import torch from transformers import BertTokenizer, BertForQuestionAnswering 加载BERT预训练模型和分词器 model_name = 'bert-base-chinese' # 中文预训练模型 tokenizer = BertTokenizer.from_pretrained(model_name) model = BertForQuestionAnswering.from_pretrained(model_name) 输入文本和问题 context = "我叫river,我在深圳上班。" question = "我是谁?" 对文本和问题进行分词和编码 inputs = tokenizer.encode_plus(question, context, add_special_tokens=True, return_tensors='pt') input_ids = inputs['input_ids'].tolist()[0] token_type_ids = inputs['token_type_ids'].tolist()[0] input_ids=torch.tensor([input_ids],dtype=torch.long) token_type_ids=torch.tensor([token_type_ids],dtype=torch.long) 使用BERT模型进行问答 start_scores, end_scores = model(input_ids=input_ids, token_type_ids=token_type_ids) 为什么strat_scores是str型的而不是tensor型的
时间: 2024-04-19 21:28:10 浏览: 155
根据您提供的代码,`start_scores` 应该是一个张量类型的对象,而不是字符串类型。如果您在打印 `start_scores` 的类型时得到了字符串类型的结果,可能是由于模型加载或处理的问题。
以下是一些可能导致 `start_scores` 类型不正确的原因和解决方法:
1. 检查模型是否成功加载:确保通过 `BertForQuestionAnswering.from_pretrained(model_name)` 成功加载了预训练模型。如果模型加载失败,可能会导致输出结果不正确。
2. 检查模型输入:确保将输入正确传递给模型。您可以使用 `print(input_ids.size(), token_type_ids.size())` 来检查输入张量的形状。确保 `input_ids` 和 `token_type_ids` 的形状符合预期。
3. 检查模型输出:在调用模型后,确保 `start_scores` 的类型是张量。您可以使用 `print(type(start_scores))` 来打印 `start_scores` 的类型。
如果问题仍然存在,请提供完整的错误信息和相关的代码细节,以便我能够更好地帮助您解决问题。
相关问题
import torch from transformers import BertTokenizer, BertForQuestionAnswering # 加载BERT预训练模型和分词器 model_name ='bert-large-uncased-whole-word-masking-finetuned-squad' tokenizer = BertTokenizer.from_pretrained(model_name) model = BertForQuestionAnswering.from_pretrained(model_name) # 输入文本和问题 context = "我叫river,我在深圳上班。" question = "我是谁?" # 对文本和问题进行分词和编码 inputs = tokenizer.encode_plus(question, context, add_special_tokens=True, return_tensors='pt') input_ids = inputs['input_ids'].tolist()[0] token_type_ids = inputs['token_type_ids'].tolist()[0] input_ids = torch.tensor([input_ids], dtype=torch.long) token_type_ids = torch.tensor([token_type_ids], dtype=torch.long) # 使用BERT模型进行问答 start_scores, end_scores = model(input_ids=input_ids, token_type_ids=token_type_ids) print(input_ids.size(), token_type_ids.size()) print(input_ids.dtype) # 获取最佳答案的起始和结束位置 print('startscores is:', type(start_scores), start_scores)
根据您提供的代码,`start_scores` 应该是一个张量类型的对象。在代码中,我注意到您已经打印了 `input_ids.dtype`,并且输出显示为 `torch.int64`,这表明 `input_ids` 是一个整数张量。
根据这个信息,我可以确定 `start_scores` 的类型也应该是张量。请注意,打印张量的类型不应该是字符串形式的,因此如果您的输出结果显示为字符串类型,可能是由于其他地方发生了错误。
为了更好地理解问题,我建议您检查以下几点:
1. 确保在运行代码之前已经正确安装了 `transformers` 库。您可以通过运行 `pip install transformers` 来安装。
2. 确保您的 PyTorch 版本与 transformers 库兼容。建议使用最新版本的 PyTorch 和 transformers。
3. 检查是否存在其他代码或操作会修改 `start_scores` 的类型。
如果问题仍然存在,请提供完整的错误信息和相关的代码细节,以便我能够更好地帮助您解决问题。
import torch from transformers import BertTokenizer, BertForSequenceClassification, AdamW # 加载预训练的BERT模型和tokenizer model_name = 'bert-base-uncased' tokenizer = BertTokenizer.from_pretrained(model_name) model = BertForSequenceClassification.from_pretrained(model_name, num_labels=2) # 假设是一个二分类任务 # 准备数据 df=pd.read_csv('./回复客户快捷短语.csv',encoding='gbk') train_texts=df['x'].to_list() print('打印train_list',train_texts) # 对文本进行tokenize和编码 train_encodings = tokenizer(train_texts, truncation=True, padding=True) print('打印encoding',train_encodings)
这段代码是使用PyTorch和Transformers库加载预训练的BERT模型和tokenizer,并准备数据进行编码。首先,导入必要的库,然后指定要使用的BERT模型的名称('bert-base-uncased')。接下来,使用BertTokenizer.from_pretrained()方法加载预训练的tokenizer,并使用BertForSequenceClassification.from_pretrained()方法加载预训练的BERT模型,同时指定任务的标签数量(在这里假设为二分类任务)。
然后,通过读取CSV文件获取训练文本数据train_texts。使用tokenizer对训练文本进行tokenize和编码,其中truncation参数指定是否截断文本,padding参数指定是否填充文本。
最后,打印train_texts和train_encodings的结果。train_texts是一个包含训练文本的列表,train_encodings是一个包含编码结果的字典。
阅读全文
相关推荐
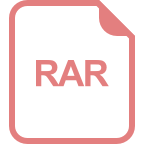
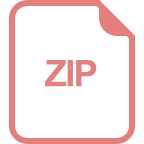












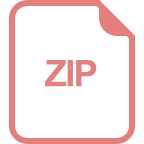