data.loc[(data['Outcome'] == 0 ) & (data['Insulin'].isnull()), 'Insulin'] = 102.5 data.loc[(data['Outcome'] == 1 ) & (data['Insulin'].isnull()), 'Insulin'] = 169.5
时间: 2023-10-18 14:04:16 浏览: 27
这是一个 Python 代码片段,用于对数据集中的 Insulin 变量进行缺失值填补。具体来说,代码将 Insulin 变量缺失的行按照分类变量 Outcome 的取值进行了填补。
在第一行中,代码使用 loc 函数选取了两个条件:data['Outcome'] == 0 和 data['Insulin'].isnull()。该条件表示选取分类变量 Outcome 取值为 0 的行,并且 Insulin 变量的值为缺失。然后,使用 loc[ , 'Insulin'] 选取满足条件的行的 Insulin 变量,并将其赋值为 102.5。
在第二行中,代码使用 loc 函数选取了另外两个条件:data['Outcome'] == 1 和 data['Insulin'].isnull()。该条件表示选取分类变量 Outcome 取值为 1 的行,并且 Insulin 变量的值为缺失。然后,使用 loc[ , 'Insulin'] 选取满足条件的行的 Insulin 变量,并将其赋值为 169.5。
这样,代码就完成了对 Insulin 变量缺失值的填补。填补的方法是根据分类变量 Outcome 的不同取值,分别用不同的数值进行填补。
相关问题
function median_target(var) { temp = data[data[var].notnull()]; temp = temp[[var, 'Outcome']].groupby(['Outcome'])[[var]].median().reset_index(); return temp; } data.loc[(data['Outcome'] == 0) & (data['Insulin'].isnull()), 'Insulin'] = 102.5; data.loc[(data['Outcome'] == 1) & (data['Insulin'].isnull()), 'Insulin'] = 169.5; data.loc[(data['Outcome'] == 0) & (data['Glucose'].isnull()), 'Glucose'] = 107; data.loc[(data['Outcome'] == 1) & (data['Glucose'].isnull()), 'Glucose'] = 1; data.loc[(data['Outcome'] == 0) & (data['SkinThickness'].isnull()), 'SkinThickness'] = 27; data.loc[(data['Outcome'] == 1) & (data['SkinThickness'].isnull()), 'SkinThickness'] = 32; data.loc[(data['Outcome'] == 0) & (data['BloodPressure'].isnull()), 'BloodPressure'] = 70; data.loc[(data['Outcome'] == 1) & (data['BloodPressure'].isnull()), 'BloodPressure'] = 74.5; data.loc[(data['Outcome'] == 0) & (data['BMI'].isnull()), 'BMI'] = 30.1; data.loc[(data['Outcome'] == 1) & (data['BMI'].isnull()), 'BMI'] = 34.3; target_col = ["Outcome"]; cat_cols = data.nunique()[data.nunique() < 12].keys().tolist(); cat_cols = [x for x in cat_cols]; num_cols = [x for x in data.columns if x not in cat_cols + target_col]; bin_cols = data.nunique()[data.nunique() == 2].keys().tolist(); multi_cols = [i for i in cat_cols if i in bin_cols]; le = LabelEncoder(); for i in bin_cols: data[i] = le.fit_transform(data[i]); data = pd.get_dummies(data=data, columns=multi_cols); std = StandardScaler(); scaled = std.fit_transform(data[num_cols]); scaled = pd.DataFrame(scaled, columns=num_cols); df_data_og = data.copy(); data = data.drop(columns=num_cols, axis=1); data = data.merge(scaled, left_index=True, right_index=True, how='left'); X = data.drop('Outcome', axis=1); y = data['Outcome']; X_train, X_test, y_train, y_test = train_test_split(X, y, train_size=0.8, shuffle=True, random_state=1); y_train = to_categorical(y_train); y_test = to_categorical(y_test);将这段代码添加注释
# 导入必要的库
import pandas as pd
from sklearn.preprocessing import LabelEncoder, StandardScaler
from keras.utils import to_categorical
from sklearn.model_selection import train_test_split
# 定义函数,返回每个特征在不同结果下的中位数
def median_target(var):
temp = data[data[var].notnull()]
temp = temp[[var, 'Outcome']].groupby(['Outcome'])[[var]].median().reset_index()
return temp
# 将缺失值填充为中位数
data.loc[(data['Outcome'] == 0) & (data['Insulin'].isnull()), 'Insulin'] = 102.5
data.loc[(data['Outcome'] == 1) & (data['Insulin'].isnull()), 'Insulin'] = 169.5
data.loc[(data['Outcome'] == 0) & (data['Glucose'].isnull()), 'Glucose'] = 107
data.loc[(data['Outcome'] == 1) & (data['Glucose'].isnull()), 'Glucose'] = 1
data.loc[(data['Outcome'] == 0) & (data['SkinThickness'].isnull()), 'SkinThickness'] = 27
data.loc[(data['Outcome'] == 1) & (data['SkinThickness'].isnull()), 'SkinThickness'] = 32
data.loc[(data['Outcome'] == 0) & (data['BloodPressure'].isnull()), 'BloodPressure'] = 70
data.loc[(data['Outcome'] == 1) & (data['BloodPressure'].isnull()), 'BloodPressure'] = 74.5
data.loc[(data['Outcome'] == 0) & (data['BMI'].isnull()), 'BMI'] = 30.1
data.loc[(data['Outcome'] == 1) & (data['BMI'].isnull()), 'BMI'] = 34.3
# 将数据进行分类处理
target_col = ["Outcome"]
cat_cols = data.nunique()[data.nunique() < 12].keys().tolist()
cat_cols = [x for x in cat_cols]
num_cols = [x for x in data.columns if x not in cat_cols + target_col]
bin_cols = data.nunique()[data.nunique() == 2].keys().tolist()
multi_cols = [i for i in cat_cols if i in bin_cols]
# 对二分类特征进行编码
le = LabelEncoder()
for i in bin_cols:
data[i] = le.fit_transform(data[i])
# 将分类特征进行独热编码
data = pd.get_dummies(data=data, columns=multi_cols)
# 对数值特征进行标准化
std = StandardScaler()
scaled = std.fit_transform(data[num_cols])
scaled = pd.DataFrame(scaled, columns=num_cols)
# 将数据进行合并
df_data_og = data.copy()
data = data.drop(columns=num_cols, axis=1)
data = data.merge(scaled, left_index=True, right_index=True, how='left')
# 划分训练集和测试集,对标签进行独热编码
X = data.drop('Outcome', axis=1)
y = data['Outcome']
X_train, X_test, y_train, y_test = train_test_split(X, y, train_size=0.8, shuffle=True, random_state=1)
y_train = to_categorical(y_train)
y_test = to_categorical(y_test)
将下列代码变为伪代码def median_target(var): temp = data[data[var].notnull()] temp = temp[[var, 'Outcome']].groupby(['Outcome'])[[var]].median().reset_index() return temp data.loc[(data['Outcome'] == 0 ) & (data['Insulin'].isnull()), 'Insulin'] = 102.5 data.loc[(data['Result'] == 1 ) & (data['Insulin'].isnull()), 'Insulin'] = 169.5 data.loc[(data['Result'] == 0 ) & (data['Glucose'].isnull()), 'Glucose'] = 107 data.loc[(data['Result'] == 1 ) & (data['Glucose'].isnull()), 'Glucose'] = 1 data.loc[(data['Result'] == 0 ) & (data['SkinThickness'].isnull()), 'SkinThickness'] = 27 data.loc[(data['Result'] == 1 ) & (data['SkinThickness'].isnull()), 'SkinThickness'] = 32 data.loc[(data['Result'] == 0 ) & (data['BloodPressure'].isnull()), 'BloodPressure'] = 70 data.loc[(data['Result'] == 1 ) & (data['BloodPressure'].isnull()), 'BloodPressure'] = 74.5 data.loc[(data['Result'] == 0 ) & (data['BMI'].isnull()), 'BMI'] = 30.1 data.loc[(data['Result'] == 1 ) & (data['BMI'].isnull()), 'BMI'] = 34.3 target_col = [“Outcome”] cat_cols = data.nunique()[data.nunique() < 12].keys().tolist() cat_cols = [x for x in cat_cols ] #numerical列 num_cols = [x for x in data.columns if x 不在 cat_cols + target_col] #Binary列有 2 个值 bin_cols = data.nunique()[data.nunique() == 2].keys().tolist() #Columns 2 个以上的值 multi_cols = [i 表示 i in cat_cols if i in bin_cols] #Label编码二进制列 le = LabelEncoder() for i in bin_cols : data[i] = le.fit_transform(data[i]) #Duplicating列用于多值列 data = pd.get_dummies(data = data,columns = multi_cols ) #Scaling 数字列 std = StandardScaler() 缩放 = std.fit_transform(数据[num_cols]) 缩放 = pd。数据帧(缩放,列=num_cols) #dropping原始值合并数字列的缩放值 df_data_og = 数据.copy() 数据 = 数据.drop(列 = num_cols,轴 = 1) 数据 = 数据.合并(缩放,left_index=真,right_index=真,如何 = “左”) # 定义 X 和 Y X = 数据.drop('结果', 轴=1) y = 数据['结果'] X_train, X_test, y_train, y_test = train_test_split(X, y, train_size=0.8, shuffle=True, random_state=1) y_train = to_categorical(y_train) y_test = to_categorical(y_test)
function median_target(var) {
temp = data[data[var].notnull()];
temp = temp[[var, 'Outcome']].groupby(['Outcome'])[[var]].median().reset_index();
return temp;
}
data.loc[(data['Outcome'] == 0) & (data['Insulin'].isnull()), 'Insulin'] = 102.5;
data.loc[(data['Outcome'] == 1) & (data['Insulin'].isnull()), 'Insulin'] = 169.5;
data.loc[(data['Outcome'] == 0) & (data['Glucose'].isnull()), 'Glucose'] = 107;
data.loc[(data['Outcome'] == 1) & (data['Glucose'].isnull()), 'Glucose'] = 1;
data.loc[(data['Outcome'] == 0) & (data['SkinThickness'].isnull()), 'SkinThickness'] = 27;
data.loc[(data['Outcome'] == 1) & (data['SkinThickness'].isnull()), 'SkinThickness'] = 32;
data.loc[(data['Outcome'] == 0) & (data['BloodPressure'].isnull()), 'BloodPressure'] = 70;
data.loc[(data['Outcome'] == 1) & (data['BloodPressure'].isnull()), 'BloodPressure'] = 74.5;
data.loc[(data['Outcome'] == 0) & (data['BMI'].isnull()), 'BMI'] = 30.1;
data.loc[(data['Outcome'] == 1) & (data['BMI'].isnull()), 'BMI'] = 34.3;
target_col = ["Outcome"];
cat_cols = data.nunique()[data.nunique() < 12].keys().tolist();
cat_cols = [x for x in cat_cols];
num_cols = [x for x in data.columns if x not in cat_cols + target_col];
bin_cols = data.nunique()[data.nunique() == 2].keys().tolist();
multi_cols = [i for i in cat_cols if i in bin_cols];
le = LabelEncoder();
for i in bin_cols:
data[i] = le.fit_transform(data[i]);
data = pd.get_dummies(data=data, columns=multi_cols);
std = StandardScaler();
scaled = std.fit_transform(data[num_cols]);
scaled = pd.DataFrame(scaled, columns=num_cols);
df_data_og = data.copy();
data = data.drop(columns=num_cols, axis=1);
data = data.merge(scaled, left_index=True, right_index=True, how='left');
X = data.drop('Outcome', axis=1);
y = data['Outcome'];
X_train, X_test, y_train, y_test = train_test_split(X, y, train_size=0.8, shuffle=True, random_state=1);
y_train = to_categorical(y_train);
y_test = to_categorical(y_test);
相关推荐
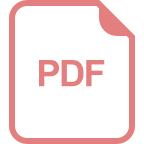
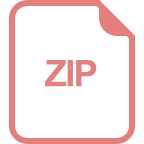













