联邦学习中这些的作用train_state = training_process.initialize()
时间: 2023-10-13 15:05:38 浏览: 34
train_state = training_process.initialize() 是联邦学习中的一个步骤,用于初始化联邦学习过程。在这个过程中,每个参与者(例如设备或客户端)都会执行一些本地的训练,然后将权重和梯度信息分享给中央服务器。服务器收到这些信息后,会将它们整合起来,计算出新的权重和梯度更新,然后将它们发送回到每个参与者。train_state = training_process.initialize() 的作用是初始化这个过程中的一些参数,为后续的联邦学习过程提供支持。具体来说,它会生成一些用于控制联邦学习过程的状态变量,并将它们保存在 train_state 变量中,以便后续使用。
相关问题
解释代码: def __init__(self, X, n_components, start_df, random_state, init_type): self.loc_, self.scale_, self.scale_inv_chole_, self.scale_chole_ = \ self.initialize_params(X, n_components, random_state, init_type) self.df_ = np.full(shape=n_components, fill_value=start_df) self.resp = None self.a_nm, self.b_nm = None, None self.E_gamma = None self.E_log_gamma = None self.E_log_weights = None self.wishart_vm = None self.kappa_m = None self.eta_m = None
这段代码定义了一个 Python 类的初始化方法 `__init__`。这个方法接收参数 `X`,`n_components`,`start_df`,`random_state` 和 `init_type`。
初始化方法会使用 `initialize_params` 方法对 `self.loc_`,`self.scale_`,`self.scale_inv_chole_` 和 `self.scale_chole_` 进行赋值。
然后,`self.df_` 将使用 `np.full` 方法创建一个 shape 为 `n_components`,元素都是 `start_df` 的数组。
其他变量 `self.resp`,`self.a_nm`,`self.b_nm`,`self.E_gamma`,`self.E_log_gamma`,`self.E_log_weights`,`self.wishart_vm`,`self.kappa_m` 和 `self.eta_m` 都被初始化为 `None`。
void initialize(const Camera<S> &camera, const noiseParams<S> &noise_params, const MSCKFParams<S> &msckf_params, const imuState<S> &imu_state) { // Constructor: camera_ = camera; noise_params_ = noise_params; msckf_params_ = msckf_params; num_feature_tracks_residualized_ = 0; imu_state_ = imu_state; pos_init_ = imu_state_.p_I_G; imu_state_.p_I_G_null = imu_state_.p_I_G; imu_state_.v_I_G_null = imu_state_.v_I_G; imu_state_.q_IG_null = imu_state_.q_IG; imu_covar_ = noise_params.initial_imu_covar; last_feature_id_ = 0; // Initialize the chi squared test table with confidence // level 0.95. chi_squared_test_table.resize(99); for (int i = 1; i < 100; ++i) { boost::math::chi_squared chi_squared_dist(i); chi_squared_test_table[i - 1] = boost::math::quantile(chi_squared_dist, 0.05); } // TODO: Adjust for 0-sized covar? }
这是一段 C++ 代码,其中定义了一个名为 initialize 的函数,该函数接受四个参数:相机对象 camera,噪声参数对象 noise_params,MSCKF 参数对象 msckf_params,IMU 状态对象 imu_state。
函数的作用是初始化 MSCKF(Multi-State Constraint Kalman Filter)对象。在函数体内,首先将传入的参数赋值给相应的成员变量;然后将一些状态量初始化;接着计算卡方检验表,用于后面的特征点跟踪;最后,TODO 表示还需要进行一些调整。
具体的实现需要查看更多代码才能确定。
相关推荐
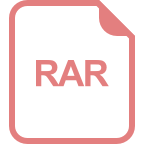













