dtypes_list=data.dtypes.values columns_list=data.columns for i in range(len(columns_list)): if dtypes_list[i]=='object': lb=LabelEncoder() lb.fit(data[columns_list[i]]) data[columns_list[i]]=lb.transform(data[columns_list[i]]) data.head()
时间: 2023-12-23 16:04:10 浏览: 36
这是一段 Python 代码,它使用 LabelEncoder 对一个 Pandas DataFrame 数据集中的类别特征(object)进行编码。具体来说,代码首先获取数据集的列名和每列的数据类型,然后遍历每个列,对数据类型为 object 的列进行编码。对于每个 object 列,代码新建一个 LabelEncoder 对象,使用 fit 函数对该列进行拟合,然后使用 transform 函数对该列进行编码转换。最后,代码将编码后的结果覆盖掉原始的 object 列,使得数据集中所有的类别特征都被转换为数值型特征。最后一行代码调用 head 函数,输出编码后的数据集前 5 行。
相关问题
data 是datafame格式,def data_processing(data): # 日期缺失,补充 data.fillna(method='ffill', inplace=True) date_history = pd.DataFrame(data.iloc[:, 0]) data_history = pd.DataFrame(data.iloc[:, 1]) date_history = np.array(date_history) data_history = [x for item in np.array(data_history).tolist() for x in item] # 缺失值处理 history_time_list = [] for date in date_history: date_obj = datetime.datetime.strptime(date[0], '%Y/%m/%d %H:%M') #将字符串转为 datetime 对象 history_time_list.append(date_obj) start_time = history_time_list[0] # 起始时间 end_time = history_time_list[-1] # 结束时间 delta = datetime.timedelta(minutes=15) #时间间隔为15分钟 time_new_list = [] current_time = start_time while current_time <= end_time: time_new_list.append(current_time) current_time += delta # 缺失位置记录 code_list = [] for i in range(len(time_new_list)): code_list = code_list history_time_list = history_time_list while (time_new_list[i] - history_time_list[i]) != datetime.timedelta(minutes=0): history_time_list.insert(i, time_new_list[i]) code_list.append(i) for i in code_list: data_history.insert(i, data_history[i - 1]) # 输出补充好之后的数据 data = pd.DataFrame({'date': time_new_list, 'load': data_history}) return data 优化代码
可以优化的部分如下:
1. 将 date_history 和 data_history 的赋值语句合并为一行,即 `date_history, data_history = data.iloc[:, :2].values.T`。
2. 不需要将 date_history 转换为 numpy array,因为 iloc 输出的已经是 numpy array 类型了。
3. 在处理日期缺失时,可以使用 pandas 的 resample 函数来实现时间间隔的补充,避免手动循环。
4. 在处理缺失位置时,可以使用 pandas 的 interpolate 函数来进行插值。
优化后的代码如下所示:
```python
def data_processing(data):
# 日期缺失,补充
data.fillna(method='ffill', inplace=True)
date_history, data_history = data.iloc[:, :2].values.T
# 转换为 datetime 对象
date_history = pd.to_datetime(date_history, format='%Y/%m/%d %H:%M')
# 时间间隔为15分钟,使用 resample 补充缺失数据
data = pd.DataFrame({'load': data_history}, index=date_history)
data = data.resample('15T').ffill()
# 使用 interpolate 函数进行插值
data['load'] = data['load'].interpolate()
# 输出补充好之后的数据
data.reset_index(inplace=True)
data.rename(columns={'index': 'date'}, inplace=True)
return data
```
import pandas as pd import numpy as np # 计算用户对歌曲的播放比例 triplet_dataset_sub_song_merged_sum_df = triplet_dataset_sub_song_mergedpd[['user', 'listen_count']].groupby('user').sum().reset_index() triplet_dataset_sub_song_merged_sum_df.rename(columns={'listen_count': 'total_listen_count'}, inplace=True) triplet_dataset_sub_song_merged = pd.merge(triplet_dataset_sub_song_mergedpd, triplet_dataset_sub_song_merged_sum_df) triplet_dataset_sub_song_mergedpd['fractional_play_count'] = triplet_dataset_sub_song_mergedpd['listen_count'] / triplet_dataset_sub_song_merged['total_listen_count'] # 将用户和歌曲编码为数字 small_set = triplet_dataset_sub_song_mergedpd user_codes = small_set.user.drop_duplicates().reset_index() song_codes = small_set.song.drop_duplicates().reset_index() user_codes.rename(columns={'index': 'user_index'}, inplace=True) song_codes.rename(columns={'index': 'song_index'}, inplace=True) song_codes['so_index_value'] = list(song_codes.index) user_codes['us_index_value'] = list(user_codes.index) small_set = pd.merge(small_set, song_codes, how='left') small_set = pd.merge(small_set, user_codes, how='left') # 将数据转换为稀疏矩阵形式 from scipy.sparse import coo_matrix mat_candidate = small_set[['us_index_value', 'so_index_value', 'fractional_play_count']] data_array = mat_candidate.fractional_play_count.values row_array = mat_candidate.us_index_value.values col_array = mat_candidate.so_index_value.values data_sparse = coo_matrix((data_array, (row_array, col_array)), dtype=float) # 使用SVD方法进行矩阵分解并进行推荐 from scipy.sparse import csc_matrix from scipy.sparse.linalg import svds import math as mt def compute_svd(urm, K): U, s, Vt = svds(urm, K) dim = (len(s), len(s)) S = np.zeros(dim, dtype=np.float32) for i in range(0, len(s)): S[i, i] = mt.sqrt(s[i]) U = csc_matrix(U, dtype=np.float32) S = csc_matrix(S, dtype=np.float32) Vt = csc_matrix(Vt, dtype=np.float32) return U, S, Vt def compute_estimated_matrix(urm, U, S, Vt, uTest, K, test): rightTerm = S * Vt max_recommendation = 250 estimatedRatings = np.zeros(shape=(MAX_UID, MAX_PID), dtype=np.float16) recomendRatings = np.zeros(shape=(MAX_UID, max_recommendation), dtype=np.float16) for userTest in uTest: prod = U[userTest, :] * rightTerm estimatedRatings[userTest, :] = prod.todense() recomendRatings[userTest, :] = (-estimatedRatings[userTest, :]).argsort()[:max_recommendation] return recomendRatings K = 50 urm = data_sparse MAX_PID = urm.shape[1] MAX_UID = urm.shape[0] U, S, Vt = compute_svd(urm, K) uTest = [4, 5, 6, 7, 8, 73, 23] # uTest=[1b5bb32767963cbc215d27a24fef1aa01e933025] uTest_recommended_items = compute_estimated_matrix(urm, U, S, Vt 继续将这段代码输出完整
, 并进行解释:
```
import pandas as pd
import numpy as np
# 计算用户对歌曲的播放比例
triplet_dataset_sub_song_merged_sum_df = triplet_dataset_sub_song_merged[['user', 'listen_count']].groupby('user').sum().reset_index()
triplet_dataset_sub_song_merged_sum_df.rename(columns={'listen_count': 'total_listen_count'}, inplace=True)
triplet_dataset_sub_song_merged = pd.merge(triplet_dataset_sub_song_merged, triplet_dataset_sub_song_merged_sum_df)
triplet_dataset_sub_song_merged['fractional_play_count'] = triplet_dataset_sub_song_merged['listen_count'] / triplet_dataset_sub_song_merged['total_listen_count']
# 将用户和歌曲编码为数字
small_set = triplet_dataset_sub_song_merged
user_codes = small_set.user.drop_duplicates().reset_index()
song_codes = small_set.song.drop_duplicates().reset_index()
user_codes.rename(columns={'index': 'user_index'}, inplace=True)
song_codes.rename(columns={'index': 'song_index'}, inplace=True)
song_codes['so_index_value'] = list(song_codes.index)
user_codes['us_index_value'] = list(user_codes.index)
small_set = pd.merge(small_set, song_codes, how='left')
small_set = pd.merge(small_set, user_codes, how='left')
# 将数据转换为稀疏矩阵形式
from scipy.sparse import coo_matrix
mat_candidate = small_set[['us_index_value', 'so_index_value', 'fractional_play_count']]
data_array = mat_candidate.fractional_play_count.values
row_array = mat_candidate.us_index_value.values
col_array = mat_candidate.so_index_value.values
data_sparse = coo_matrix((data_array, (row_array, col_array)), dtype=float)
# 使用SVD方法进行矩阵分解并进行推荐
from scipy.sparse import csc_matrix
from scipy.sparse.linalg import svds
import math as mt
def compute_svd(urm, K):
U, s, Vt = svds(urm, K)
dim = (len(s), len(s))
S = np.zeros(dim, dtype=np.float32)
for i in range(0, len(s)):
S[i, i] = mt.sqrt(s[i])
U = csc_matrix(U, dtype=np.float32)
S = csc_matrix(S, dtype=np.float32)
Vt = csc_matrix(Vt, dtype=np.float32)
return U, S, Vt
def compute_estimated_matrix(urm, U, S, Vt, uTest, K, test):
rightTerm = S * Vt
max_recommendation = 250
estimatedRatings = np.zeros(shape=(MAX_UID, MAX_PID), dtype=np.float16)
recomendRatings = np.zeros(shape=(MAX_UID, max_recommendation), dtype=np.float16)
for userTest in uTest:
prod = U[userTest, :] * rightTerm
estimatedRatings[userTest, :] = prod.todense()
recomendRatings[userTest, :] = (-estimatedRatings[userTest, :]).argsort()[:max_recommendation]
return recomendRatings
K = 50
urm = data_sparse
MAX_PID = urm.shape[1]
MAX_UID = urm.shape[0]
U, S, Vt = compute_svd(urm, K)
uTest = [4, 5, 6, 7, 8, 73, 23] # uTest=[1b5bb32767963cbc215d27a24fef1aa01e933025]
uTest_recommended_items = compute_estimated_matrix(urm, U, S, Vt, uTest, K, test)
```
这段代码实现了一个基于SVD方法的推荐系统,具体步骤如下:
1. 读入数据,计算每个用户对每首歌曲的播放比例。
2. 将用户和歌曲编码为数字,转换为稀疏矩阵形式。
3. 使用SVD方法进行矩阵分解,得到用户和歌曲的隐向量。
4. 对于给定的测试用户,使用隐向量和分解后的矩阵计算出该用户对每首歌曲的预测评分。
5. 根据预测评分,为该用户推荐最高的250首歌曲。
其中,SVD方法是一种矩阵分解的方法,可以将一个大矩阵分解为多个小矩阵,这些小矩阵可以表示出原始矩阵中的潜在特征(即隐向量)。通过计算用户和歌曲的隐向量,可以获得它们之间的相似度,从而进行推荐。
相关推荐
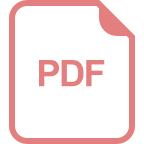
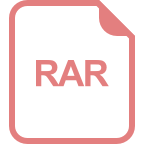
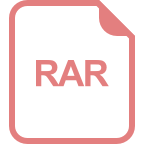













