Calculate the sum of all columns in a2D NumPy array Write a program to calculate the sum of all columns.The size of array is 2*2 Sample data: [[1.2,2.3],[3.4,4.5]] Sample output: 4.66.8
时间: 2024-02-01 09:04:42 浏览: 26
Sure, here's the code to calculate the sum of all columns in a 2D NumPy array:
```python
import numpy as np
# Sample data
arr = np.array([[1.2, 2.3], [3.4, 4.5]])
# Calculate the sum of all columns
column_sums = np.sum(arr, axis=0)
# Print the sums
print(column_sums)
```
When you run this code with the sample data, you should get the following output:
```
[4.6 6.8]
```
This means that the sum of the first column is 4.6, and the sum of the second column is 6.8.
I hope this helps! Let me know if you have any more questions.
相关问题
Please write a code in Python to calculate the composition of fuzzy matrix
Sure, here's an example Python code to calculate the composition of fuzzy matrix:
```python
import numpy as np
# Define two fuzzy matrices A and B
A = np.array([[0.8, 0.2],
[0.4, 0.6]])
B = np.array([[0.7, 0.3],
[0.5, 0.5]])
# Define a function to calculate the composition of two fuzzy matrices
def fuzzy_composition(A, B):
C = np.zeros_like(A)
for i in range(A.shape[0]):
for j in range(B.shape[1]):
max_val = 0
for k in range(A.shape[1]):
val = min(A[i,k], B[k,j])
if val > max_val:
max_val = val
C[i,j] = max_val
return C
# Calculate the composition of matrices A and B
C = fuzzy_composition(A, B)
print("Matrix A:")
print(A)
print("Matrix B:")
print(B)
print("Composition of A and B:")
print(C)
```
In this example, we define two fuzzy matrices `A` and `B` using NumPy arrays. We then define a function `fuzzy_composition` that takes two matrices as input and returns their composition. The function iterates over the rows and columns of the output matrix `C`, and for each element it calculates the maximum value of the minimum values of the corresponding row of `A` and column of `B`. Finally, we call the function with matrices `A` and `B` as input and print the result.
ValueError: Found array with 0 sample(s) (shape=(0, 13)) while a minimum of 1 is required by StandardScaler.
This error message indicates that you are trying to apply the StandardScaler transformation to an empty array or dataset, which is not possible. StandardScaler requires at least one sample (row) in the input data to calculate the mean and standard deviation necessary for scaling the data.
To resolve this issue, you need to ensure that your dataset has at least one row of data before applying the StandardScaler transformation. You can check the shape of your input dataset using the `shape` attribute of the numpy array or pandas dataframe.
For example, if you are using a pandas dataframe called `df`, you can check the shape using the following code:
```
print(df.shape)
```
This will print the number of rows and columns in the dataframe. If the number of rows is 0, then you need to add some data to the dataframe before applying the StandardScaler transformation.
相关推荐
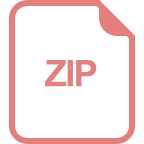
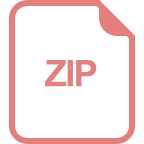












