如何在Matlab中实现K-means聚类算法?
时间: 2024-06-14 19:03:47 浏览: 141
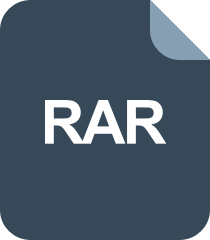
用matlab语言实现K-MEANS聚类算法
```matlab
% 三维数据分类的K-means聚类算法示例
% 假设数据存储在变量X中,包含三列分别代表三个维度的数据
% 假设要将数据分为k个类别
% 设置聚类的个数
k = 3;
% 使用k-means算法进行聚类
[idx, C] = kmeans(X, k);
% idx包含了每个数据点所属的类别
% C包含了每个类别的中心点坐标
```
阅读全文
相关推荐
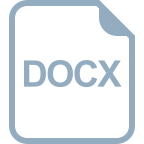
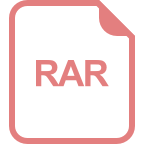
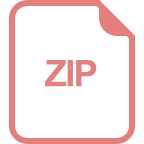
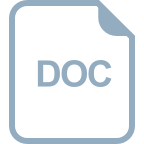
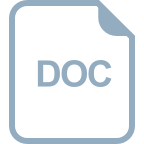
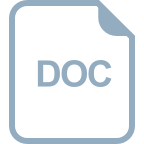
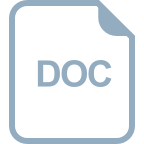
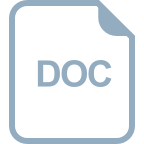
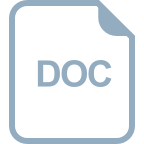
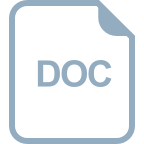
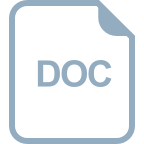
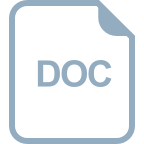



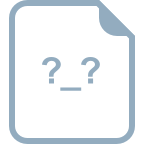