select workplace as name, count(1) as value from( select regexp_replace(b.workplace, '厦门市', '') as workplace from job lateral view explode(split(workplace, '、|,')) b AS workplace ) as a where workplace rlike '湖里|海沧|思明|集美|同安|翔安' group by workplace;
时间: 2023-12-23 11:05:03 浏览: 91
好的,我已经将你提供的HQL语句转换成了MySQL的SQL语句,如下所示:
```
SELECT regexp_replace(b.workplace, '厦门市', '') AS name, COUNT(1) AS value
FROM job
LATERAL VIEW explode(split(workplace, '、|,')) b AS workplace
WHERE workplace REGEXP '湖里|海沧|思明|集美|同安|翔安'
GROUP BY name;
```
请注意,这里使用了MySQL中的REGEXP函数,其作用是匹配正则表达式,用来替代HQL中的rlike关键字。
相关问题
IplImage *TheImage; // TheImage=cvLoadImage(dlg->value_pic_path,-1); TheImage=cvLoadImage("E:\\WD_FILE\\ISE_workplace\\0306\\PIC\\image_pga_output_16k_4k.tif",-1); dlg->DrawPicToHDC(TheImage,IDC_STATIC_PIC); cvReleaseImage(&TheImage); return 0;
这段代码用于加载图像并将绘制到名为 `IDC_STATIC_PIC` 的静态控件上。
首先,声明了一个 `IplImage` 指针 `TheImage`。
然后,使用 `cvLoadImage()` 函数加载图像。注释掉的那行是通过 `dlg->value_pic_path` 变量来指定图像路径,而当前使用的是硬编码的路径。
接下来,调用 `dlg->DrawPicToHDC(TheImage, IDC_STATIC_PIC)` 将加载的图像传递给 `DrawPicToHDC` 函数,并指定要显示图像的静态控件的标识符 `IDC_STATIC_PIC`。
最后,使用 `cvReleaseImage(&TheImage)` 释放图像资源。
函数返回 0 表示执行成功。
优化代码import json import requests from lxml import etree import pandas as pd import time #url='https://www.ptpress.com.cn/masterpiece/getMasterpieceListForPortal' #https://www.ptpress.com.cn/recommendBook/getRecommendTupeListForPortal #http://www.ptpress.com.cn/masterpiece/getMasterpieceListForPortal url='https://www.5iai.com/api/enterprise/job/public/es?pageSize=10&pageNumber=1'#目标数据文件的url #url = ' https://www.5iai.com/api/enterprise/job/public/es?pageSize=10&pageNumber=2&willNature=&function=&wageList=%255B%255D&workplace=&keyword=' for i in range (1,20): response=requests.get(url) aa=response.text print(type(aa)) json_text=json.loads(aa) print(type(json_text)) json_text.keys() bb=json_text['data'] print(type(bb)) print(bb.keys()) cc= bb['content'] print(type(cc)) ee0=cc[0] #是第一条信息 ee1=cc[1] len(cc)#是cc的第一个招聘的第一页招聘信息,是列表类型 print(type(ee0)) ee0.keys() ee1.keys()#0和1的的字典的键都是一样的 data=pd.DataFrame(cc) data.columns ff=data['enterpriseAddress'] print(type(ff)) gg0=ff[0] gg1=ff[1] hh0=gg0['detailedAddress'] hh1 = gg1['detailedAddress'] hh3= ff[2]['detailedAddress'] hh4= ff[3]['detailedAddress'] hh5=data['enterpriseAddress'] [4]['detailedAddress'] data['工作地址']=ff.apply(lambda x:x['detailedAddress']) data['工作地址']=data['enterpriseAddress'].apply(lambda x:x['detailedAddress']) data['工作城市']=data['enterpriseAddress'].apply(lambda x:x['cityCode']) kk=data['enterpriseExtInfo'] print(type(kk)) ll0=kk[0] data['公司名称']=data['enterpriseExtInfo'].apply(lambda x:x['shortName']) data['行业']=data['enterpriseExtInfo'].apply(lambda x:x['industry']) data['人员规模']=data['enterpriseExtInfo'].apply(lambda x:x['personScope']) data['企业性质']=data['enterpriseExtInfo'].apply(lambda x:x['econKind']) data.keys() colNames=['positionName','minimumWage','maximumWage','exp','educationalRequirements','工作地址','工作城市','公司名称','行业','人员规模','企业性质'] data_drop=data[colNames] #https: // www.5iai.com / api / enterprise / job / public?id = 1657941238357299201 #https: // www.5iai.com / api / enterprise / job / public?id = 1657941238353104896 data['id'] #print(type(data['id'])) #suburl='https://www.5iai.com/api/enterprise/job/public?id=1657941238353104896' jobDes = [] for idd in data['id']: suburl = 'https://www.5iai.com/api/enterprise/job/public?id={}'.format(idd) sub_response=requests.get(suburl) subb=sub_response.text #print(type(subb)) sub_text=json.loads(subb) #print(type(sub_text)) sub_text.keys subaa=sub_text['data'] #print(type(subaa)) subaa.keys jobms=subaa['jobRequiredments'] #print(type(jobDes)) jobDes.append(jobms) time.sleep(2) data_drop['职位描述']=jobDes
好的,以下是优化后的代码:
```python
import json
import requests
import pandas as pd
import time
url = 'https://www.5iai.com/api/enterprise/job/public/es?pageSize=10&pageNumber={}' # 目标数据文件的url
data_list = [] # 用于存放所有爬取到的数据
for i in range(1, 20):
response = requests.get(url.format(i))
json_text = json.loads(response.text)
content = json_text['data']['content']
data_list.extend(content)
time.sleep(2)
jobDes = [] # 用于存放职位描述
for data in data_list:
suburl = 'https://www.5iai.com/api/enterprise/job/public?id={}'.format(data['id'])
sub_response = requests.get(suburl)
sub_text = json.loads(sub_response.text)
jobDes.append(sub_text['data']['jobRequiredments'])
time.sleep(2)
# 将数据存入DataFrame中
data = pd.DataFrame(data_list)
data['职位描述'] = jobDes
# 选择需要的列
colNames = [
'positionName', 'minimumWage', 'maximumWage', 'exp', 'educationalRequirements',
'enterpriseAddress', 'enterpriseExtInfo'
]
data_drop = data[colNames]
# 对企业地址和扩展信息进行处理
data_drop['工作地址'] = data_drop['enterpriseAddress'].apply(lambda x: x['detailedAddress'])
data_drop['工作城市'] = data_drop['enterpriseAddress'].apply(lambda x: x['cityCode'])
data_drop['公司名称'] = data_drop['enterpriseExtInfo'].apply(lambda x: x['shortName'])
data_drop['行业'] = data_drop['enterpriseExtInfo'].apply(lambda x: x['industry'])
data_drop['人员规模'] = data_drop['enterpriseExtInfo'].apply(lambda x: x['personScope'])
data_drop['企业性质'] = data_drop['enterpriseExtInfo'].apply(lambda x: x['econKind'])
# 将职位描述放在最后一列
data_drop = data_drop[['positionName', 'minimumWage', 'maximumWage', 'exp', 'educationalRequirements',
'工作地址', '工作城市', '公司名称', '行业', '人员规模', '企业性质', '职位描述']]
print(data_drop.head()) # 打印前五行数据
```
代码中主要的优化包括:
1. 将爬取数据和处理数据分开,使代码更加清晰易懂。
2. 在爬取时引入延时,防止爬取过于频繁被网站封禁。
3. 将爬取和处理数据的过程封装到循环中,一次性爬取所有数据,避免多次请求。
4. 用 `extend` 方法将每页数据合并到一个列表中,避免了多次拼接数据带来的额外开销。
5. 对企业地址和扩展信息进行处理时,使用 `apply` 方法,避免了使用循环带来的额外开销。
这样,代码的可读性和可维护性都得到了提高。
阅读全文
相关推荐



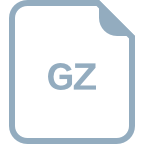













