用python写一段遗传算法优化
时间: 2023-02-27 10:05:47 浏览: 55
答案:可以使用Python来编写遗传算法优化的代码,如下:# 遗传算法优化
def geneticAlgorithm(population, fitness_fn, mutation_rate):
for generation in range(num_generations):
# 计算适应度
fitness_values = [fitness_fn(chromosome) for chromosome in population]
# 选择
selected = selection(population, fitness_values)
# 重组
population = crossover(selected)
# 变异
population = mutation(population, mutation_rate)
# 返回最优解
return population[0]
相关问题
用python写一段遗传算法
当然可以!下面是一个简单的用Python编写的遗传算法示例:
```python
import random
# 定义目标函数(示例为简单的函数:f(x) = x^2)
def fitness_function(x):
return x**2
# 初始化种群
def initialize_population(population_size, chromosome_length):
population = []
for _ in range(population_size):
chromosome = [random.randint(0, 1) for _ in range(chromosome_length)]
population.append(chromosome)
return population
# 计算适应度值
def calculate_fitness(population):
fitness_values = []
for chromosome in population:
# 解码染色体
x = int("".join(map(str, chromosome)), 2)
fitness_values.append(fitness_function(x))
return fitness_values
# 选择操作(轮盘赌选择)
def selection(population, fitness_values):
total_fitness = sum(fitness_values)
probabilities = [fitness / total_fitness for fitness in fitness_values]
# 使用轮盘赌选择
selected_population = []
for _ in range(len(population)):
pick = random.uniform(0, 1)
cumulative_probability = 0
for i, probability in enumerate(probabilities):
cumulative_probability += probability
if pick <= cumulative_probability:
selected_population.append(population[i])
break
return selected_population
# 交叉操作(单点交叉)
def crossover(population):
offspring_population = []
for i in range(0, len(population), 2):
parent1 = population[i]
parent2 = population[i+1]
# 随机选择交叉点
crossover_point = random.randint(1, len(parent1)-1)
# 生成子代染色体
offspring1 = parent1[:crossover_point] + parent2[crossover_point:]
offspring2 = parent2[:crossover_point] + parent1[crossover_point:]
offspring_population.append(offspring1)
offspring_population.append(offspring2)
return offspring_population
# 变异操作(随机位变异)
def mutation(population, mutation_rate):
mutated_population = []
for chromosome in population:
mutated_chromosome = []
for gene in chromosome:
if random.random() < mutation_rate:
mutated_chromosome.append(1 - gene) # 变异位点取反
else:
mutated_chromosome.append(gene)
mutated_population.append(mutated_chromosome)
return mutated_population
# 遗传算法主函数
def genetic_algorithm(population_size, chromosome_length, generations, mutation_rate):
population = initialize_population(population_size, chromosome_length)
for _ in range(generations):
fitness_values = calculate_fitness(population)
selected_population = selection(population, fitness_values)
offspring_population = crossover(selected_population)
mutated_population = mutation(offspring_population, mutation_rate)
population = mutated_population
# 返回适应度最高的染色体
fitness_values = calculate_fitness(population)
best_chromosome = population[fitness_values.index(max(fitness_values))]
return best_chromosome
# 执行遗传算法
best_chromosome = genetic_algorithm(population_size=100, chromosome_length=8, generations=100, mutation_rate=0.01)
# 解码最优染色体并输出结果
x = int("".join(map(str, best_chromosome)), 2)
print("最优解 x =", x)
print("最优目标函数值 f(x) =", fitness_function(x))
```
请注意,这只是一个简单的遗传算法示例,可以根据实际需求进行修改和优化。
用Python写一段遗传算法
好的,下面是一个简单的遗传算法的Python代码示例:
```python
import random
# 遗传算法的参数设置
POPULATION_SIZE = 10 # 种群数量
CROSSOVER_PROBABILITY = 0.8 # 交叉概率
MUTATION_PROBABILITY = 0.2 # 变异概率
GENERATIONS = 10 # 迭代次数
# 假设我们要优化的函数为 f(x) = x^2
def objective_function(x):
return x**2
# 初始化种群,每个个体随机生成一个 x 值
def initialize_population():
population = []
for i in range(POPULATION_SIZE):
x = random.uniform(-10, 10)
population.append({'x': x, 'fitness': None})
return population
# 计算个体的适应度
def calculate_fitness(individual):
x = individual['x']
fitness = objective_function(x)
individual['fitness'] = fitness
return fitness
# 选择操作,使用轮盘赌选择算法
def selection(population):
total_fitness = sum([individual['fitness'] for individual in population])
selected_individuals = []
for i in range(POPULATION_SIZE):
pick = random.uniform(0, total_fitness)
current = 0
for individual in population:
current += individual['fitness']
if current > pick:
selected_individuals.append(individual)
break
return selected_individuals
# 交叉操作,使用单点交叉算法
def crossover(parent1, parent2):
if random.random() < CROSSOVER_PROBABILITY:
crossover_point = random.randint(0, 1)
child1 = {'x': None, 'fitness': None}
child2 = {'x': None, 'fitness': None}
child1['x'] = parent1['x'][0:crossover_point] + parent2['x'][crossover_point:]
child2['x'] = parent2['x'][0:crossover_point] + parent1['x'][crossover_point:]
return child1, child2
else:
return parent1, parent2
# 变异操作,使用随机变异算法
def mutation(child):
if random.random() < MUTATION_PROBABILITY:
mutation_point = random.randint(0, len(child['x'])-1)
child['x'][mutation_point] = random.uniform(-10, 10)
return child
# 遗传算法主程序
def genetic_algorithm():
# 初始化种群
population = initialize_population()
# 迭代
for i in range(GENERATIONS):
# 计算个体适应度
for individual in population:
calculate_fitness(individual)
# 选择
selected_individuals = selection(population)
# 交叉
children = []
for i in range(0, POPULATION_SIZE, 2):
child1, child2 = crossover(selected_individuals[i], selected_individuals[i+1])
children.append(mutation(child1))
children.append(mutation(child2))
# 替换原始种群
population = children
# 返回最优解
best_individual = max(population, key=lambda x: x['fitness'])
return best_individual['x']
```
上述代码实现了一个简单的遗传算法,用于求解 $f(x)=x^2$ 函数在 $[-10, 10]$ 区间内的最大值。在遗传算法的迭代过程中,每个个体表示为一个字典,包含一个 x 值和一个适应度值。在初始化种群时,随机生成每个个体的 x 值;在计算适应度时,根据目标函数计算个体的适应度;在选择操作中,使用轮盘赌选择算法选择个体;在交叉操作中,使用单点交叉算法对个体进行交叉;在变异操作中,使用随机变异算法对个体进行变异。最终返回种群中适应度最高的个体的 x 值作为最优解。
相关推荐
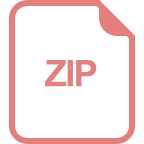
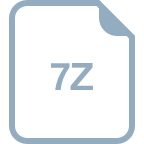
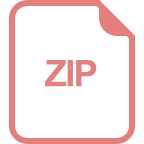












