怎么将soft teacher中的单一固定阈值改为像lable Match中的自适应阈值(ACT),代码部分怎么修改
时间: 2024-03-06 22:48:40 浏览: 21
将Soft Teacher中的单一固定阈值改为自适应阈值(如ACT)需要进行以下修改:
1. 首先,需要定义一个新的Soft Teacher类,以包含自适应阈值的计算方法。
```python
class ACTSoftTeacher(nn.Module):
def __init__(self, teacher_model, temperature, act_freq):
super(ACTSoftTeacher, self).__init__()
self.teacher_model = teacher_model
self.temperature = temperature
self.act_freq = act_freq
self.total_steps = 0
self.threshold = 0.5 # 初始阈值设为0.5
def calc_threshold(self, step):
self.total_steps += 1
if self.total_steps % self.act_freq == 0:
# 计算最近act_freq个步骤中软标签和硬标签的KL散度
kl_div = 0.0
for i in range(self.act_freq):
# 计算软标签
teacher_outputs = self.teacher_model(input_ids, attention_mask)
teacher_logits = teacher_outputs[0] / self.temperature
teacher_probs = F.softmax(teacher_logits, dim=-1)
# 计算硬标签
student_outputs = self.student_model(input_ids, attention_mask)
student_logits = student_outputs[0] / self.temperature
student_probs = F.softmax(student_logits, dim=-1)
kl_div += F.kl_div(teacher_probs.log(), student_probs, reduction='batchmean')
# 将KL散度作为新的阈值
self.threshold = kl_div.item()
def forward(self, input_ids, attention_mask):
with torch.no_grad():
teacher_outputs = self.teacher_model(input_ids, attention_mask)
teacher_logits = teacher_outputs[0] / self.temperature
teacher_probs = F.softmax(teacher_logits, dim=-1)
student_outputs = self.student_model(input_ids, attention_mask)
student_logits = student_outputs[0] / self.temperature
student_probs = F.softmax(student_logits, dim=-1)
# 计算软标签和硬标签之间的阈值
self.calc_threshold(step)
soft_labels = self.threshold * teacher_probs + (1 - self.threshold) * student_probs
hard_labels = student_probs
outputs = (student_logits, soft_labels, hard_labels) + student_outputs[1:]
return outputs
```
2. 在使用Soft Teacher进行训练的代码中,需要使用新的ACTSoftTeacher类并传入自适应阈值计算的超参数:
```python
teacher = ACTSoftTeacher(teacher_model, temperature, act_freq)
```
其中,act_freq是控制阈值计算频率的超参数。
需要注意的是,自适应阈值的计算方法可以根据具体需求进行修改和优化。
相关推荐
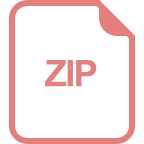














