w_tmp = np.random.rand(X_tmp.shape[1]).reshape(-1,)-0.5 什么意思
时间: 2024-04-19 22:25:18 浏览: 191
这行代码的意思是,创建一个形状与X_tmp的列数相同的随机数数组,并将其值给变量w_tmp。np.random.rand()函数会生成位于[0, 1)之间的随机数。reshape(-1,)的作用是将数组的形状调整为1维,-0.5是将数组中的每个元素都减去0.5。最终得到的w_tmp是一个形状为(6,)的一维数组,其中的元素为随机数减去0.5的结果。
相关问题
import numpy as np import pandas as pd def localmin(points, pixel_size): x_min = np.min(points[:, 0]) y_min = np.min(points[:, 1]) x_max = np.max(points[:, 0]) y_max = np.max(points[:, 1]) w = x_max - x_min h = y_max - y_min wn = w // pixel_size + 1 hn = h // pixel_size + 1 x_bins = np.array([i * pixel_size for i in range(int(wn + 1))]) y_bins = np.array([i * pixel_size for i in range(int(hn + 1))]) df = pd.DataFrame(points, columns=['x', 'y', 'z', 'classification', 'indices']) df['x_bin'] = pd.cut(df['x'], bins=x_bins) df['y_bin'] = pd.cut(df['y'], bins=y_bins) result = df.groupby(['x_bin', 'y_bin']).apply(lambda x: x.loc[x['z'].idxmin()])[['x', 'y', 'z', 'classification', 'indices']] return result a = np.random.random([100,3]) b = np.random.random([100, 1])//0.5 c = np.arange(1000).reshape([100,1]) a = np.concatenate([a,b,c],axis=-1) d = localmin(a,0.2) 如何从a中删除d中的点
可以使用pandas的isin()方法来判断a中的点是否出现在d中,然后使用~运算符将不包含在d中的点筛选出来。具体实现代码如下:
```python
import numpy as np
import pandas as pd
def localmin(points, pixel_size):
x_min = np.min(points[:, 0])
y_min = np.min(points[:, 1])
x_max = np.max(points[:, 0])
y_max = np.max(points[:, 1])
w = x_max - x_min
h = y_max - y_min
wn = w // pixel_size + 1
hn = h // pixel_size + 1
x_bins = np.array([i * pixel_size for i in range(int(wn + 1))])
y_bins = np.array([i * pixel_size for i in range(int(hn + 1))])
df = pd.DataFrame(points, columns=['x', 'y', 'z', 'classification', 'indices'])
df['x_bin'] = pd.cut(df['x'], bins=x_bins)
df['y_bin'] = pd.cut(df['y'], bins=y_bins)
result = df.groupby(['x_bin', 'y_bin']).apply(lambda x: x.loc[x['z'].idxmin()])[['x', 'y', 'z', 'classification', 'indices']]
return result
a = np.random.random([100,3])
b = np.random.random([100, 1])//0.5
c = np.arange(1000).reshape([100,1])
a = np.concatenate([a,b,c],axis=-1)
d = localmin(a,0.2)
# 筛选出不包含在d中的点并重新赋值给a
a = a[~a[:, :2].isin(d.iloc[:, :2].values).any(axis=1)]
```
其中,isin()方法用于判断一个DataFrame或Series对象中的值是否在另一个DataFrame或Series对象中出现过。在这里我们用isin()方法来判断a中的前两列(即x和y坐标)是否出现在d中,然后使用any()方法将结果沿着列方向合并,得到一个长度为100的布尔型数组,表示a中每个点是否在d中出现过。最后使用~运算符对数组进行取反,得到不包含在d中的点的布尔型数组,然后使用该数组对a进行筛选即可。
翻译这段程序并自行赋值调用:import matplotlib.pyplot as plt import numpy as np import sklearn import sklearn.datasets import sklearn.linear_model def plot_decision_boundary(model, X, y): # Set min and max values and give it some padding x_min, x_max = X[0, :].min() - 1, X[0, :].max() + 1 y_min, y_max = X[1, :].min() - 1, X[1, :].max() + 1 h = 0.01 # Generate a grid of points with distance h between them xx, yy = np.meshgrid(np.arange(x_min, x_max, h), np.arange(y_min, y_max, h)) # Predict the function value for the whole grid Z = model(np.c_[xx.ravel(), yy.ravel()]) Z = Z.reshape(xx.shape) # Plot the contour and training examples plt.contourf(xx, yy, Z, cmap=plt.cm.Spectral) plt.ylabel('x2') plt.xlabel('x1') plt.scatter(X[0, :], X[1, :], c=y, cmap=plt.cm.Spectral) def sigmoid(x): s = 1/(1+np.exp(-x)) return s def load_planar_dataset(): np.random.seed(1) m = 400 # number of examples N = int(m/2) # number of points per class print(np.random.randn(N)) D = 2 # dimensionality X = np.zeros((m,D)) # data matrix where each row is a single example Y = np.zeros((m,1), dtype='uint8') # labels vector (0 for red, 1 for blue) a = 4 # maximum ray of the flower for j in range(2): ix = range(Nj,N(j+1)) t = np.linspace(j3.12,(j+1)3.12,N) + np.random.randn(N)0.2 # theta r = anp.sin(4t) + np.random.randn(N)0.2 # radius X[ix] = np.c_[rnp.sin(t), rnp.cos(t)] Y[ix] = j X = X.T Y = Y.T return X, Y def load_extra_datasets(): N = 200 noisy_circles = sklearn.datasets.make_circles(n_samples=N, factor=.5, noise=.3) noisy_moons = sklearn.datasets.make_moons(n_samples=N, noise=.2) blobs = sklearn.datasets.make_blobs(n_samples=N, random_state=5, n_features=2, centers=6) gaussian_quantiles = sklearn.datasets.make_gaussian_quantiles(mean=None, cov=0.5, n_samples=N, n_features=2, n_classes=2, shuffle=True, random_state=None) no_structure = np.random.rand(N, 2), np.random.rand(N, 2) return noisy_circles, noisy_moons, blobs, gaussian_quantiles, no_structure
这段程序是一个分类模型的辅助函数,包括了绘制决策边界、sigmoid函数和加载数据集的函数。具体实现如下:
```python
import matplotlib.pyplot as plt
import numpy as np
import sklearn
import sklearn.datasets
import sklearn.linear_model
def plot_decision_boundary(model, X, y):
# 设置最小值和最大值,并给它们一些填充
x_min, x_max = X[0, :].min() - 1, X[0, :].max() + 1
y_min, y_max = X[1, :].min() - 1, X[1, :].max() + 1
h = 0.01
# 生成一个网格,网格中点的距离为h
xx, yy = np.meshgrid(np.arange(x_min, x_max, h), np.arange(y_min, y_max, h))
# 对整个网格预测函数值
Z = model(np.c_[xx.ravel(), yy.ravel()])
Z = Z.reshape(xx.shape)
# 绘制轮廓和训练样本
plt.contourf(xx, yy, Z, cmap=plt.cm.Spectral)
plt.ylabel('x2')
plt.xlabel('x1')
plt.scatter(X[0, :], X[1, :], c=y, cmap=plt.cm.Spectral)
def sigmoid(x):
s = 1 / (1 + np.exp(-x))
return s
def load_planar_dataset():
np.random.seed(1)
m = 400 # 样本数量
N = int(m / 2) # 每个类的样本数量
# 生成数据集
D = 2 # 特征维度
X = np.zeros((m, D)) # 特征矩阵
Y = np.zeros((m, 1), dtype='uint8') # 标签向量
a = 4 # 花的最大半径
for j in range(2):
ix = range(N*j, N*(j+1))
t = np.linspace(j*3.12, (j+1)*3.12, N) + np.random.randn(N)*0.2 # theta
r = a*np.sin(4*t) + np.random.randn(N)*0.2 # radius
X[ix] = np.c_[r*np.sin(t), r*np.cos(t)]
Y[ix] = j
X = X.T
Y = Y.T
return X, Y
def load_extra_datasets():
N = 200
noisy_circles = sklearn.datasets.make_circles(n_samples=N, factor=.5, noise=.3)
noisy_moons = sklearn.datasets.make_moons(n_samples=N, noise=.2)
blobs = sklearn.datasets.make_blobs(n_samples=N, random_state=5, n_features=2, centers=6)
gaussian_quantiles = sklearn.datasets.make_gaussian_quantiles(mean=None, cov=0.5, n_samples=N, n_features=2, n_classes=2, shuffle=True, random_state=None)
no_structure = np.random.rand(N, 2), np.random.rand(N, 2)
return noisy_circles, noisy_moons, blobs, gaussian_quantiles, no_structure
```
这段程序中包含了以下函数:
- `plot_decision_boundary(model, X, y)`:绘制分类模型的决策边界,其中`model`是分类模型,`X`是特征矩阵,`y`是标签向量。
- `sigmoid(x)`:实现sigmoid函数。
- `load_planar_dataset()`:加载一个二维的花瓣数据集。
- `load_extra_datasets()`:加载五个其他数据集。
阅读全文
相关推荐
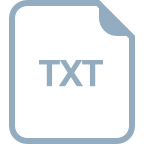
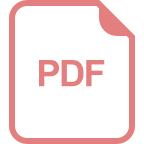
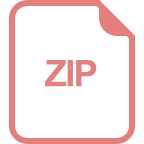














