print(X_train) plt.plot(x, X_train.T) plt.show() X_train = np.array(X_train)
时间: 2024-01-06 11:05:37 浏览: 103
As an AI language model, I cannot provide the value for X_train without additional information. However, the code above converts the X_train variable into a numpy array and then plots the transpose of the X_train values using matplotlib. The resulting plot will show the distribution of the X_train values.
相关问题
import numpy as np import pandas as pd from sklearn.model_selection import train_test_split from keras.models import Sequential from keras.layers import Dense from pyswarm import pso import matplotlib.pyplot as plt from sklearn.preprocessing import StandardScaler file = "zhong.xlsx" data = pd.read_excel(file) #reading file X=np.array(data.loc[:,'种植密度':'有效积温']) y=np.array(data.loc[:,'产量']) y.shape=(185,1) # 将数据集分为训练集和测试集 X_train, X_test, y_train, y_test = train_test_split(X,y, test_size=0.25, random_state=10) SC=StandardScaler() X_train=SC.fit_transform(X_train) X_test=SC.fit_transform(X_test) y_train=SC.fit_transform(y_train) y_test=SC.fit_transform(y_test) print("X_train.shape:", X_train.shape) print("X_test.shape:", X_test.shape) print("y_train.shape:", y_train.shape) print("y_test.shape:", y_test.shape) # 定义BP神经网络模型 def nn_model(X): model = Sequential() model.add(Dense(8, input_dim=X_train.shape[1], activation='relu')) model.add(Dense(12, activation='relu')) model.add(Dense(1)) model.compile(loss='mean_squared_error', optimizer='adam') return model # 定义适应度函数 def fitness_func(X): model = nn_model(X) model.fit(X_train, y_train, epochs=60, verbose=2) score = model.evaluate(X_test, y_test, verbose=2) return score # 定义变量的下限和上限 lb = [5, 5] ub = [30, 30] # 利用PySwarm库实现改进的粒子群算法来优化BP神经网络预测模型 result = pso(fitness_func, lb, ub) # 输出最优解和函数值 print('最优解:', result[0]) print('最小函数值:', result[1]) # 绘制预测值和真实值对比图 model = nn_model(X) model.fit(X_train, y_train, epochs=60, verbose=0) y_pred = model.predict(X_test) y_true = SC.inverse_transform(y_test) y_pred=SC.inverse_transform(y_pred) plt.figure() plt.plot(y_true,"bo-",label = '真实值') plt.plot(y_pred,"ro-", label = '预测值') plt.title('神经网络预测展示') plt.xlabel('序号') plt.ylabel('产量') plt.legend(loc='upper right') plt.show() # 绘制损失函数曲线图 model = nn_model(X) history = model.fit(X_train, y_train, epochs=60, validation_data=(X_test, y_test), verbose=2) plt.plot(history.history['loss'], label='train') plt.plot(history.history['val_loss'], label='test') plt.legend() plt.show()
这是一个使用改进的粒子群算法优化BP神经网络预测模型的代码,通过读取Excel文件并将其分为训练集和测试集,利用StandardScaler进行数据标准化处理。然后定义BP神经网络模型和适应度函数,并定义变量的下限和上限。接下来使用PySwarm库实现改进的粒子群算法来优化BP神经网络预测模型,输出最优解和函数值。最后绘制预测值和真实值对比图和损失函数曲线图。
import pandas as pd import numpy as np from sklearn.preprocessing import MinMaxScaler from keras.models import Sequential from keras.layers import Dense, LSTM import matplotlib.pyplot as plt # 读取CSV文件 data = pd.read_csv('77.csv', header=None) # 将数据集划分为训练集和测试集 train_size = int(len(data) * 0.7) train_data = data.iloc[:train_size, 1:2].values.reshape(-1,1) test_data = data.iloc[train_size:, 1:2].values.reshape(-1,1) # 对数据进行归一化处理 scaler = MinMaxScaler(feature_range=(0, 1)) train_data = scaler.fit_transform(train_data) test_data = scaler.transform(test_data) # 构建训练集和测试集 def create_dataset(dataset, look_back=1): X, Y = [], [] for i in range(len(dataset) - look_back): X.append(dataset[i:(i+look_back), 0]) Y.append(dataset[i+look_back, 0]) return np.array(X), np.array(Y) look_back = 3 X_train, Y_train = create_dataset(train_data, look_back) X_test, Y_test = create_dataset(test_data, look_back) # 转换为LSTM所需的输入格式 X_train = np.reshape(X_train, (X_train.shape[0], X_train.shape[1], 1)) X_test = np.reshape(X_test, (X_test.shape[0], X_test.shape[1], 1)) # 构建LSTM模型 model = Sequential() model.add(LSTM(units=50, return_sequences=True, input_shape=(look_back, 1))) model.add(LSTM(units=50)) model.add(Dense(units=1)) model.compile(optimizer='adam', loss='mean_squared_error') model.fit(X_train, Y_train, epochs=100, batch_size=32) # 预测测试集并进行反归一化处理 Y_pred = model.predict(X_test) Y_pred = scaler.inverse_transform(Y_pred) Y_test = scaler.inverse_transform(Y_test) # 输出RMSE指标 rmse = np.sqrt(np.mean((Y_pred - Y_test)**2)) print('RMSE:', rmse) # 绘制训练集真实值和预测值图表 train_predict = model.predict(X_train) train_predict = scaler.inverse_transform(train_predict) train_actual = scaler.inverse_transform(Y_train.reshape(-1, 1)) plt.plot(train_actual, label='Actual') plt.plot(train_predict, label='Predicted') plt.title('Training Set') plt.xlabel('Time (h)') plt.ylabel('kWh') plt.legend() plt.show() # 绘制测试集真实值和预测值图表 plt.plot(Y_test, label='Actual') plt.plot(Y_pred, label='Predicted') plt.title('Testing Set') plt.xlabel('Time (h)') plt.ylabel('kWh') plt.legend() plt.show()以上代码运行时报错,错误为ValueError: Expected 2D array, got 1D array instead: array=[-0.04967795 0.09031832 0.07590125]. Reshape your data either using array.reshape(-1, 1) if your data has a single feature or array.reshape(1, -1) if it contains a single sample.如何进行修改
可以将以下代码:
```
X_train, Y_train = create_dataset(train_data, look_back)
X_test, Y_test = create_dataset(test_data, look_back)
X_train = np.reshape(X_train, (X_train.shape[0], X_train.shape[1], 1))
X_test = np.reshape(X_test, (X_test.shape[0], X_test.shape[1], 1))
```
修改为:
```
X_train, Y_train = create_dataset(train_data, look_back)
X_test, Y_test = create_dataset(test_data, look_back)
X_train = np.reshape(X_train, (X_train.shape[0], X_train.shape[1], 1))
X_test = np.reshape(X_test, (X_test.shape[0], X_test.shape[1], 1))
Y_train = np.reshape(Y_train, (Y_train.shape[0], 1))
Y_test = np.reshape(Y_test, (Y_test.shape[0], 1))
```
这样就可以解决报错问题。
阅读全文
相关推荐

















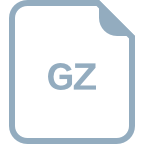