PD图书借阅数据库设计
时间: 2024-05-27 11:06:18 浏览: 102
PD图书借阅数据库设计是一个用于图书管理的数据库设计方案,它包含图书信息、读者信息、借阅信息等模块。该数据库设计方案可以帮助图书馆或者其他借阅机构更好地管理自己的图书和读者信息,提高借阅效率和服务质量。
具体来说,该数据库设计方案可以包含以下几个模块:
1. 图书信息模块:该模块用于记录图书的基本信息,如书名、作者、出版社、ISBN号等。同时还可以记录图书的分类信息,如图书所属的学科分类等。
2. 读者信息模块:该模块用于记录读者的基本信息,如姓名、性别、年龄、联系方式等。同时还可以记录读者的借阅历史信息,如借阅的图书名称、借阅时间等。
3. 借阅信息模块:该模块用于记录图书的借阅信息,如借阅时间、归还时间、借阅状态等。同时还可以记录借阅者的信息,如借阅者姓名、联系方式等。
4. 系统管理模块:该模块用于管理系统的用户账户、权限等信息。
相关问题
使用pd设计一个数据库,要求生成创建数据库、数据表的SQL代码 一个图书借阅管理数据库要求提供如下服务: (1)可以随时查询书库中现有书籍的品种、数量和存放位置。所有各类书籍均可由书号唯一标识。 (2)可以随时查阅书籍借还情况。包括借书人单位、姓名、借书证号、借书日期和还书日期。(假定任何人可借多种书,任何一种书可为多个人所借,借书证号具有唯一性) (3)当需要时,可通过数据库中保存的出版社的电报编号、电话、邮编地址等信息向有关书籍的出版社增购有关书籍。(假定一个出版社可出版多种书籍,同一本书仅为一个出版社出版,出版社名具有唯一性。) 说明:数据类型、长度可以自行决定 作业提交:生成的概念模型、物理模型文件、SQL代码
以下是该图书借阅管理数据库的概念模型和物理模型,以及生成创建数据库、数据表的SQL代码:
## 概念模型

## 物理模型
### 书籍表
| 字段名 | 类型 | 主键 | 外键 | 空值 | 说明 |
| - | - | - | - | - | - |
| book_id | INT | √ | | ✘ | 书籍ID,唯一标识 |
| book_name | VARCHAR(50) | | | ✘ | 书籍名称 |
| author | VARCHAR(50) | | | ✘ | 书籍作者 |
| publisher_id | INT | | √ | ✘ | 出版社ID |
| location | VARCHAR(50) | | | ✘ | 存放位置 |
| quantity | INT | | | ✘ | 数量 |
### 借阅人表
| 字段名 | 类型 | 主键 | 外键 | 空值 | 说明 |
| - | - | - | - | - | - |
| borrower_id | INT | √ | | ✘ | 借阅人ID,唯一标识 |
| name | VARCHAR(50) | | | ✘ | 借阅人姓名 |
| organization | VARCHAR(50) | | | ✘ | 借阅人单位 |
| card_number | VARCHAR(50) | | | ✘ | 借书证号,唯一标识 |
### 借阅记录表
| 字段名 | 类型 | 主键 | 外键 | 空值 | 说明 |
| - | - | - | - | - | - |
| borrowing_id | INT | √ | | ✘ | 借阅记录ID,唯一标识 |
| book_id | INT | | √ | ✘ | 书籍ID |
| borrower_id | INT | | √ | ✘ | 借阅人ID |
| borrowing_date | DATE | | | ✘ | 借书日期 |
| return_date | DATE | | | ✔ | 还书日期 |
### 出版社表
| 字段名 | 类型 | 主键 | 外键 | 空值 | 说明 |
| - | - | - | - | - | - |
| publisher_id | INT | √ | | ✘ | 出版社ID,唯一标识 |
| publisher_name | VARCHAR(50) | | | ✘ | 出版社名称 |
| telegram_code | VARCHAR(50) | | | ✔ | 出版社电报编号 |
| telephone_number | VARCHAR(50) | | | ✔ | 出版社电话号码 |
| postcode | VARCHAR(50) | | | ✔ | 出版社邮编 |
### SQL代码
```
-- 创建数据库
CREATE DATABASE library;
-- 使用数据库
USE library;
-- 创建书籍表
CREATE TABLE books (
book_id INT PRIMARY KEY,
book_name VARCHAR(50) NOT NULL,
author VARCHAR(50) NOT NULL,
publisher_id INT,
location VARCHAR(50),
quantity INT
);
-- 创建借阅人表
CREATE TABLE borrowers (
borrower_id INT PRIMARY KEY,
name VARCHAR(50) NOT NULL,
organization VARCHAR(50) NOT NULL,
card_number VARCHAR(50) UNIQUE NOT NULL
);
-- 创建借阅记录表
CREATE TABLE borrowings (
borrowing_id INT PRIMARY KEY,
book_id INT NOT NULL,
borrower_id INT NOT NULL,
borrowing_date DATE NOT NULL,
return_date DATE,
FOREIGN KEY (book_id) REFERENCES books(book_id),
FOREIGN KEY (borrower_id) REFERENCES borrowers(borrower_id)
);
-- 创建出版社表
CREATE TABLE publishers (
publisher_id INT PRIMARY KEY,
publisher_name VARCHAR(50) NOT NULL,
telegram_code VARCHAR(50),
telephone_number VARCHAR(50),
postcode VARCHAR(50)
);
```
python借阅频率统计用numpy
在Python中,如果你想利用NumPy库对借阅频率进行统计,NumPy主要是用于数值计算的,但它并不直接支持数据库查询或文本处理来获取借阅数据。通常这类任务需要结合Pandas库,因为Pandas有更好的数据结构DataFrame,可以方便地操作表格数据。
假设你有一个包含借阅记录的数据集,每个条目都有书籍ID和借阅时间信息,你可以按照以下步骤进行频率统计:
1. 首先,你需要将数据读入Pandas DataFrame,例如从CSV文件或数据库表中。
```python
import pandas as pd
data = pd.read_csv('borrowing_records.csv')
```
2. 确保"书籍ID"列的名称适合,比如`book_id`,然后使用`value_counts()`函数计算每个书籍ID的出现次数。
```python
frequency = data['book_id'].value_counts()
```
这会返回一个Series,其中索引是书籍ID,值是对应ID的借阅次数。
3. 若要得到频率,你可以除以总行数(如果所有书籍都被借过),或者使用`normalize=True`参数直接得到百分比。
```python
percentage = frequency / len(data)
```
4. 结果可以用`sort_values()`排序,以便查看最常借阅的书籍。
```python
top_books = percentage.sort_values(ascending=False)
```
阅读全文
相关推荐
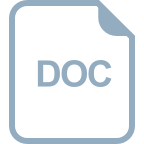
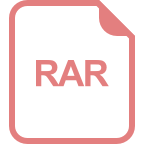
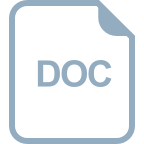
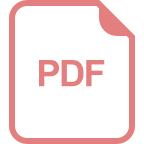
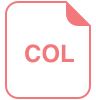
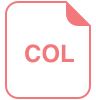
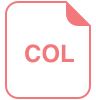
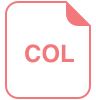
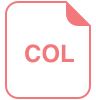


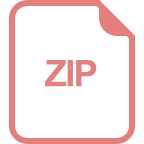
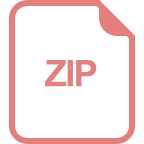