opencv c++灰度图像阈值处理
时间: 2024-09-30 09:00:54 浏览: 40
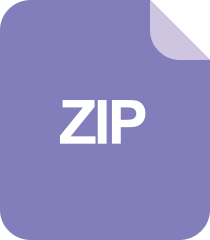
C++ - opencv - 图像增强 - 文本图像增强

在OpenCV库中,C++处理灰度图像的阈值操作通常涉及以下几个步骤:
1. **读取图像**:首先,你需要使用`cv::imread()`函数从文件或内存中加载灰度图像。例如:
```cpp
cv::Mat grayImage = cv::imread("gray_image.jpg", cv::IMREAD_GRAYSCALE);
```
2. **设置阈值**:你可以使用`cv::threshold()`函数对图像进行二值化。这个函数需要输入图像、输出图像、阈值范围以及阈值选择的方式(如高于阈值设为白色,低于设为黑色):
```cpp
cv::Mat binaryImage;
cv::threshold(grayImage, binaryImage, thresholdValue, 255, cv::THRESH_BINARY | cv::THRESH_OTSU); // 自动计算最佳阈值
```
这里的`cv::THRESH_BINARY`表示二进制阈值分割,`cv::THRESH_OTSU`则用于自动确定最佳阈值。
3. **结果分析**:处理后的`binaryImage`是一个二值图像,其中像素值要么是0(黑),要么是255(白)。你可以进一步分析这个图像,比如边缘检测或形状识别。
阅读全文
相关推荐
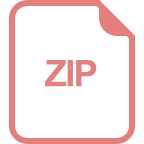
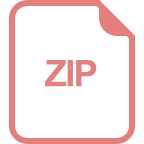
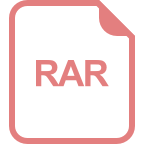
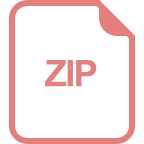
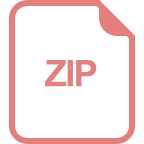
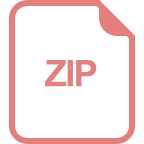
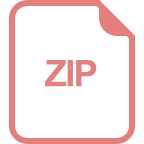
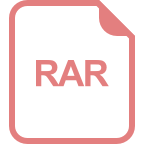
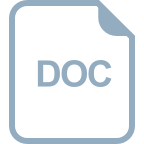
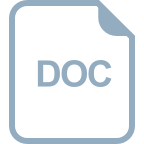
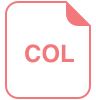






