from sklearn.decomposition import LatentDirichletAllocation from sklearn.feature_extraction.text import CountVectorizer # LDA 分析 vectorizer = CountVectorizer() X = vectorizer.fit_transform(data['seg_word']) feature_names = vectorizer.get_feature_names() lda_model = LatentDirichletAllocation(n_components=2, random_state=0) lda_model.fit(vectorizer.fit_transform(data['seg_word']))
时间: 2023-06-17 22:02:52 浏览: 184
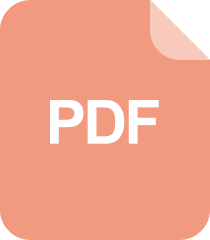
LDA算法分析(中文教程)

这段代码是使用sklearn库中的LatentDirichletAllocation模型进行LDA主题模型分析,其中使用了CountVectorizer将文本转化为词频矩阵。在这个例子中,我们将文本数据data['seg_word']作为输入,经过CountVectorizer处理后,生成了一个词频矩阵X。然后将这个词频矩阵作为输入,使用LatentDirichletAllocation模型进行LDA主题模型分析,其中n_components参数指定了我们希望得到的主题数量。最终,我们可以得到每个主题中每个词的权重,以及每个文本所属的主题。
阅读全文
相关推荐
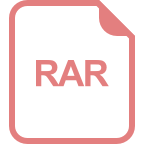

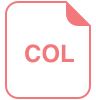
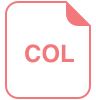
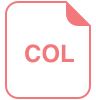
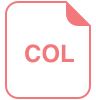
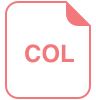
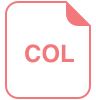
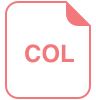








