BasicConv2d()
时间: 2023-12-15 17:05:10 浏览: 44
BasicConv2d() 是一个基本的卷积神经网络层,用于图像分类、目标检测等计算机视觉任务。它通常包含两个步骤:卷积和激活函数。卷积用于提取图像特征,激活函数用于对卷积后的特征进行非线性变换。
BasicConv2d() 的输入是一个四维张量,即形状为 [batch_size, height, width, channels] 的图像。它的输出也是一个四维张量,即形状为 [batch_size, new_height, new_width, num_filters] 的特征图。其中,new_height 和 new_width 是卷积后的特征图的高度和宽度,num_filters 是卷积核的数量,也是特征图的通道数。
在 BasicConv2d() 中,通常会设置卷积核的大小、步长、填充方式等参数,以及激活函数的类型。常用的激活函数有 relu、sigmoid、tanh 等。
相关问题
BasicConv2d
BasicConv2d is a type of convolutional layer used in deep learning models for image classification tasks. It is a simple, yet effective, implementation of a convolutional layer that performs a 2D convolution operation on the input image. The BasicConv2d layer typically consists of a set of learnable filters or kernels, which are convolved with the input image to produce a set of output feature maps. These feature maps are then passed through a non-linear activation function to introduce non-linearity into the model. The BasicConv2d layer is often used as the first layer in a deep learning model, as it can help to extract low-level features from the input image. Overall, BasicConv2d is a widely used building block in many state-of-the-art deep learning models for image classification tasks.
怎么引入BasicConv2d
在 PyTorch 中,可以使用 `nn.Module` 类来定义自己的神经网络模型。下面是一个使用 `BasicConv2d` 的例子:
```python
import torch.nn as nn
class MyModel(nn.Module):
def __init__(self):
super(MyModel, self).__init__()
# 定义 BasicConv2d 层
self.conv = nn.Sequential(
nn.Conv2d(in_channels=3, out_channels=64, kernel_size=3, padding=1),
nn.BatchNorm2d(64),
nn.ReLU(inplace=True),
BasicConv2d(64, 128, kernel_size=3, padding=1),
nn.MaxPool2d(kernel_size=2, stride=2),
BasicConv2d(128, 256, kernel_size=3, padding=1),
nn.MaxPool2d(kernel_size=2, stride=2),
BasicConv2d(256, 512, kernel_size=3, padding=1),
nn.MaxPool2d(kernel_size=2, stride=2),
BasicConv2d(512, 512, kernel_size=3, padding=1),
nn.MaxPool2d(kernel_size=2, stride=2)
)
def forward(self, x):
x = self.conv(x)
return x
```
在这个例子中,我们先定义了一个基本的卷积层,然后在 `self.conv` 中使用了多个 `BasicConv2d` 层,将这些层串联在一起。在 `forward` 方法中,我们将输入数据 `x` 通过 `self.conv` 进行前向传播并返回输出。
阅读全文
相关推荐
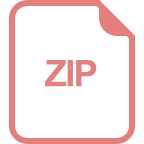
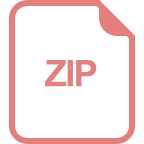







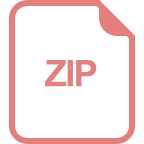
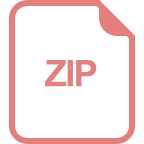
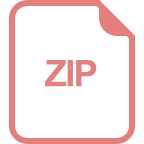
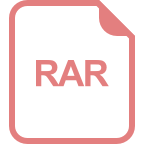
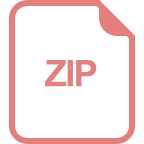