用python语言编写一个程序,使用logistic回归实现梯度下降算法,决定迭代次数,计算每次迭代并绘制结果。数据集通过txt文件输入且x和y的数据集彼此独立。不使用sklearn库的LogisticRegression模型
时间: 2024-12-20 19:30:35 浏览: 11
为了使用Python编写一个简单的逻辑回归模型,并实现梯度下降算法,我们可以创建一个包含sigmoid函数、代价函数、梯度函数以及更新步骤的类。首先,你需要安装numpy库,因为我们将使用它来进行数值计算。
```python
import numpy as np
class LogisticRegression:
def __init__(self, learning_rate=0.01, num_iterations=1000):
self.lr = learning_rate
self.num_iterations = num_iterations
self.weights = None
self.bias = None
def sigmoid(self, z):
return 1 / (1 + np.exp(-z))
def cost_function(self, X, y, weights, bias):
m = len(y)
z = np.dot(X, weights) + bias
h = self.sigmoid(z)
J = (-1/m) * (np.sum(y * np.log(h)) + np.sum((1 - y) * np.log(1 - h)))
return J
def gradient_descent(self, X, y):
m = X.shape[0]
dw = (1/m) * np.dot(X.T, (h - y))
db = (1/m) * np.sum(h - y)
self.weights -= self.lr * dw
self.bias -= self.lr * db
def fit(self, X, y):
X = np.insert(X, 0, 1, axis=1) # 添加偏置项
self.weights = np.zeros(X.shape[1])
self.bias = 0
for _ in range(self.num_iterations):
z = np.dot(X, self.weights) + self.bias
h = self.sigmoid(z)
self.gradient_descent(X, y)
def read_data_from_file(filename):
with open(filename, 'r') as file:
data = [line.strip().split(',') for line in file.readlines()]
X = np.array([list(map(float, row[:-1])) for row in data], dtype=np.float64)
y = np.array([float(row[-1]) for row in data])
return X, y
# 使用数据集
X, y = read_data_from_file('data.txt')
model = LogisticRegression()
model.fit(X, y)
# 每次迭代后的决策边界可视化(假设你可以用matplotlib)
# 这里由于代码限制,我们简化了绘图部分
# 实际上,你可能需要保存每个迭代点的权重,然后用它们画出线或曲线
# plot_interpolating_line(X, model.weights, model.bias, i+1)
# 结果
print("Weights after {} iterations:".format(model.num_iterations), model.weights)
print("Bias after {} iterations:".format(model.num_iterations), model.bias)
阅读全文
相关推荐
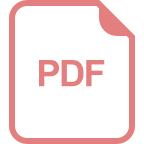
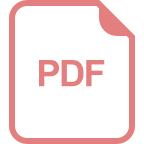
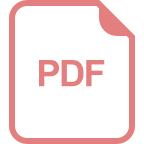


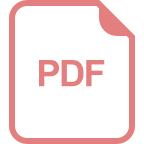
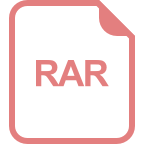
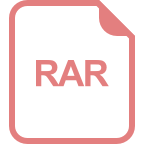
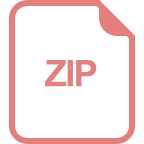
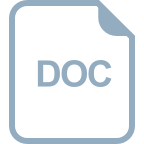
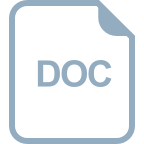
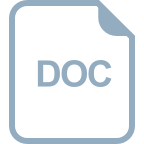
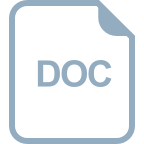
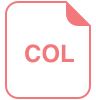
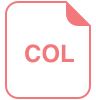
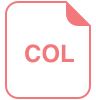
