YOLOv5算法流程伪代码
时间: 2023-06-20 13:07:31 浏览: 560
以下是YOLOv5算法的伪代码流程:
```
1. 加载预训练模型或者随机初始化网络参数
2. 对输入图像进行缩放和归一化处理
3. 将图像输入到神经网络,得到输出特征图
4. 利用特征图对每个网格单元进行预测
a. 预测每个网格单元是否包含物体
b. 预测每个网格单元中物体的类别(使用softmax激活函数)
c. 预测每个网格单元中物体的位置和大小(使用sigmoid激活函数)
5. 对预测得到的边界框进行筛选和筛除
a. 去除置信度低于阈值的边界框
b. 使用非极大值抑制算法去除重叠的边界框
6. 输出最终的预测结果,包括每个物体的类别、置信度和位置信息
```
相关问题
用伪代码描述YOLOv5算法流程
以下是YOLOv5算法的简化流程伪代码:
```
# 加载模型
model = load_model("yolov5")
# 输入图像
image = load_image("input_image.jpg")
# 预处理图像
processed_image = preprocess(image)
# 进行目标检测
detections = model.detect(processed_image)
# 处理检测结果
for detection in detections:
# 解析检测结果
class_id, confidence, x, y, w, h = parse_detection(detection)
# 绘制检测框和类别标签
draw_detection(image, class_id, confidence, x, y, w, h)
# 输出图像
save_image(image, "output_image.jpg")
```
其中,`load_model`函数用于加载YOLOv5模型,`load_image`函数用于加载输入图像,`preprocess`函数用于预处理图像,`detect`函数用于进行目标检测,`parse_detection`函数用于解析检测结果,`draw_detection`函数用于绘制检测框和类别标签,`save_image`函数用于保存输出图像。
用伪代码描述yolov5s算法流程
以下是 YOLOv5s 算法的伪代码描述:
1. 加载预训练模型
model = load_pretrained_model('yolov5s.weights')
2. 对输入图像进行预处理
image = preprocess_image('input_image.jpg')
3. 将图像输入模型中进行前向传播
outputs = model.forward(image)
4. 对模型输出进行后处理
detections = postprocess_outputs(outputs)
5. 对检测结果进行筛选和处理
filtered_detections = filter_detections(detections)
6. 输出最终的检测结果
output_results(filtered_detections)
其中,预处理和后处理的具体实现方式可以根据具体的实现细节进行调整。而筛选和处理的具体方式则包括了非极大值抑制(NMS)、类别置信度阈值等操作。
相关推荐
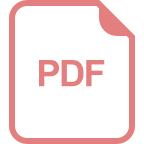
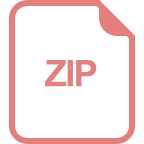
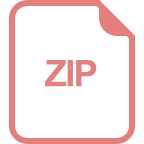












