Keras+pipelines+glove+textcnn做英文文本二分类代码
时间: 2023-08-06 09:04:24 浏览: 55
Sure! Here's an example code using Keras with pipelines, GloVe word embeddings, and TextCNN for English text classification:
```python
import numpy as np
from keras.models import Sequential
from keras.layers import Embedding, Conv1D, GlobalMaxPooling1D, Dense
from keras.preprocessing.text import Tokenizer
from keras.preprocessing.sequence import pad_sequences
from sklearn.model_selection import train_test_split
from sklearn.pipeline import Pipeline
from sklearn.feature_extraction.text import TfidfVectorizer
# Load the GloVe word embeddings
def load_glove_embeddings(embeddings_file):
embeddings_index = {}
with open(embeddings_file, encoding='utf-8') as f:
for line in f:
values = line.split()
word = values[0]
coefs = np.asarray(values[1:], dtype='float32')
embeddings_index[word] = coefs
return embeddings_index
# Define TextCNN model
def create_textcnn_model(embedding_matrix, input_length):
model = Sequential()
model.add(Embedding(input_dim=embedding_matrix.shape[0], output_dim=embedding_matrix.shape[1],
weights=[embedding_matrix], input_length=input_length, trainable=False))
model.add(Conv1D(128, kernel_size=3, activation='relu'))
model.add(GlobalMaxPooling1D())
model.add(Dense(128, activation='relu'))
model.add(Dense(1, activation='sigmoid'))
model.compile(loss='binary_crossentropy', optimizer='adam', metrics=['accuracy'])
return model
# Load the dataset
# Assuming you have a file named 'data.txt' containing text samples and labels separated by a tab character
def load_dataset(dataset_file):
texts = []
labels = []
with open(dataset_file, encoding='utf-8') as f:
for line in f:
text, label = line.strip().split('\t')
texts.append(text)
labels.append(int(label))
return texts, labels
# Set the paths and parameters
glove_file = 'path/to/glove.6B.100d.txt'
dataset_file = 'path/to/data.txt'
max_words = 10000
max_sequence_length = 100
embedding_dim = 100
# Load the GloVe word embeddings
embeddings_index = load_glove_embeddings(glove_file)
# Load the dataset
texts, labels = load_dataset(dataset_file)
# Split the dataset into training and testing sets
train_texts, test_texts, train_labels, test_labels = train_test_split(texts, labels, test_size=0.2, random_state=42)
# Tokenize the texts and convert them to sequences
tokenizer = Tokenizer(num_words=max_words)
tokenizer.fit_on_texts(train_texts)
train_sequences = tokenizer.texts_to_sequences(train_texts)
test_sequences = tokenizer.texts_to_sequences(test_texts)
# Pad the sequences to a fixed length
train_data = pad_sequences(train_sequences, maxlen=max_sequence_length)
test_data = pad_sequences(test_sequences, maxlen=max_sequence_length)
# Create the embedding matrix
word_index = tokenizer.word_index
embedding_matrix = np.zeros((len(word_index) + 1, embedding_dim))
for word, i in word_index.items():
embedding_vector = embeddings_index.get(word)
if embedding_vector is not None:
embedding_matrix[i] = embedding_vector
# Create the TextCNN model
model = create_textcnn_model(embedding_matrix, max_sequence_length)
# Train the model
model.fit(train_data, np.array(train_labels), validation_split=0.2, epochs=10, batch_size=32)
# Evaluate the model
loss, accuracy = model.evaluate(test_data, np.array(test_labels))
print(f'Test loss: {loss:.4f}')
print(f'Test accuracy: {accuracy:.4f}')
# Save the model
model.save('textcnn_model.h5')
```
Please make sure to replace `'path/to/glove.6B.100d.txt'` with the actual path to your GloVe embeddings file, and `'path/to/data.txt'` with the actual path to your dataset file.
This code uses the Keras library to define a TextCNN model for text classification. The GloVe word embeddings are loaded and used to initialize the embedding layer in the model. The dataset is loaded, tokenized, and padded to a fixed length. The model is then trained and evaluated on the dataset.
Note: This code assumes that you have already installed the required dependencies (Keras, NumPy, scikit-learn).
相关推荐
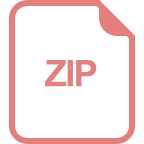
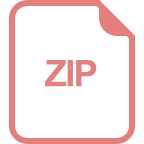














