np.argmax能用于tensor吗
时间: 2023-11-30 15:02:42 浏览: 55
可以,`np.argmax`可以用于numpy数组和tensor。如果你想在PyTorch中使用`argmax`函数,可以使用`torch.argmax`函数。例如,如果你有一个PyTorch张量`x`,并想找到它的最大值,你可以这样做:
```
import torch
x = torch.tensor([[1, 2, 3], [4, 5, 6]])
max_indices = torch.argmax(x)
print(max_indices) # 输出:tensor(5)
```
如果你想在TensorFlow中使用`argmax`函数,可以使用`tf.argmax`函数。例如,如果你有一个TensorFlow张量`x`,并想找到它的最大值,你可以这样做:
```
import tensorflow as tf
x = tf.constant([[1, 2, 3], [4, 5, 6]])
max_indices = tf.argmax(x)
print(max_indices) # 输出:tf.Tensor([1 1 1], shape=(3,), dtype=int64)
```
需要注意的是,这两个函数返回的最大值索引的形状可能是不同的,具体取决于输入张量的形状。
相关问题
TracerWarning: Converting a tensor to a NumPy array might cause the trace to be incorrect. We can't record the data flow of Python values, so this value will be treated as a constant in the future. This means that the trace might not generalize to other inputs! idx = np.argmax(valid_pose.detach().numpy())
这个警告的含义是将一个tensor转换为numpy数组可能会导致追踪记录不正确。在未来的版本中,这个值将被视为常数,这意味着追踪记录可能无法推广到其他输入!
这个警告是因为在使用`valid_pose.detach().numpy()`将一个tensor转换为numpy数组时,PyTorch无法追踪记录数据流。因此,这个值将被视为常数,而不是一个可追踪的变量。如果将这个常数用于后续的计算,可能会导致追踪记录不正确,从而影响模型的训练和预测结果。
为了避免这个问题,建议在PyTorch环境中使用tensor进行计算,而不是将tensor转换为numpy数组。如果确实需要将tensor转换为numpy数组进行计算,可以使用`torch.tensor()`将numpy数组转换为tensor,这样可以避免出现追踪记录不正确的问题。例如:
```python
import numpy as np
import torch
valid_pose = torch.Tensor([1, 2, 3, 4, 5])
idx = torch.argmax(valid_pose.detach())
idx_np = np.array([idx.item()])
```
在上面的代码中,我们使用`idx.item()`将tensor中的单个元素提取出来,然后使用`np.array()`将其转换为numpy数组。这种方式可以避免出现追踪记录不正确的问题。
torch.argmin
引用\[1\]:1、两个框架的argmin等价; 2、torch.min包含了argmin和两个tensor对比这两个功能,分别对应numpy中的np.argmin和np.minimun; 3、torch.min包含了torch.argmin的功能。 引用\[2\]:torch.argmin 返回在指定维度方向上最小值的索引。 引用\[3\]:例2——不指定维度,返回整体上最大值的序号。
根据引用\[2\]和引用\[3\]的内容,torch.argmin函数用于返回在指定维度方向上最小值的索引。如果不指定维度,则返回整体上最小值的索引。例如,对于一个3x3的张量a,使用torch.argmin(a, dim=1)将返回每行最小值的索引,而使用torch.argmin(a)将返回整个张量中最小值的索引。
#### 引用[.reference_title]
- *1* *2* [Numpy与Pytorch对比(一)最大/最小值寻找](https://blog.csdn.net/sinat_37145472/article/details/94753866)[target="_blank" data-report-click={"spm":"1018.2226.3001.9630","extra":{"utm_source":"vip_chatgpt_common_search_pc_result","utm_medium":"distribute.pc_search_result.none-task-cask-2~all~insert_cask~default-1-null.142^v91^insertT0,239^v3^insert_chatgpt"}} ] [.reference_item]
- *3* [PyTorch:torch.max、min、argmax、argmin](https://blog.csdn.net/Flag_ing/article/details/109128931)[target="_blank" data-report-click={"spm":"1018.2226.3001.9630","extra":{"utm_source":"vip_chatgpt_common_search_pc_result","utm_medium":"distribute.pc_search_result.none-task-cask-2~all~insert_cask~default-1-null.142^v91^insertT0,239^v3^insert_chatgpt"}} ] [.reference_item]
[ .reference_list ]
相关推荐
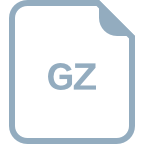
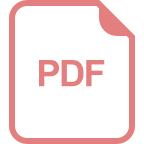
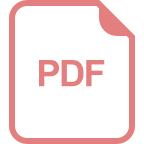












