请帮我写一段基于情感词典的文本情感分析代码,用python语言
时间: 2023-02-23 20:29:38 浏览: 149
好的,下面是一个基于情感词典的文本情感分析的简单代码:
```
# 定义情感词典,key为词语,value为分值
sentiment_dict = {
"开心": 1,
"高兴": 1,
"快乐": 1,
"郁闷": -1,
"悲伤": -1,
"不开心": -1
}
# 定义文本
text = "今天天气不错,心情开心"
# 对文本进行分析,统计情感分值
sentiment_score = 0
words = text.split()
for word in words:
if word in sentiment_dict:
sentiment_score += sentiment_dict[word]
# 判断文本的情感极性
if sentiment_score > 0:
print("正面情感")
elif sentiment_score == 0:
print("中性情感")
else:
print("负面情感")
```
这段代码实现了基本的文本情感分析功能,你可以根据实际需要进行修改和扩展。
相关问题
请帮我写一段基于esg情感词典的文本情感分析代码,用python语言
### 回答1:
当然,我很愿意帮助您写一段基于ESG情感词典的文本情感分析代码。
以下是基于Python语言的代码示例:
```
import nltk
from nltk.sentiment import SentimentIntensityAnalyzer
nltk.download('vader_lexicon')
def analyze_sentiment(text):
sid = SentimentIntensityAnalyzer()
sentiment = sid.polarity_scores(text)
if sentiment['compound'] >= 0.05:
return 'positive'
elif sentiment['compound'] <= -0.05:
return 'negative'
else:
return 'neutral'
text = "这是一段很好的文本,我感到非常开心。"
sentiment = analyze_sentiment(text)
print("情感分析结果:", sentiment)
```
该代码使用了 `nltk` 库中的 `SentimentIntensityAnalyzer` 函数,该函数基于VADER情感词典对文本进行情感分析。代码中的 `analyze_sentiment` 函数接收文本作为输入,返回该文本的情感(positive,negative或neutral)。
### 回答2:
当进行文本情感分析时,可以使用esg情感词典作为参考。下面是一个基于Python语言的简单文本情感分析代码示例:
```python
import pandas as pd
# 读取情感词典
esg_dict = pd.read_csv('esg_dict.csv')
# 定义情感分析函数
def sentiment_analysis(text):
# 初始化情感得分
sentiment_score = 0
# 将文本拆分为单词
words = text.strip().split()
# 遍历每个单词
for word in words:
# 查找情感词典中的单词
result = esg_dict[esg_dict['Word'] == word]
if not result.empty:
# 如果单词在情感词典中存在,获取情感得分
sentiment_score += result['Sentiment'].values[0]
# 判断情感得分并返回结果
if sentiment_score > 0:
return '积极'
elif sentiment_score < 0:
return '消极'
else:
return '中性'
# 测试文本情感分析函数
text = '这部电影很棒!'
result = sentiment_analysis(text)
print(result)
```
上述代码首先读取了一个包含情感词和对应情感得分的esg_dict.csv文件,并定义了一个情感分析函数sentiment_analysis。在函数中,我们将输入的文本拆分为单词,然后逐个查找情感词典中的单词并累加情感得分。最后根据得分判断情感为积极、消极或中性,并返回结果。
在示例中,我们使用了一个测试文本“这部电影很棒!”进行情感分析,得到的结果是积极。你可以将代码中的esg_dict.csv替换为你的情感词典文件,并根据需要进行修改和拓展以适应你的实际情况。
### 回答3:
当谈到基于ESG情感词典的文本情感分析代码时,我们可以使用Python语言编写一个简单的示例。
首先,我们需要准备好ESG情感词典。ESG情感词典是一个包含了一系列词汇及其情感极性(例如积极,消极或中性)的文件。
接下来,我们需要使用Python的文本处理库来进行情感分析。在这个示例中,我们将使用NLTK(Natural Language Toolkit)库作为我们的文本处理工具。
首先,导入必要的库:
```
import nltk
```
然后,加载ESG情感词典。在这个示例中,我们将假设情感词典是一个以行为单位的文本文件,每行包含一个词汇及其情感极性(以逗号或制表符分隔)。
```
esg_dict = {}
with open('esg_dict.txt', 'r') as f:
for line in f:
word, polarity = line.strip().split(',')
esg_dict[word] = polarity
```
接下来,我们可以定义一个函数来进行情感分析。在这个函数中,我们将接受一段文本作为输入,并根据ESG情感词典来计算总体情感得分。
```
def sentiment_analysis(text):
tokens = nltk.word_tokenize(text) # 将文本分词
score = 0 # 总体情感得分
for token in tokens:
if token in esg_dict:
polarity = esg_dict[token] # 获取词汇的情感极性
score += int(polarity) # 将情感极性加到总体得分中
return score
```
最后,我们可以调用这个函数并传入我们感兴趣的一段文本来执行情感分析。示例如下:
```
text = "这是一个非常积极的消息,将有利于公司的可持续发展。"
result = sentiment_analysis(text)
print("情感得分:", result)
```
这个例子是一个简化的情感分析代码,可以根据实际需求进行扩展和改进。
python基于情感词典进行情感分析的代码
以下是一段基于情感词典的Python情感分析代码。使用情感词典可以将文本中的每个单词与情感极性相关联,然后计算每个单词的情感极性得分并对得分进行加权平均,从而得出整个文本的情感极性。
首先,需要下载并导入情感词典。这里使用的是NTUSD情感词典,可以从以下链接下载并解压缩:
http://www.nlg.csie.ntu.edu.tw/nlpresource/NTUSD-Fin/NTUSD-Fin.zip
然后读取情感词典文件并将每个单词与情感极性相关联:
```python
def read_sentiment_dict(filename):
sentiment_dict = {}
with open(filename, 'r', encoding='utf-8') as f:
for line in f:
tokens = line.strip().split('\t')
word = tokens[0]
sentiment = int(tokens[1])
sentiment_dict[word] = sentiment
return sentiment_dict
filename = 'NTUSD-Fin/NTUSD_positive_negative_simplified.txt'
sentiment_dict = read_sentiment_dict(filename)
```
接下来,可以定义一个函数来计算文本的情感极性得分。该函数将文本分成单词,并对每个单词计算情感极性得分,然后将得分进行加权平均:
```python
def calculate_sentiment(text, sentiment_dict):
words = text.split()
sentiment_scores = []
for word in words:
if word in sentiment_dict:
sentiment_scores.append(sentiment_dict[word])
if len(sentiment_scores) > 0:
sentiment_score = sum(sentiment_scores) / len(sentiment_scores)
else:
sentiment_score = 0
return sentiment_score
```
最后,可以使用该函数来计算文本的情感极性得分,并根据得分来判断情感:
```python
text = "I love this product, it's amazing!"
sentiment_score = calculate_sentiment(text, sentiment_dict)
if sentiment_score > 0:
sentiment = 'Positive'
elif sentiment_score == 0:
sentiment = 'Neutral'
else:
sentiment = 'Negative'
print(sentiment)
```
完整代码:
```python
def read_sentiment_dict(filename):
sentiment_dict = {}
with open(filename, 'r', encoding='utf-8') as f:
for line in f:
tokens = line.strip().split('\t')
word = tokens[0]
sentiment = int(tokens[1])
sentiment_dict[word] = sentiment
return sentiment_dict
def calculate_sentiment(text, sentiment_dict):
words = text.split()
sentiment_scores = []
for word in words:
if word in sentiment_dict:
sentiment_scores.append(sentiment_dict[word])
if len(sentiment_scores) > 0:
sentiment_score = sum(sentiment_scores) / len(sentiment_scores)
else:
sentiment_score = 0
return sentiment_score
filename = 'NTUSD-Fin/NTUSD_positive_negative_simplified.txt'
sentiment_dict = read_sentiment_dict(filename)
text = "I love this product, it's amazing!"
sentiment_score = calculate_sentiment(text, sentiment_dict)
if sentiment_score > 0:
sentiment = 'Positive'
elif sentiment_score == 0:
sentiment = 'Neutral'
else:
sentiment = 'Negative'
print(sentiment)
```
阅读全文
相关推荐
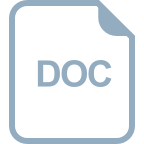
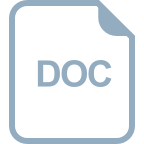
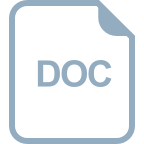
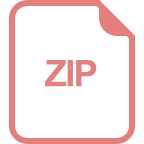
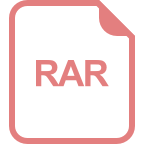
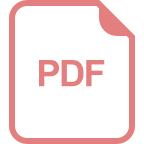
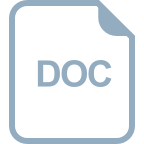
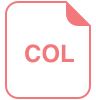








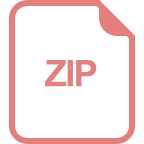