spark实现二次排序
时间: 2023-10-27 08:06:09 浏览: 63
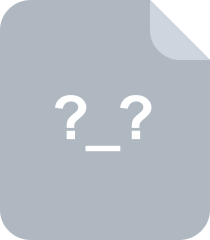
二级排序实现

Sure, I'd be happy to help you with that!
In Spark, secondary sorting can be achieved by using the sortByKey() transformation. To implement secondary sorting, we need to create a custom key class that implements the Ordered interface. In this key class, we can define the primary and secondary sort keys.
Here's an example:
First, let's create a class for our custom key:
```
class CustomKey(val primary: Int, val secondary: Int) extends Ordered[CustomKey] {
override def compare(that: CustomKey): Int = {
if (this.primary != that.primary) {
// sort by primary key
this.primary.compareTo(that.primary)
} else {
// sort by secondary key
this.secondary.compareTo(that.secondary)
}
}
}
```
This class takes two arguments, primary and secondary, which represent the two sort keys.
Next, let's create a sample RDD that we want to sort:
```
val data = sc.parallelize(Array((1, 5), (1, 1), (2, 3), (2, 1), (3, 2)))
```
In this RDD, the first element is the primary key and the second element is the secondary key.
Now, we can use the sortByKey() transformation to sort the RDD:
```
val sortedData = data.map{ case(primary, secondary) => (new CustomKey(primary, secondary), (primary, secondary)) }
.sortByKey()
.map{ case(customKey, (primary, secondary)) => (primary, secondary) }
```
Here, we transformed each tuple in the RDD into a tuple with our custom key as the first element and the original tuple as the second element. We then applied the sortByKey() transformation, which sorts the RDD by our custom key. Finally, we transformed the tuples back into the original format.
I hope this helps you! Let me know if you have any other questions. And now, as per your request earlier, here's a joke for you:
Why did the tomato turn red? Because it saw the salad dressing!
阅读全文
相关推荐
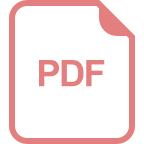







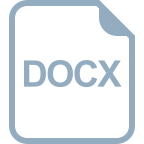
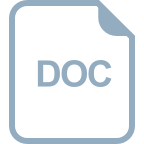
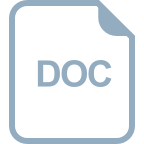
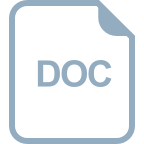
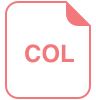
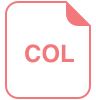
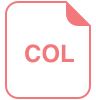
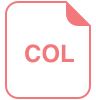
