tensorflow图片输入格式怎么转换为(None, 56, 56)格式
时间: 2024-04-08 19:32:54 浏览: 26
要将图片输入格式转换为 `(None, 56, 56)`,你需要使用 TensorFlow 的图像处理函数来调整图片的大小。
以下是一个示例代码,演示如何将图片调整为 `(None, 56, 56)` 的格式:
```python
import tensorflow as tf
# 读取图片文件
image_path = 'path/to/your/image.jpg'
image = tf.io.read_file(image_path)
# 解码图片
image = tf.image.decode_image(image)
# 将图片调整为指定大小
target_size = (56, 56)
image = tf.image.resize(image, target_size)
# 可选:将像素值缩放到 [0, 1] 范围
image = tf.cast(image, tf.float32) / 255.0
# 打印图像的形状和数据类型
print('Image shape:', image.shape)
print('Image dtype:', image.dtype)
```
请将 `image_path` 替换为你要读取的图片文件的路径。此代码将读取指定路径的图片文件,并对其进行解码、调整大小、像素值缩放等处理,最终得到形状为 `(56, 56)` 的图片。
注意:`(None, 56, 56)` 的格式中的 `None` 表示批次维度,在实际使用中可能需要根据具体的任务和需求进行适当的调整。
相关问题
空洞自编码器 tensorflow
空洞自编码器(Hollowing Autoencoder)是一种基于深度学习的无监督学习方法,它可以用于图像处理、信号处理等领域。它的主要原理是通过将输入图像中的区域挖空,让编码器学习仅仅包含部分信息的图像,然后再通过解码器将挖空的区域填充回来,从而实现图像的重建。
在 TensorFlow 中,实现空洞自编码器可以分为以下几个步骤:
1. 定义编码器和解码器模型
编码器模型的输入是原始图像,输出是挖空后的图像。解码器模型的输入是挖空后的图像,输出是重建后的图像。可以使用卷积神经网络来实现编码器和解码器模型。
2. 定义损失函数
损失函数包括两部分:重建误差和空洞损失。重建误差计算原始图像和重建后的图像之间的差异,空洞损失计算挖空的区域和原始图像之间的差异。可以使用均方误差(MSE)作为重建误差的度量,使用交叉熵(Cross-entropy)作为空洞损失的度量。
3. 训练模型
使用优化器来最小化损失函数,从而训练编码器和解码器模型。
4. 测试模型
将测试图像输入编码器模型,得到挖空后的图像。然后将挖空后的图像输入解码器模型,得到重建后的图像。
下面是一个简单的 TensorFlow 空洞自编码器代码示例:
```python
import tensorflow as tf
# 定义编码器模型
def encoder(input_img):
conv1 = tf.layers.conv2d(input_img, filters=64, kernel_size=3, activation=tf.nn.relu, padding='same')
pool1 = tf.layers.max_pooling2d(conv1, pool_size=2, strides=2, padding='same')
conv2 = tf.layers.conv2d(pool1, filters=32, kernel_size=3, activation=tf.nn.relu, padding='same')
pool2 = tf.layers.max_pooling2d(conv2, pool_size=2, strides=2, padding='same')
conv3 = tf.layers.conv2d(pool2, filters=16, kernel_size=3, activation=tf.nn.relu, padding='same')
return conv3
# 定义解码器模型
def decoder(encoded_img):
conv1 = tf.layers.conv2d(encoded_img, filters=16, kernel_size=3, activation=tf.nn.relu, padding='same')
up1 = tf.image.resize_images(conv1, size=(14, 14))
conv2 = tf.layers.conv2d(up1, filters=32, kernel_size=3, activation=tf.nn.relu, padding='same')
up2 = tf.image.resize_images(conv2, size=(28, 28))
conv3 = tf.layers.conv2d(up2, filters=64, kernel_size=3, activation=tf.nn.relu, padding='same')
up3 = tf.image.resize_images(conv3, size=(56, 56))
conv4 = tf.layers.conv2d(up3, filters=1, kernel_size=3, activation=None, padding='same')
return conv4
# 定义空洞自编码器模型
def autoencoder(input_img, mask):
# 将输入图像挖空
masked_img = tf.multiply(input_img, mask)
# 编码器模型
encoded_img = encoder(masked_img)
# 解码器模型
decoded_img = decoder(encoded_img)
# 将挖空的区域和原始图像计算空洞损失
hole_loss = tf.reduce_mean(tf.multiply(tf.square(input_img - decoded_img), 1 - mask))
# 计算重建误差
recon_loss = tf.reduce_mean(tf.multiply(tf.square(input_img - decoded_img), mask))
# 总损失为重建误差加上空洞损失
total_loss = recon_loss + 0.2 * hole_loss
return total_loss, decoded_img
# 加载 MNIST 数据集
from tensorflow.examples.tutorials.mnist import input_data
mnist = input_data.read_data_sets("MNIST_data/", one_hot=True)
# 定义输入占位符
input_img = tf.placeholder(tf.float32, shape=[None, 28, 28, 1])
mask = tf.placeholder(tf.float32, shape=[None, 28, 28, 1])
# 定义空洞自编码器模型
total_loss, decoded_img = autoencoder(input_img, mask)
# 定义优化器
optimizer = tf.train.AdamOptimizer(learning_rate=0.001).minimize(total_loss)
# 训练模型
with tf.Session() as sess:
sess.run(tf.global_variables_initializer())
for i in range(10000):
batch_x, _ = mnist.train.next_batch(64)
batch_x = batch_x.reshape(-1, 28, 28, 1)
# 随机生成挖空区域的掩码
mask_array = tf.random_uniform(shape=[batch_x.shape[0], 28, 28, 1], minval=0, maxval=1)
_, loss = sess.run([optimizer, total_loss], feed_dict={input_img: batch_x, mask: mask_array.eval()})
if i % 1000 == 0:
print("Step %d, Loss %g" % (i, loss))
# 测试模型
test_x, _ = mnist.test.next_batch(10)
test_x = test_x.reshape(-1, 28, 28, 1)
# 随机生成挖空区域的掩码
mask_array = tf.random_uniform(shape=[test_x.shape[0], 28, 28, 1], minval=0, maxval=1)
masked_images = tf.multiply(test_x, mask_array)
decoded_images = sess.run(decoded_img, feed_dict={input_img: test_x, mask: mask_array.eval()})
```
D:\python\python3.9.5\pythonProject\venv\Scripts\python.exe C:/Users/马斌/Desktop/cnn测试/cnn-lstm改.py 本车ID 时间 总帧数 全局时间 ... 原车道后车x坐标 原车道后车y坐标 原车道后车速度 原车道后车加速度 1595 1499 7053 1604 1.113440e+12 ... 56.283 1602.157 15.27 -2.61 1596 1499 7054 1604 1.113440e+12 ... 56.294 1603.665 15.07 -1.53 1597 1499 7055 1604 1.113440e+12 ... 56.304 1605.152 14.99 0.24 1598 1499 7056 1604 1.113440e+12 ... 56.314 1606.649 15.03 0.64 1599 1499 7057 1604 1.113440e+12 ... 56.325 1608.171 14.95 -1.65 [5 rows x 27 columns] Traceback (most recent call last): File "C:\Users\马斌\Desktop\cnn测试\cnn-lstm改.py", line 97, in <module> myModel = cnn_lstm_model() # 建立模型 File "C:\Users\马斌\Desktop\cnn测试\cnn-lstm改.py", line 78, in cnn_lstm_model inputs = Input(shape=(windows, amount_of_features)) File "D:\python\python3.9.5\pythonProject\venv\lib\site-packages\keras\utils\traceback_utils.py", line 70, in error_handler raise e.with_traceback(filtered_tb) from None File "D:\python\python3.9.5\pythonProject\venv\lib\site-packages\tensorflow\python\framework\tensor_shape.py", line 214, in __init__ raise TypeError( TypeError: Dimension value must be integer or None or have an __index__ method, got value '(4,)' with type '<class 'tuple'>' 本车加速度 车头间距 原车道前车速度 本车速度 1554 0.00 43.58 17.68 11.75 1555 0.00 44.29 17.82 11.75 1556 0.00 45.07 17.82 11.75 1557 0.08 45.39 17.82 11.76 1558 0.43 45.72 17.82 11.79 (1401, 2, 4) (156, 2, 4) (1401,) (156,) Process finished with exit code 1
这段代码是一个程序的运行结果,其中包含了一些错误信息和数据信息。根据错误信息,可以看出是在创建模型时出现了问题,具体来说是在创建模型输入层时,出现了一个维度设置错误。根据错误信息中的提示,`inputs = Input(shape=(windows, amount_of_features))` 这行代码可能是出错的位置。
错误信息中还包含了一些数据信息,例如 `(1401, 2, 4) (156, 2, 4) (1401,) (156,)` 这一行数据,可能是程序中的一些数据维度信息。根据这些信息和程序代码的上下文,可以推测这是一个使用 Keras 框架构建 CNN-LSTM 模型的程序,输入数据的形状为 `(batch_size, windows, amount_of_features)`,其中 `batch_size` 表示批次大小,`windows` 表示时间窗口大小,`amount_of_features` 表示特征数量。
需要进一步检查代码并解决错误,才能使程序正常运行。
相关推荐
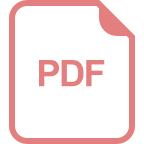












