请提供使用torch.nn.functional中的函数来实现RBF的程序示例
时间: 2024-03-17 11:44:38 浏览: 22
当使用`torch.nn.RBF`不可用时,你可以使用`torch.nn.functional`中的`torch.exp`和`torch.norm`函数来实现RBF。下面是一个简单的示例代码:
```python
import torch
def rbf(x, c, gamma):
return torch.exp(-gamma * torch.norm(x-c, dim=-1))
x = torch.randn(10, 5)
c = torch.randn(3, 5)
gamma = 0.1
out = rbf(x, c, gamma)
print(out)
```
在上面的代码中,`x`是输入数据,`c`是中心向量,`gamma`是RBF函数的一个超参数。`torch.norm`函数用来计算输入数据和中心向量之间的欧几里得距离。`torch.exp`用来计算指数。
相关问题
torch.nn.functional 的relu函数
torch.nn.functional中的relu函数是一个常用的激活函数,将输入的负值置为零,保持非负值不变。具体来说,relu函数的定义如下:
```
relu(input, inplace=False) -> Tensor
```
其中,input是一个张量,表示输入的数据。inplace是一个布尔值,表示是否进行原地操作,默认为False。
relu函数的计算公式如下:
```
relu(x) = max(0, x)
```
即,对于输入x,如果x大于等于零,则输出为x;如果x小于零,则输出为零。
使用relu函数可以有效地引入非线性特性,使神经网络能够更好地拟合非线性数据。在深度学习中,relu函数常被用作隐藏层的激活函数。
pytroch中如何使用torch.nn.functional.accuracy
您可以使用torch.nn.functional.accuracy函数来计算分类问题的准确率。该函数需要两个参数:模型的输出和真实标签。您可以使用torch.max函数来获取模型输出的预测标签,然后将其与真实标签进行比较,最后计算准确率。以下是使用torch.nn.functional.accuracy函数的示例代码:
```
import torch
import torch.nn.functional as F
# 模型输出
outputs = torch.randn(10, 5)
# 真实标签
targets = torch.randint(5, (10,))
# 获取预测标签
preds = torch.argmax(outputs, dim=1)
# 计算准确率
acc = F.accuracy(preds, targets)
print(acc)
```
输出结果将是一个到1之间的浮点数,表示模型的准确率。
相关推荐
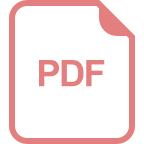
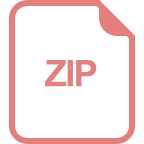
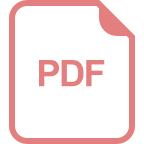












