pycharm python load 读入绝对路径
时间: 2023-08-22 14:10:29 浏览: 50
在PyCharm中,你可以使用Python的内置函数`open`来读取绝对路径下的文件。下面是一个示例代码:
```python
file_path = '/absolute/path/to/file.txt'
with open(file_path, 'r') as file:
content = file.read()
print(content)
```
在上面的代码中,`file_path`是文件的绝对路径。使用`open`函数打开文件,并指定模式为`'r'`,这表示以只读方式打开文件。然后可以使用`read`方法读取文件内容并将其存储在变量`content`中。最后,通过打印`content`可以查看文件内容。
请将`/absolute/path/to/file.txt`替换为你实际的文件路径。注意,在Windows系统中,路径分隔符为反斜杠(`\`),而在Linux和Mac系统中,路径分隔符为正斜杠(`/`)。确保提供正确的路径。
相关问题
修改pycharm中python解析器路径
可以在PyCharm的设置中修改Python解析器路径。打开PyCharm,点击“File”菜单,选择“Settings”,在弹出的窗口中选择“Project: [项目名称]”下的“Project Interpreter”,然后点击右上角的齿轮图标,选择“Add”,在弹出的窗口中选择你想要使用的Python解析器路径即可。
如何在pycharm修改python默认下载路径
在PyCharm中修改Python默认下载路径,可以按照以下步骤进行操作:
1. 打开PyCharm,进入File -> Settings菜单。
2. 在左侧的菜单中,选择Project: your_project_name -> Project Interpreter。
3. 在右侧的窗口中,找到Python解释器,点击右侧的设置按钮(齿轮图标)。
4. 在弹出的菜单中,选择Show All。
5. 选中Paths选项卡,然后在右侧的窗口中,找到下面的Downloaded Packages选项。在这个选项下,可以看到Python默认的下载路径。
6. 点击右侧的Edit按钮,然后在弹出的对话框中,输入你想要设置的新的下载路径。注意,路径必须存在,否则会报错。
7. 点击OK保存修改,然后关闭所有的设置窗口。
这样,你就可以在PyCharm中修改Python默认下载路径了。
相关推荐
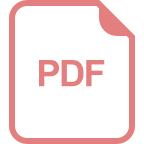
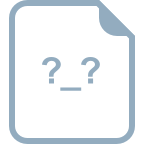












