pytorch的损失函数loss function接口介绍
时间: 2023-09-02 10:02:30 浏览: 138
PyTorch是一个开源的Python机器学习库,提供了丰富的损失函数接口,用于训练神经网络模型。损失函数是用于衡量模型预测结果与真实值之间的差异的函数。
在PyTorch中,常用的损失函数接口包括:
1. nn.L1Loss:计算预测值与真实值之间的绝对值差的平均值,也称为平均绝对值误差(MAE)损失函数。
2. nn.MSELoss:计算预测值与真实值之间的平方差的平均值,也称为均方误差(MSE)损失函数。
3. nn.CrossEntropyLoss:适用于多分类问题的交叉熵损失函数,结合了softmax激活函数和负对数似然损失函数。
4. nn.NLLLoss:适用于多分类问题的负对数似然损失函数,需要与log_softmax激活函数一起使用。
5. nn.BCELoss:适用于二分类问题的二元交叉熵损失函数,对于每个样本,计算预测值与真实值之间的交叉熵。
这些损失函数接口都可以通过创建对应的损失函数对象来使用。使用时,需要将模型的预测值和真实值作为参数传入,计算出模型的损失值。然后可以通过反向传播和优化器来更新模型的参数,以减小损失值。
总而言之,PyTorch提供了丰富的损失函数接口,可以根据不同的任务选择合适的损失函数,帮助训练神经网络模型。
相关问题
pytorch代码实现AdaReg Loss损失函数并用于ConvNeXt v2模型中
AdaReg Loss是一种用于标签平衡的损失函数,其主要作用是解决类别分布不均匀的问题。在这里,我们以ConvNeXt v2模型为例,介绍如何在PyTorch中实现AdaReg Loss。
首先,我们需要定义AdaReg Loss的公式:
$$
\text{AdaRegLoss} = \frac{1}{N}\sum_{i=1}^{N}\left(\alpha\cdot\frac{\text{CELoss}(x_i, y_i)}{1 + \exp(-\beta\cdot(p_i - \gamma))} + (1 - \alpha)\cdot\frac{\text{FocalLoss}(x_i, y_i)}{1 + \exp(\beta\cdot(p_i - \gamma))}\right)
$$
其中,CELoss表示交叉熵损失函数,FocalLoss表示Focal Loss函数,$x_i$表示模型的输出,$y_i$表示真实标签,$p_i$表示模型对样本$i$属于正类的预测概率,$\alpha$表示交叉熵损失函数的权重,$\beta$和$\gamma$是两个超参数,用于调整AdaReg Loss的形状。
接着,我们可以按照以下步骤实现AdaReg Loss:
1. 从PyTorch中导入所需的库和模块:
```python
import torch
import torch.nn as nn
import torch.nn.functional as F
```
2. 定义AdaReg Loss类,并继承自nn.Module:
```python
class AdaRegLoss(nn.Module):
def __init__(self, alpha=0.5, beta=4, gamma=0.5, reduction='mean'):
super(AdaRegLoss, self).__init__()
self.alpha = alpha
self.beta = beta
self.gamma = gamma
self.reduction = reduction
self.ce_loss = nn.CrossEntropyLoss(reduction='none')
self.focal_loss = FocalLoss(reduction='none')
```
在这里,我们定义了三个超参数alpha、beta和gamma,以及一个reduction参数,用于指定如何对batch中的损失进行平均。我们还定义了两个损失函数:交叉熵损失函数和Focal Loss函数。
3. 实现AdaReg Loss的前向传播函数:
```python
def forward(self, inputs, targets):
ce_loss = self.ce_loss(inputs, targets)
pt = torch.exp(-ce_loss)
focal_loss = self.focal_loss(inputs, targets)
alpha_t = self.alpha * pt / (1 + torch.exp(-self.beta * (pt - self.gamma)))
loss = alpha_t * ce_loss + (1 - alpha_t) * focal_loss
if self.reduction == 'mean':
loss = torch.mean(loss)
elif self.reduction == 'sum':
loss = torch.sum(loss)
return loss
```
在这里,我们首先计算交叉熵损失函数和Focal Loss函数。然后,我们计算每个样本的权重alpha_t,并将其应用于交叉熵损失函数和Focal Loss函数。最后,我们根据reduction参数将batch中的损失进行平均或求和。
4. 将AdaReg Loss应用于ConvNeXt v2模型中:
```python
class ConvNeXtV2(nn.Module):
def __init__(self, num_classes=10):
super(ConvNeXtV2, self).__init__()
# define the model architecture
...
# define the loss function
self.loss_fn = AdaRegLoss(alpha=0.5, beta=4, gamma=0.5, reduction='mean')
def forward(self, x, targets=None):
# define the forward pass
...
if targets is not None:
loss = self.loss_fn(output, targets)
return output, loss
else:
return output
```
在这里,我们定义了一个ConvNeXt v2模型,并将AdaReg Loss作为其损失函数。在模型的前向传播函数中,如果传入了真实标签,我们就计算AdaReg Loss,并返回输出和损失;否则,我们只返回输出。
最后,我们可以使用类似以下的代码来训练ConvNeXt v2模型,并使用AdaReg Loss作为其损失函数:
```python
model = ConvNeXtV2(num_classes=10)
optimizer = torch.optim.Adam(model.parameters(), lr=1e-3)
for epoch in range(num_epochs):
for images, labels in train_loader:
images = images.to(device)
labels = labels.to(device)
optimizer.zero_grad()
output, loss = model(images, labels)
loss.backward()
optimizer.step()
```
VAE损失函数编写代码,pytorch
在PyTorch中,Variational Autoencoder (VAE) 的损失函数通常包括两部分:重构误差(reconstruction loss)和潜在变量分布的Kullback-Leibler散度(KL divergence)。下面是一个简单的VANELoss类的示例代码:
```python
import torch
from torch.distributions import Normal
class VAELoss(torch.nn.Module):
def __init__(self, recon_loss_function=torch.nn.MSELoss(), kl_div_weight=1.0):
super(VAELoss, self).__init__()
self.recon_loss = recon_loss_function
self.kl_div_weight = kl_div_weight
self.normal = Normal(loc=torch.zeros(1), scale=torch.ones(1))
def forward(self, x, mu, logvar):
# Reconstruction Loss
reconstruction_error = self.recon_loss(x, mu)
# KL Divergence between Prior and Posterior Distribution
kl_div = -0.5 * torch.sum(1 + logvar - mu.pow(2) - logvar.exp())
# Combine losses with weights
vaeloss = reconstruction_error + self.kl_div_weight * kl_div
return vaeloss, reconstruction_error, kl_div
```
在这个例子中,`x` 是输入数据,`mu` 和 `logvar` 分别是编码后的均值和方差。`recon_loss_function` 可以为MSELoss或其他合适的损失函数。`kl_div_weight` 设置了KL散度的权重,你可以根据需求调整这个值。
阅读全文
相关推荐
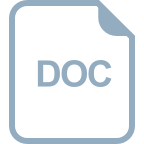
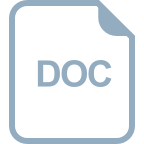
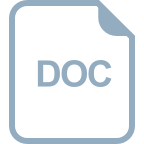
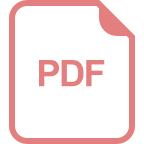
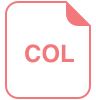
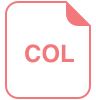
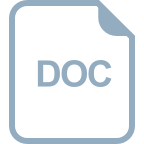
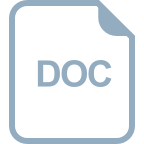
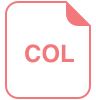
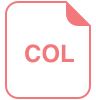
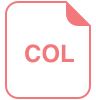
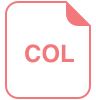




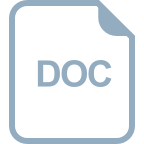