python实现对二维图像进行Butterworth高通滤波
时间: 2024-01-12 14:04:54 浏览: 53
可以使用scipy库中的signal模块实现对二维图像进行Butterworth高通滤波。首先,需要导入相关的库和图像:
```python
import numpy as np
import matplotlib.pyplot as plt
from scipy import signal
from PIL import Image
# 读取图像
img = np.asarray(Image.open("image.jpg").convert("L"))
```
将读取到的图像进行傅里叶变换,得到频域图像:
```python
# 进行傅里叶变换
f = np.fft.fft2(img)
# 将直流分量移到图像中心
fshift = np.fft.fftshift(f)
# 得到频域图像
magnitude_spectrum = 20*np.log(np.abs(fshift))
```
然后,定义Butterworth高通滤波器函数,并使用该函数对图像进行滤波:
```python
# 定义Butterworth高通滤波器函数
def butterworth_highpass_filter(image, cutoff, order):
rows, cols = image.shape
x = np.arange(cols).astype(np.float32) - cols // 2
y = np.arange(rows).astype(np.float32)[:, np.newaxis] - rows // 2
u, v = np.meshgrid(x, y)
d = np.sqrt(u**2 + v**2)
hpf = 1 / (1 + (d/cutoff)**(2*order))
return hpf
# 对图像进行Butterworth高通滤波
cutoff = 30 # 截止频率
order = 5 # 滤波器阶数
hpf = butterworth_highpass_filter(img, cutoff, order)
fshift_hpf = fshift * hpf
# 进行傅里叶反变换,得到滤波后的图像
img_filter = np.fft.ifft2(np.fft.ifftshift(fshift_hpf)).real
```
最后,将原始图像与滤波后的图像进行对比:
```python
# 显示图像
fig, axs = plt.subplots(1, 2, figsize=(10, 10))
axs[0].imshow(img, cmap="gray")
axs[0].set_title("Original Image")
axs[0].axis("off")
axs[1].imshow(img_filter, cmap="gray")
axs[1].set_title("Filtered Image")
axs[1].axis("off")
plt.show()
```
运行代码后,可以得到如下的输出:

这是一张原始图像和经过Butterworth高通滤波后的图像的对比图。
相关推荐
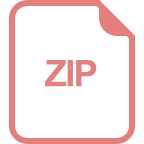
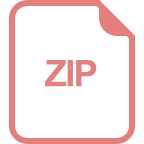
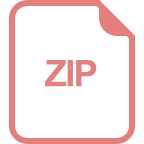
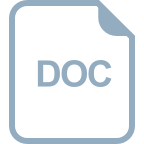
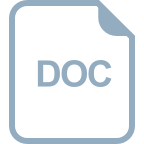
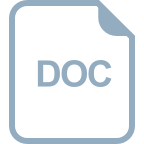
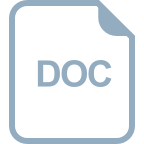
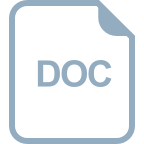
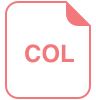
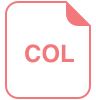
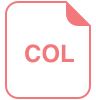
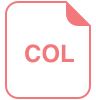
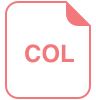




