分析以下代码: public static Dict getIPAndCity() { Dict dict = Dict.create(); try { Document document = Jsoup.connect("https://ip.900cha.com/").get(); // /html/body/div/div/div/div[1]/div[1]/h3 String ip = document.selectXpath("/html/body/div/div/div/div[1]/div[1]/h3").get(0).text().trim(); // 通过 Xpath 直接获取了网页里面的内容 dict.set("ip", ip); String city = ""; if (IP_MAP.get(ip) != null) { city = IP_MAP.get(ip); dict.set("city", city); return dict; } String url = "https://api.map.baidu.com/location/ip?ip=" + ip + "&ak=bmvg8yeOopwOB4aHl5uvx52rgIa3VrPO"; String res = HttpUtil.createRequest(Method.GET, url).execute().body(); String json = UnicodeUtil.toString(res); JSON jsonObject = JSONUtil.parseObj(json); city = jsonObject.getByPath("content.address", String.class); dict.set("city", city); } catch (Exception e) { log.error("获取IP失败", e); } return dict; } }
时间: 2023-12-06 07:43:40 浏览: 148
这段代码的作用是获取当前机器的公网IP地址以及所在城市信息,并将结果封装到一个Dict对象中返回。
具体实现过程如下:
1. 创建一个空的Dict对象。
2. 通过Jsoup框架向一个网站(https://ip.900cha.com/)发送请求,并获取返回的HTML文档。
3. 使用xpath语法获取HTML文档中指定元素的文本内容,即当前机器的公网IP地址,并将其存入Dict对象中。
4. 判断当前IP地址是否已经被存储在一个名为IP_MAP的Map对象中,如果已经存在,则直接从Map对象中获取对应的城市信息并存入Dict对象中。
5. 如果当前IP地址不存在于IP_MAP中,则向百度地图API发送请求,通过IP地址获取所在城市的信息,并将城市信息存入Dict对象中。
6. 如果在任何步骤中出现了异常,则记录日志,并返回一个空的Dict对象。
7. 最后,将存储有IP地址和城市信息的Dict对象返回给调用者。
相关问题
def create_patrol_plan(cls, vals) -> dict: model_env = request.env[cls.models].sudo() data = request.jsonrequest or {} # 校验 for i in data: patrol_date = i.get('patrol_date') obj = model_env.search([('patrol_date', '=', patrol_date)]) if obj: # 已存在则修改 vals.update({'id': obj.id}) cls.home_public_write_dict(cls.models, vals) continue # 新增 create_data = cls.home_public_create_dict(model=cls.models, data=i) model_env.create(create_data) return 1该功能有什么缺陷
根据您提供的代码片段,我发现以下潜在问题:
1. 数据校验只检查了日期是否已存在,但未检查数据的完整性和一致性,例如是否包含必需的字段、字段是否符合规范和逻辑等。
2. 函数返回的是数字1,没有明确表示是否操作成功,也没有返回更详细的错误信息,难以进行错误处理和调试。
3. 函数中使用了`sudo()`和`jsonrequest`,这可能会带来安全风险和性能问题,因为`sudo()`会绕过访问控制和审计日志,而`jsonrequest`需要将请求体解析为JSON对象,可能存在解析失败和恶意数据攻击的风险。
4. 函数中的`cls.home_public_write_dict`和`cls.home_public_create_dict`方法未给出其实现细节和输入输出,难以判断其是否符合要求和最佳实践。
为了提高代码的可维护性、可扩展性和安全性,建议对函数进行重构和优化,例如:
1. 引入数据模型和验证器,将校验逻辑和数据操作分离,确保数据的完整性和一致性。
2. 使用标准的HTTP响应代码和消息,例如200表示成功,400表示请求无效,401表示未授权,403表示禁止访问,500表示服务器内部错误等,方便客户端处理错误和跟踪问题。
3. 避免直接操作数据库,使用ORM框架或者数据访问层封装,以提高代码的可读性和可测试性。
4. 使用适当的安全措施,例如防止SQL注入、XSS攻击、CSRF攻击、数据泄露等,以保护应用程序和用户的安全。
def Stop_words(): stopword = [] data = [] f = open('C:/Users/Administrator/Desktop/data/stopword.txt',encoding='utf8') for line in f.readlines(): data.append(line) for i in data: output = str(i).replace('\n','')#replace用法和sub函数很接近 stopword.append(output) return stopword # 采用jieba进行词性标注,对当前文档过滤词性和停用词 def Filter_word(text): filter_word = [] stopword = Stop_words() text = jieba.posseg.cut(text) for word, flag in text: if flag.startswith('n') is False:#用于检测字符串是否以指定的子字符串开始 continue if not word in stopword and len(word) > 1: filter_word.append(word) return filter_word # 对文档集过滤词性和停用词 def Filter_words(data_path =r'C:/Users/Administrator/Desktop/data//corpus.txt'): document = [] for line in open(data_path, 'r',encoding= 'utf8') : segment = jieba.posseg.cut(line.strip()) filter_words = [] stopword = Stop_words() for word, flag in segment: if flag.startswith('n') is False: continue if not word in stopword and len(word) > 1: filter_words.append(word) document.append(filter_words) return document def tf_idf(): tf_dict = {} idf_dict = {} filter_word = Filter_word(text) for word in filter_word: if word not in tf_dict: tf_dict[word] = 1 else: tf_dict[word] += 1 for word in tf_dict: tf_dict[word] = tf_dict[word] / len(text) document = Filter_words() doc_total = len(document) for doc in document: for word in set(doc): if word not in idf_dict: idf_dict[word] = 1 else: idf_dict[word] += 1 for word in idf_dict: idf_dict[word] = math.log(doc_total / (idf_dict[word] + 1)) tf_idf_dict = {} for word in filter_word: if word not in idf_dict: idf_dict[word] = 0 tf_idf_dict[word] = tf_dict[word] * idf_dict[word] return tf_idf_dict tf_idf_dict = tf_idf() keyword = 6 print('TF-IDF模型结果:') for key, value in sorted(tf_idf_dict.items(), key=operator.itemgetter(1),reverse=True)[:keyword]: print(key, end=' ') print('\n')
这段代码实现了一个 TF-IDF 模型,用于计算文本中关键词的权重。其中,Stop_words 函数用于读取停用词表,Filter_word 函数用于对单个文档进行过滤,Filter_words 函数用于对整个文档集进行过滤。tf_idf 函数用于计算 TF-IDF 值,最后输出了权重最高的前 keyword 个关键词。
阅读全文
相关推荐
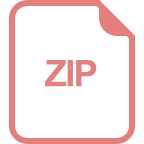
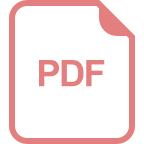















