doc doesn't support values of type: START_ARRAY
时间: 2024-04-16 16:25:03 浏览: 12
这个错误通常表示在文档中使用了一个不支持的数据类型:START_ARRAY(开始数组)。在许多情况下,这可能是由于尝试将一个数组作为不允许数组的值传递给某个方法或函数。
要解决这个问题,可以检查代码中的相关部分,并确保正确处理数组类型的值。可能需要查看文档或参考相关的示例代码来了解如何正确使用该方法或函数。如果您可以提供更多的上下文或代码示例,我可以提供更具体的帮助。
相关问题
xml确定是utf-8编码的文件,用pugi::xml_document doc;pugi::xml_parse_result result = doc.load_file("example.xml", pugi::parse_default, pugi::encoding_utf8);解析,中文乱码
在使用pugixml库解析xml文件时,需要确保编码格式正确,否则就会出现中文乱码的情况。
如果你已经确定了xml文件的编码格式为UTF-8,但还是出现中文乱码的情况,可以尝试以下几种方法:
1. 确认xml文件中的中文字符是否是utf-8编码,如果不是,需要将其转换为utf-8编码。
2. 在调用load_file函数时,可以指定编码格式为pugi::encoding_auto,让pugixml库自动检测编码格式:
```
pugi::xml_document doc;
pugi::xml_parse_result result = doc.load_file("example.xml", pugi::parse_default, pugi::encoding_auto);
```
3. 如果以上两种方法都没有解决问题,可以尝试使用pugi::xml_parse_result对象的encoding函数获取实际的编码格式:
```
pugi::xml_document doc;
pugi::xml_parse_result result = doc.load_file("example.xml", pugi::parse_default, pugi::encoding_utf8);
if (result) {
std::string encoding = result.encoding;
// 如果实际编码格式与指定的不同,可以重新加载文件并指定正确的编码格式
}
```
希望这些方法可以帮助你解决中文乱码的问题。
变量:开始时间start_time,结束时间end_time 查询表:SALES_ORDER_DOC 条件:start_time<=SALES_ORDER_DOC.ORDER_DATE<=end_time 计算:所有符合条件的数据中AMT_UNINCLUDE_TAX_OC的和
好的,根据您的要求,以下是一段适用于MySQL数据库的SQL语句,用于查询SALES_ORDER_DOC表中符合条件的数据,并计算AMT_UNINCLUDE_TAX_OC列的总和:
```
SELECT SUM(AMT_UNINCLUDE_TAX_OC) AS total_amount
FROM SALES_ORDER_DOC
WHERE ORDER_DATE BETWEEN start_time AND end_time;
```
请注意,上述SQL语句中的start_time和end_time均为变量,需要在实际应用时进行替换。另外,ORDER_DATE列的数据类型应为日期型或时间戳型。如果您在实际应用中遇到了任何问题,请随时与我联系。
相关推荐
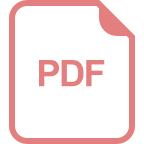












