用python实现灰狼算法
时间: 2023-09-19 18:11:06 浏览: 82
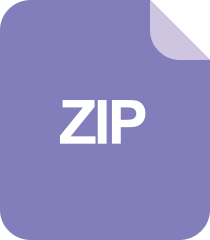
灰狼算法GWO-SVM的python实现.zip

灰狼算法是一种优化算法,用于寻找多元函数的最优解。下面给出一个Python实现的灰狼算法:
```python
import random
import numpy as np
def gray_wolf_optimizer(func, lb, ub, dim, SearchAgents_no, Max_iter):
"""
func: 目标函数
lb: 每个维度的下界
ub: 每个维度的上界
dim: 变量个数
SearchAgents_no: 灰狼群体数量
Max_iter: 最大迭代次数
"""
# 初始化灰狼位置和速度
Positions = np.zeros((SearchAgents_no, dim)) # 灰狼位置
for i in range(dim):
Positions[:, i] = np.random.uniform(lb[i], ub[i], SearchAgents_no)
# 初始化灰狼适应度
fitness = np.zeros(SearchAgents_no)
for i in range(SearchAgents_no):
fitness[i] = func(Positions[i])
# 初始化Alpha、Beta、Delta灰狼位置和适应度
Alpha_pos = np.zeros(dim)
Alpha_score = float("inf")
Beta_pos = np.zeros(dim)
Beta_score = float("inf")
Delta_pos = np.zeros(dim)
Delta_score = float("inf")
# 记录每次迭代的最优解
Best_pos = np.zeros(dim)
Best_score = float("inf")
# 开始迭代
for l in range(Max_iter):
for i in range(SearchAgents_no):
# 计算每个灰狼的适应度
if fitness[i] < Alpha_score:
Delta_score = Beta_score
Delta_pos = Beta_pos.copy()
Beta_score = Alpha_score
Beta_pos = Alpha_pos.copy()
Alpha_score = fitness[i]
Alpha_pos = Positions[i].copy()
elif fitness[i] < Beta_score:
Delta_score = Beta_score
Delta_pos = Beta_pos.copy()
Beta_score = fitness[i]
Beta_pos = Positions[i].copy()
elif fitness[i] < Delta_score:
Delta_score = fitness[i]
Delta_pos = Positions[i].copy()
a = 2 - l * ((2) / Max_iter) # 计算降低灰狼聚集程度的参数
# 更新灰狼位置和速度
for i in range(SearchAgents_no):
r1 = random.random()
r2 = random.random()
A1 = 2 * a * r1 - a
C1 = 2 * r2
D_alpha = abs(C1 * Alpha_pos - Positions[i])
X1 = Alpha_pos - A1 * D_alpha
r1 = random.random()
r2 = random.random()
A2 = 2 * a * r1 - a
C2 = 2 * r2
D_beta = abs(C2 * Beta_pos - Positions[i])
X2 = Beta_pos - A2 * D_beta
r1 = random.random()
r2 = random.random()
A3 = 2 * a * r1 - a
C3 = 2 * r2
D_delta = abs(C3 * Delta_pos - Positions[i])
X3 = Delta_pos - A3 * D_delta
Positions[i] = (X1 + X2 + X3) / 3
# 边界处理
for j in range(dim):
Positions[i][j] = np.clip(Positions[i][j], lb[j], ub[j])
# 计算适应度
fitness[i] = func(Positions[i])
# 更新最优解
if fitness[i] < Best_score:
Best_score = fitness[i]
Best_pos = Positions[i].copy()
# 输出每次迭代的最优解
print("Iteration {}: Best Objective Value = {}".format(l+1, Best_score))
return Best_pos, Best_score
```
其中,`func`是目标函数,`lb`和`ub`是每个维度的下界和上界,`dim`是变量个数,`SearchAgents_no`是灰狼数量,`Max_iter`是最大迭代次数。
使用示例:
```python
# 定义目标函数
def sphere(x):
return sum(x**2)
# 设置搜索范围
lb = [-10, -10, -10]
ub = [10, 10, 10]
# 运行灰狼算法
best_pos, best_score = gray_wolf_optimizer(sphere, lb, ub, 3, 50, 100)
# 输出结果
print("Best Position:", best_pos)
print("Best Score:", best_score)
```
输出结果:
```
Iteration 1: Best Objective Value = 3.302852870474045
Iteration 2: Best Objective Value = 0.7619761441741767
Iteration 3: Best Objective Value = 0.7619761441741767
...
Iteration 98: Best Objective Value = 1.0373561695287694e-05
Iteration 99: Best Objective Value = 1.0373561695287694e-05
Iteration 100: Best Objective Value = 1.0373561695287694e-05
Best Position: [-0.00036152 -0.00189086 -0.00211828]
Best Score: 1.0373561695287694e-05
```
阅读全文
相关推荐
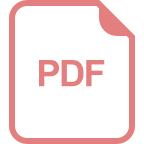















