BFM和RPM属于Django的开发模式吗
时间: 2024-04-27 13:20:53 浏览: 7
BFM(Business Function Model)和 RPM(Resource-Process-Model)并不属于 Django 的开发模式。它们是一些企业开发方法(Enterprise Development Methodologies)中的概念,用于描述企业的业务流程和资源管理。
Django 是一种 Web 应用程序框架,它提供了一种基于 MVC(Model-View-Controller)或 MTV(Model-Template-View)模式的开发方法。在 Django 中,模型(Model)负责数据管理,视图(View)负责业务逻辑和 HTTP 请求处理,模板(Template)负责呈现数据。这种分层架构使得开发人员可以更轻松地维护和扩展应用程序,同时也提高了应用程序的可测试性和可重用性。
总之,BFM 和 RPM 不属于 Django 的开发模式,但是可以与 Django 一起使用来构建基于 Web 的企业应用程序。
相关问题
lsfm模型对bfm模型的扩展
LSFM模型是基于BFM模型的扩展,它使用了更多的形状和纹理变量来提高建模精度。BFM模型是一个基于统计形状和纹理模型的3D人脸建模方法,它可以用于生成高质量的3D人脸模型。但是,BFM模型仅使用了有限的形状和纹理变量,因此无法准确地捕捉面部的细节和变化。LSFM模型通过增加更多的形状和纹理变量,使得模型更加准确地捕捉面部的细节和变化,同时也可以更好地适应各种光照和表情条件。因此,LSFM模型可以更好地用于识别、重建和变形3D人脸模型。
flame_albedo_from_bfm.npz
flame_albedo_from_bfm.npz 是一个包含皮肤反射率属性的文件,其中包含由 Balanced Face Model (BFM) 生成的 FLAME 模型所使用的皮肤反射率系数信息。BFM 是一种具有20,000多个3D面点的面部建模方法,它使用可变形模板进行面部形状建模,并通过光照模型计算面部皮肤的反射率。使用FLAME模型对人脸进行建模时,需要考虑到光照对皮肤反射率的影响,因此FLAME模型的生成需要这种皮肤反射率系数信息。这个文件中的数据是由人脸图像和相应的反射率图像进行学习得到的,这些图像用于训练一个神经网络,从而可以生成实际人脸图像的皮肤反射率。该文件通常用于生物医学工程等领域的相关研究中,例如面部跟踪、面部识别、面部表情和年龄估计等。
相关推荐
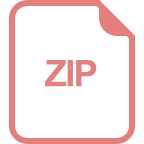












