stringtokenizer itr = new stringtokenizer(value.tostring()); while (itr.hasm
时间: 2023-09-22 15:02:42 浏览: 89
StringTokenizer itr = new StringTokenizer(value.toString());
while (itr.hasMoreTokens()) {
String token = itr.nextToken();
// 在这里添加你想要实现的逻辑
// 比如打印每个token, 存储每个token等等
}
StringTokenizer是用于分隔字符串的类。在这个例子中,我们创建了一个StringTokenizer对象,并将value.toString()作为构造函数的参数传入。然后我们使用while循环来遍历所有的token。在循环中,我们可以对每个token执行我们想要的操作,比如打印每个token的值,将每个token存储到一个List或者数组中等等。具体操作取决于你的需求。最后,当没有更多的token可用时,循环结束。
相关问题
帮我解释下面的代码:import java.io.IOException; import java.util.StringTokenizer; import org.apache.hadoop.conf.Configuration; import org.apache.hadoop.fs.Path; import org.apache.hadoop.io.IntWritable; import org.apache.hadoop.io.Text; import org.apache.hadoop.mapreduce.Job; import org.apache.hadoop.mapreduce.Mapper; import org.apache.hadoop.mapreduce.Reducer; import org.apache.hadoop.mapreduce.lib.input.FileInputFormat; import org.apache.hadoop.mapreduce.lib.output.FileOutputFormat; import org.apache.hadoop.util.GenericOptionsParser; public class WordCount { public static class TokenizerMapper extends Mapper<Object, Text, Text, IntWritable>{ private final static IntWritable one = new IntWritable(1); private Text word = new Text(); public void map(Object key, Text value, Context context ) throws IOException, InterruptedException { StringTokenizer itr = new StringTokenizer(value.toString()); while (itr.hasMoreTokens()) { word.set(itr.nextToken()); context.write(word, one); } } } public static class IntSumReducer extends Reducer<Text,IntWritable,Text,IntWritable> { private IntWritable result = new IntWritable(); public void reduce(Text key, Iterable<IntWritable> values, Context context ) throws IOException, InterruptedException { int sum = 0; for (IntWritable val : values) { sum += val.get(); } result.set(sum); context.write(key, result); } } public static void main(String[] args) throws Exception { Configuration conf = new Configuration(); String[] otherArgs = new GenericOptionsParser(conf, args).getRemainingArgs(); if (otherArgs.length != 2) { System.err.println("Usage: wordcount <in> <out>"); System.exit(2); } Job job = new Job(conf, "word count"); job.setJarByClass(WordCount.class); job.setMapperClass(TokenizerMapper.class); job.setCombinerClass(IntSumReducer.class); job.setReducerClass(IntSumReducer.class); job.setOutputKeyClass(Text.class); job.setOutputValueClass(IntWritable.class); FileInputFormat.addInputPath(job, new Path(otherArgs[0])); FileOutputFormat.setOutputPath(job, new Path(otherArgs[1])); System.exit(job.waitForCompletion(true) ? 0 : 1); } }
这段代码是一个 Hadoop MapReduce 的 WordCount(词频统计)程序,主要包括以下几个部分:
1. 引入需要用到的类库:
```
import java.io.IOException;
import java.util.StringTokenizer;
import org.apache.hadoop.conf.Configuration;
import org.apache.hadoop.fs.Path;
import org.apache.hadoop.io.IntWritable;
import org.apache.hadoop.io.Text;
import org.apache.hadoop.mapreduce.Job;
import org.apache.hadoop.mapreduce.Mapper;
import org.apache.hadoop.mapreduce.Reducer;
import org.apache.hadoop.mapreduce.lib.input.FileInputFormat;
import org.apache.hadoop.mapreduce.lib.output.FileOutputFormat;
import org.apache.hadoop.util.GenericOptionsParser;
```
2. 定义 Mapper 类:
```
public static class TokenizerMapper
extends Mapper<Object, Text, Text, IntWritable>{
private final static IntWritable one = new IntWritable(1);
private Text word = new Text();
public void map(Object key, Text value, Context context)
throws IOException, InterruptedException {
StringTokenizer itr = new StringTokenizer(value.toString());
while (itr.hasMoreTokens()) {
word.set(itr.nextToken());
context.write(word, one);
}
}
}
```
Mapper 类主要是将输入的文本分割成单词,然后对每个单词输出一个键值对,其中键为单词,值为 1。
3. 定义 Reducer 类:
```
public static class IntSumReducer
extends Reducer<Text,IntWritable,Text,IntWritable> {
private IntWritable result = new IntWritable();
public void reduce(Text key, Iterable<IntWritable> values,
Context context) throws IOException, InterruptedException {
int sum = 0;
for (IntWritable val : values) {
sum += val.get();
}
result.set(sum);
context.write(key, result);
}
}
```
Reducer 类主要是将 Mapper 输出的键值对按照键进行分组,然后对每组键值进行统计,输出每个单词出现的次数。
4. 定义主函数:
```
public static void main(String[] args) throws Exception {
Configuration conf = new Configuration();
String[] otherArgs = new GenericOptionsParser(conf, args).getRemainingArgs();
if (otherArgs.length != 2) {
System.err.println("Usage: wordcount <in> <out>");
System.exit(2);
}
Job job = new Job(conf, "word count");
job.setJarByClass(WordCount.class);
job.setMapperClass(TokenizerMapper.class);
job.setCombinerClass(IntSumReducer.class);
job.setReducerClass(IntSumReducer.class);
job.setOutputKeyClass(Text.class);
job.setOutputValueClass(IntWritable.class);
FileInputFormat.addInputPath(job, new Path(otherArgs[0]));
FileOutputFormat.setOutputPath(job, new Path(otherArgs[1]));
System.exit(job.waitForCompletion(true) ? 0 : 1);
}
```
主函数主要是设置 MapReduce 作业的相关参数,包括输入路径、输出路径、Mapper 类、Reducer 类、输出键值对类型等。最后调用 `waitForCompletion` 函数提交作业并等待作业完成。
Hadoop统计、排序、去重代码
好的,在 Hadoop 中统计、排序、去重常常使用 MapReduce 来实现。下面是一个简单的例子:
统计单词出现的次数:
```java
public static class TokenizerMapper
extends Mapper<Object, Text, Text, IntWritable>{
private final static IntWritable one = new IntWritable(1);
private Text word = new Text();
public void map(Object key, Text value, Context context
) throws IOException, InterruptedException {
StringTokenizer itr = new StringTokenizer(value.toString());
while (itr.hasMoreTokens()) {
word.set(itr.nextToken());
context.write(word, one);
}
}
}
public static class IntSumReducer
extends Reducer<Text,IntWritable,Text,IntWritable> {
private IntWritable result = new IntWritable();
public void reduce(Text key, Iterable<IntWritable> values,
Context context
) throws IOException, InterruptedException {
int sum = 0;
for (IntWritable val : values) {
sum += val.get();
}
result.set(sum);
context.write(key, result);
}
}
```
排序:
```java
public static class TokenizerMapper
extends Mapper<Object, Text, Text, IntWritable>{
private final static IntWritable one = new IntWritable(1);
private Text word = new Text();
public void map(Object key, Text value, Context context
) throws IOException, InterruptedException {
StringTokenizer itr = new StringTokenizer(value.toString());
while (itr.hasMoreTokens()) {
word.set(itr.nextToken());
context.write(word, one);
}
}
}
public static class IntSumReducer
extends Reducer<Text,IntWritable,Text,IntWritable> {
private IntWritable result = new IntWritable();
public void reduce(Text key, Iterable<IntWritable> values,
Context context
) throws IOException, InterruptedException {
int sum = 0;
for (IntWritable val : values) {
sum += val.get();
}
result.set(sum);
context.write(key, result);
}
}
public static void main(String[] args) throws Exception {
Configuration conf = new Configuration();
Job job = Job.getInstance(conf, "word count");
job.setJarByClass(WordCount.class);
job.setMapperClass(TokenizerMapper.class);
job.setCombinerClass(IntSumReducer.class);
job.setReducerClass(IntSumReducer.class);
job.setOutputKeyClass(Text.class);
job.setOutputValueClass
相关推荐
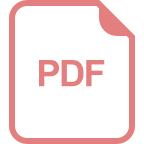
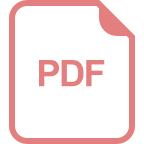












