metropolis algorithm
时间: 2023-04-29 17:06:33 浏览: 95
Metropolis算法是一种 Monte Carlo (蒙特卡罗) 模拟的算法,用于计算复杂系统的性质。它是由Nicholas Metropolis等人于1953年开发的,可以用于计算温度、能量、热动力学性质等。 这种算法的核心思想是使用一些随机变化来模拟系统的状态,将变化的概率与系统的能量和温度之间的比较进行计算,然后根据这个比较,接受或拒绝变化,进而导致系统在不同状态之间进行转移。该算法在许多领域都有广泛的应用,例如统计力学、计算物理学、化学等。
相关问题
Use the Metropolis-Hastings algorithm to generate samples from the beta distribution. Try using the uniform distribution as a candidate distribution. Note that you can simplify by canceling con-stants.
Sure, I can help you with that.
The Metropolis-Hastings algorithm is a Markov Chain Monte Carlo (MCMC) method used to generate samples from a target distribution that is difficult to sample from directly. In this case, we want to generate samples from the beta distribution using the uniform distribution as a candidate distribution. The beta distribution is given by:
$$p(x) \propto x^{\alpha-1}(1-x)^{\beta-1}$$
where $x \in [0, 1]$ and $\alpha, \beta > 0$ are the shape parameters.
To apply the Metropolis-Hastings algorithm, we need to follow these steps:
1. Initialize $x_0$ to some value in $[0, 1]$.
2. For each iteration $t$:
- Generate a candidate sample $y$ from the uniform distribution $U(x_t - \epsilon, x_t + \epsilon)$, where $\epsilon$ is a small positive constant.
- Compute the acceptance ratio $r = \frac{p(y)}{p(x_t)}$.
- Generate a random number $u$ from the uniform distribution $U(0, 1)$.
- If $u < r$, set $x_{t+1} = y$, otherwise set $x_{t+1} = x_t$.
3. Repeat step 2 until enough samples have been generated.
Note that we can simplify the acceptance ratio by canceling constants:
$$r = \frac{p(y)}{p(x_t)} = \frac{y^{\alpha-1}(1-y)^{\beta-1}}{x_t^{\alpha-1}(1-x_t)^{\beta-1}}$$
Here's some Python code that implements the Metropolis-Hastings algorithm to generate samples from the beta distribution:
```python
import numpy as np
def beta_mh(alpha, beta, epsilon, n_samples):
# Initialize
x = np.random.rand()
samples = np.zeros(n_samples)
# Generate samples
for i in range(n_samples):
# Generate candidate sample
y = np.random.uniform(x - epsilon, x + epsilon)
# Compute acceptance ratio
r = (y**(alpha-1) * (1-y)**(beta-1)) / (x**(alpha-1) * (1-x)**(beta-1))
# Generate random number
u = np.random.rand()
# Accept or reject candidate sample
if u < r:
x = y
# Save sample
samples[i] = x
return samples
```
You can call this function with the desired shape parameters $\alpha$ and $\beta$, the step size $\epsilon$, and the number of samples to generate. For example:
```python
alpha = 2
beta = 5
epsilon = 0.1
n_samples = 1000
samples = beta_mh(alpha, beta, epsilon, n_samples)
```
This will generate 1000 samples from the beta distribution with shape parameters $\alpha=2$ and $\beta=5$, using the uniform distribution with step size 0.1 as the candidate distribution.
metropolis hastings
Metropolis Hastings is a Markov chain Monte Carlo (MCMC) algorithm used to generate samples from a target probability distribution that is difficult to sample directly. The algorithm is named after its inventors, Nicholas Metropolis and Nicholas Hastings.
The Metropolis Hastings algorithm works by proposing a new state in the Markov chain from the current state, using a proposal distribution. The acceptance probability of the proposed state is then calculated based on the target distribution and the proposal distribution. If the proposed state is accepted, it becomes the new current state in the chain, otherwise the current state remains unchanged.
The algorithm iterates through this process, generating a sequence of states in the Markov chain that eventually converge to the desired target distribution. The convergence of the algorithm depends on the proposal distribution and the acceptance probability function.
The Metropolis Hastings algorithm is widely used in Bayesian statistics, where it is used to generate samples from the posterior distribution of a model. It is also used in physics and other fields where simulation of complex systems is needed.
阅读全文
相关推荐
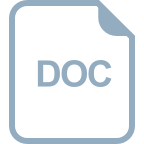
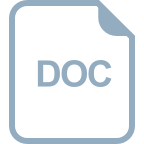
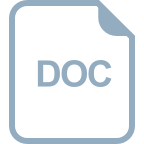
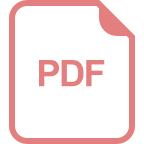
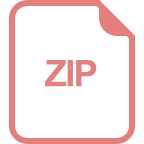
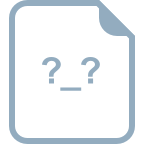
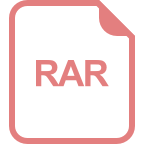
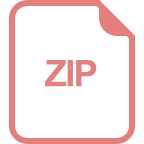
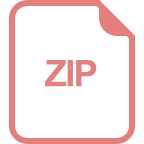
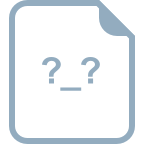
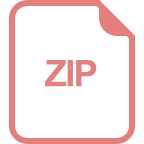
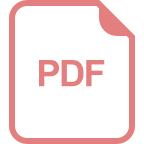
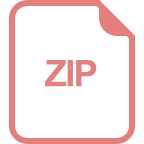
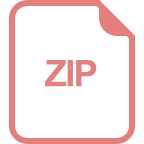
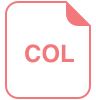

