matlab arima模型
时间: 2023-10-09 07:04:12 浏览: 113
ARIMA(Autoregressive Integrated Moving Average)模型是一种常用的时间序列预测方法,可以用于分析和预测一些具有时间相关性的数据,如股票价格、气温等。MATLAB中提供了ARIMA模型的分析工具箱。
ARIMA模型分为3个部分:自回归模型(AR)、差分模型(I)、移动平均模型(MA)。ARIMA模型的参数可以通过自相关函数(ACF)和偏自相关函数(PACF)确定。
MATLAB中可以使用arima函数创建ARIMA模型对象,然后使用estimate函数对模型进行参数估计,最后使用forecast函数进行预测。例如:
```matlab
% 读入时间序列数据
data = readtable('data.csv');
y = data.Var1;
% 创建ARIMA模型对象
model = arima(2,1,2);
% 估计模型参数
estModel = estimate(model, y);
% 预测未来10个时间点的数据
forecastData = forecast(estModel, 10);
```
其中,arima函数的参数依次为AR阶数、差分阶数、MA阶数。在估计模型参数时,可以使用MLE(最大似然估计)或OLS(最小二乘估计)方法。在预测时,可以指定预测时间点的个数。
相关问题
matlab ARIMA模型
ARIMA模型是一种时间序列模型,它结合了自回归(AR)、差分(I)和移动平均(MA)模型的特点。ARIMA模型可以用来对时间序列数据进行预测和分析。在MATLAB中,可以使用arima函数来建立ARIMA模型,并使用estimate函数来估计模型的参数。
例如,如果我们想建立一个ARIMA(2,1,0)模型,即具有2阶自回归和1阶差分的模型,可以使用以下代码:
AR_Order = 2;
MA_Order = 0;
ARIMA_Model = arima(AR_Order, 1, MA_Order);
然后,可以使用estimate函数来估计模型的参数:
EstMdl = estimate(ARIMA_Model, y);
其中,y是输入的时间序列数据。通过估计参数,我们可以得到一个已经训练好的ARIMA模型,可以用来进行预测和分析。
请注意,上述代码只是一个示例,实际使用ARIMA模型时,需要根据具体的数据和问题来选择合适的模型阶数和参数。
#### 引用[.reference_title]
- *1* *2* *3* [如何MATLAB实现用ARIMA模型输出参数实施预测](https://blog.csdn.net/Will_Zhan/article/details/116425215)[target="_blank" data-report-click={"spm":"1018.2226.3001.9630","extra":{"utm_source":"vip_chatgpt_common_search_pc_result","utm_medium":"distribute.pc_search_result.none-task-cask-2~all~insert_cask~default-1-null.142^v91^insert_down28v1,239^v3^insert_chatgpt"}} ] [.reference_item]
[ .reference_list ]
matlabarima模型代码
MATLAB中ARIMA模型的代码如下所示:
```matlab
% 假设我们有一个时间序列数据y
% 先使用arima模型拟合数据
model = arima('ARLags', 1, 'MALags', 1);
fit = estimate(model, y);
% 拟合之后,我们可以用该模型进行预测
forecast_horizon = 10; % 设置预测的时间段
[forecasts, ~] = forecast(fit, forecast_horizon);
% 可以使用plot函数来显示拟合结果和预测结果
figure
hold on
plot(y, 'b', 'LineWidth', 1.5); % 原始数据
plot((numel(y)+1):(numel(y)+forecast_horizon), forecasts, 'r--', 'LineWidth', 1.5); % 预测结果
hold off
xlabel('时间');
ylabel('数值');
legend('原始数据', '预测结果');
title('ARIMA模型拟合和预测');
% 还可以用该模型进行残差分析
residuals = infer(fit, y);
figure
plot(residuals)
xlabel('时间');
ylabel('残差');
title('ARIMA模型的残差分析');
% 可以根据需要对模型进行参数调整,如选择更合适的Lags等等
```
这段代码基本上完成了ARIMA模型的拟合和预测,同时也可以进行残差分析。需要特别注意的是,这里只是一个基本示例,具体实际应用中可能需要进行更多的参数调整和模型比较。
阅读全文
相关推荐
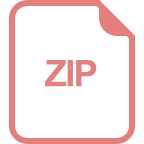
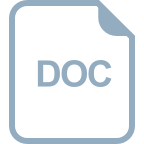






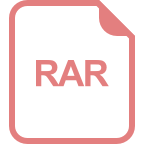
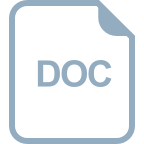
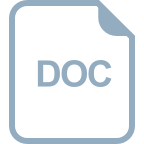
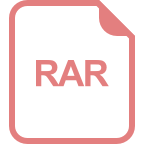
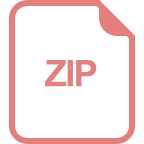
