sequential convex programming
时间: 2023-10-09 17:03:55 浏览: 31
Sequential convex programming (SCP) is an optimization technique used to solve non-convex optimization problems by transforming them into a sequence of convex optimization problems. It is a type of iterative algorithm that starts with an initial feasible solution and then iteratively improves the solution by solving a sequence of convex subproblems.
The basic idea of SCP is to approximate the non-convex objective function with a sequence of convex functions that converge to the original objective function. This is done by dividing the original optimization problem into a sequence of subproblems, each of which is a convex optimization problem. The solution to each subproblem is used as the starting point for the next subproblem, and the process continues until convergence is achieved.
SCP has been successfully applied to a wide range of optimization problems, including machine learning, control, and engineering design. It is particularly useful in problems where the objective function is non-convex, and the constraints are either convex or can be approximated by convex functions.
One of the main advantages of SCP is that it often converges faster than other optimization techniques, such as gradient descent or Newton's method, especially for non-convex problems. Additionally, SCP can handle constraints more effectively than many other optimization techniques.
However, SCP does have some limitations. It can be computationally expensive, particularly when the number of variables is large, and the performance of the algorithm can be sensitive to the choice of initial starting point.
相关推荐
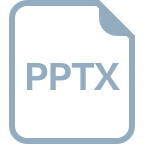
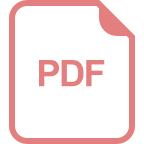





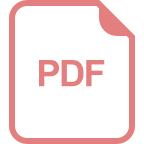
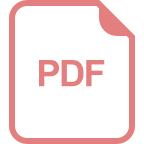
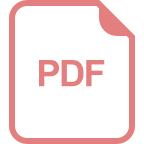
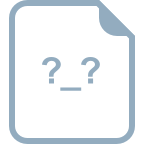
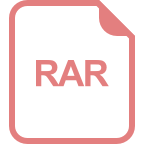
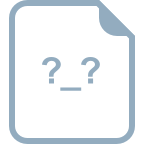
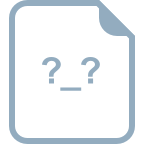