idx pytorch
时间: 2023-08-08 07:08:49 浏览: 83
PyTorch中的idx代表索引(index),它通常用于访问张量(Tensor)中的元素。您可以使用idx来获取张量中特定位置的值。例如,如果有一个名为"tensor"的张量,您可以使用以下代码获取第一个元素的值:
```
value = tensor[0]
```
这将返回张量中索引为0的元素的值。同样地,您还可以使用idx获取其他位置的元素。希望这能回答您的问题!如果还有其他问题,请随时提问。
相关问题
pytorch ONNX
Pytorch是一个深度学习框架,而ONNX是一种开放的神经网络交换格式。Pytorch可以通过torch.onnx.export函数将模型转换为ONNX格式。然而,Pytorch自带的torch.onnx.export转换得到的ONNX,ONNXRuntime需要的ONNX,TensorRT需要的ONNX都是不同的。这意味着不同的工具和库对ONNX格式有不同的要求和支持。因此,在将Pytorch模型转换为ONNX时,需要注意不同库之间的兼容性和差异。
对于Pytorch转换为ONNX的过程,由于Pytorch没有图的概念,所以需要通过让ONNX记录模型运行的结果来抽象成一张图。这样的转换过程有两个天然的局限。另外,Pytorch的MaxUnpool实现和ONNXRuntime对输入的Idx格式要求也不同,因此需要对输入的Idx做额外的处理,以便在ONNXRuntime中获得相同的结果。
resnet pytorch
ResNet (Residual Neural Network) is a popular deep learning architecture commonly used for image classification tasks. It was introduced by Kaiming He et al. in 2015. ResNet uses residual connections to allow the network to learn residual mappings, making it easier to train very deep networks.
To implement ResNet in PyTorch, you can use the torchvision library, which provides pre-trained ResNet models as well as the ability to create custom ResNet architectures.
Here is an example of how to use the torchvision library to load a pre-trained ResNet model and perform image classification:
```python
import torch
import torchvision.models as models
import torchvision.transforms as transforms
from PIL import Image
# Load the pre-trained ResNet model
resnet = models.resnet50(pretrained=True)
# Preprocess the input image
transform = transforms.Compose([
transforms.Resize(256),
transforms.CenterCrop(224),
transforms.ToTensor(),
transforms.Normalize(mean=[0.485, 0.456, 0.406], std=[0.229, 0.224, 0.225])
])
# Load and preprocess the image
image = Image.open("image.jpg")
input_tensor = transform(image)
input_batch = input_tensor.unsqueeze(0)
# Move the input tensor to the GPU if available
device = torch.device("cuda" if torch.cuda.is_available() else "cpu")
input_batch = input_batch.to(device)
# Set the model to evaluation mode
resnet.eval()
# Make predictions
with torch.no_grad():
input_batch = input_batch.to(device)
output = resnet(input_batch)
# Load the class labels
with open("imagenet_classes.txt") as f:
class_labels = [line.strip() for line in f.readlines()]
# Get the predicted class label
_, predicted_idx = torch.max(output, 1)
predicted_label = class_labels[predicted_idx.item()]
# Print the predicted label
print("Predicted label: ", predicted_label)
```
In the above code, the input image is preprocessed using the same transformations used during training of the ResNet model. The pre-trained ResNet model is loaded, and the input image is passed through the model to get predictions. The predicted class label is then obtained and printed.
Note: Make sure to replace "image.jpg" with the path to your input image and "imagenet_classes.txt" with the path to the class labels file corresponding to the pre-trained ResNet model you are using.
阅读全文
相关推荐
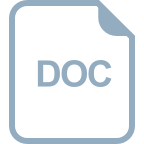
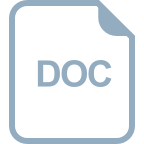
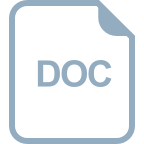
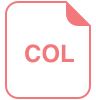












