模拟退火算法Python
时间: 2023-11-05 15:22:29 浏览: 122
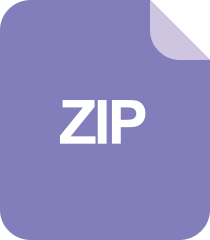
模拟退火算法python.zip
模拟退火算法是一种现代优化算法,它基于蒙特卡洛迭代求解方法的随机寻优算法。它最早于1983年成功地应用到组合优化领域。与固体物理退火过程的相似性相结合,模拟退火算法通过模拟固体物质的退火过程来获得问题的最优解。
在Python中,可以使用模拟退火算法来解决各种优化问题。通常,实现模拟退火算法的Python代码包括以下几个步骤:
1. 初始化解决方案,可以是随机生成的或者根据问题的特点来设定的。
2. 设定初始温度和降温速率,初始温度越高,搜索的范围越广,但可能会错过最优解;降温速率越慢,搜索的时间越长,但可能会找到更好的解。
3. 迭代地进行退火过程,每次迭代中,通过改变解决方案的邻域来尝试找到更优的解,接受一定概率的差解,以避免陷入局部最优解。
4. 当达到停止条件时(例如达到最大迭代次数或达到一定的温度),停止迭代,返回最优解。
在Python中,可以使用NumPy和Matplotlib等库来实现模拟退火算法,并进行可视化。代码示例可以参考引用中的代码。在该示例中,参数如初始温度、降温速率、迭代次数、解决方案的长度等都需要根据具体问题进行调整。<span class="em">1</span><span class="em">2</span><span class="em">3</span>
阅读全文
相关推荐
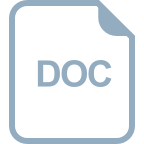
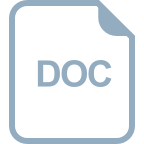


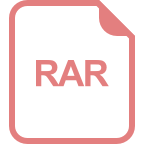









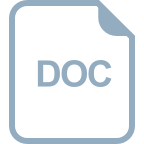
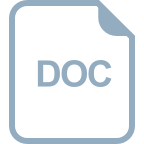