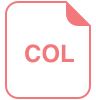
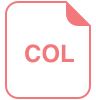
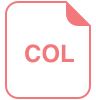
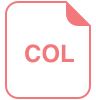
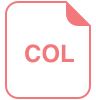



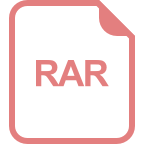