ERROR: test_pt_common_predict (__main__.TestCommonExecutor) ---------------------------------------------------------------------- Traceback (most recent call last): File "d_warehouse/vot/z_test/z_model/cv/pt_common.py", line 54, in test_pt_common_predict Mnist(self.data_dir, man="gcgS467j").b("0001").run() File "/django_scrapy/d_warehouse/vot/base/base.py", line 357, in run return self.do_run() File "/django_scrapy/d_warehouse/vot/data/dataset/cv/mnist.py", line 34, in do_run train_df = self.sqlc.createDataFrame(train_data_list) File "/usr/local/lib/python3.8/dist-packages/pyspark/sql/context.py", line 473, in createDataFrame return self.sparkSession.createDataFrame( # type: ignore[call-overload] File "/usr/local/lib/python3.8/dist-packages/pyspark/sql/session.py", line 1222, in createDataFrame self._jvm.SparkSession.setActiveSession(self._jsparkSession) File "/usr/local/lib/python3.8/dist-packages/py4j/java_gateway.py", line 1712, in __getattr__ answer = self._gateway_client.send_command( File "/usr/local/lib/python3.8/dist-packages/py4j/java_gateway.py", line 1036, in send_command connection = self._get_connection() File "/usr/local/lib/python3.8/dist-packages/py4j/clientserver.py", line 284, in _get_connection connection = self._create_new_connection() File "/usr/local/lib/python3.8/dist-packages/py4j/clientserver.py", line 291, in _create_new_connection connection.connect_to_java_server() File "/usr/local/lib/python3.8/dist-packages/py4j/clientserver.py", line 438, in connect_to_java_server self.socket.connect((self.java_address, self.java_port)) ConnectionRefusedError: [Errno 111] Connection refused
时间: 2023-12-14 10:02:37 浏览: 35
这个错误表明你的代码尝试连接到一个 Java 服务器,但连接被拒绝了。可能有几个原因导致这个问题:
1. Java 服务器没有正确启动或正在运行。请确保你的 Java 服务器正在运行,并且监听的端口没有被其他进程占用。
2. 防火墙或网络配置问题。防火墙或网络配置可能会阻止你的代码与 Java 服务器建立连接。请检查防火墙设置并确保网络配置正确。
3. IP 地址或端口号错误。请确保你提供给代码的 Java 服务器的 IP 地址和端口号是正确的。
请仔细检查上述原因,并根据实际情况进行相应的调整。如果问题仍然存在,请提供更多的代码和错误上下文,以便进一步帮助你解决问题。
相关问题
calibration_curve c-index python
calibration_curve是一个在机器学习中可用于评估分类模型校准性的函数,它可以帮助我们了解模型的预测概率与实际概率之间的偏差。而c-index是一个用于度量模型预测排序能力的评价指标。在Python中,我们可以使用scikit-learn库中的相关函数和工具进行处理。
首先,我们需要导入scikit-learn库中的calibration_curve和metrics模块:
```python
from sklearn.calibration import calibration_curve
from sklearn import metrics
```
接下来,我们可以使用calibration_curve函数来计算校准曲线的数据,同时传入模型的预测概率和真实标签:
```python
probs = model.predict_proba(X_test)[:, 1]
true_label = y_test
fraction_of_positives, mean_predicted_value = calibration_curve(true_label, probs, n_bins=10)
```
在这个例子中,我们使用X_test作为测试数据集的特征,y_test作为测试数据集的真实标签。model是我们训练好的分类模型。
接着,我们可以使用matplotlib库来绘制校准曲线:
```python
import matplotlib.pyplot as plt
plt.plot(mean_predicted_value, fraction_of_positives, 's-', label='Calibration curve')
plt.plot([0, 1], [0, 1], '--', color='gray', label='Perfectly calibrated')
plt.xlabel('Mean predicted value')
plt.ylabel('Fraction of positives')
plt.legend()
plt.title('Calibration curve')
plt.show()
```
最后,我们可以使用metrics模块中的c-index函数来计算c-index:
```python
c_index = metrics.roc_auc_score(true_label, probs)
```
这样,我们就可以通过使用calibration_curve函数来绘制校准曲线,并使用c-index函数来计算模型的c-index值,从而对模型的预测效果进行评估。
klearn 文本分类_详细解析scikit-learn进行文本分类
Scikit-learn是一个Python的机器学习库,提供了丰富的机器学习算法和工具。其中,文本分类是机器学习中一个重要的应用场景,而Scikit-learn也提供了许多文本分类的工具和算法。
下面详细解析如何使用Scikit-learn进行文本分类。
1. 数据准备
首先需要准备好文本数据,可以从文本文件中读取,也可以直接使用Python字符串。
对于分类问题,需要将文本数据与对应的标签组成二元组。例如:
```
data = [("This is a positive sentence", "positive"),
("I am happy today", "positive"),
("This is a negative sentence", "negative"),
("I am sad today", "negative")]
```
2. 特征提取
在文本分类中,通常需要将文本数据转换为数值特征,以便机器学习算法进行处理。常用的特征提取方法有:
- 词袋模型(Bag-of-Words):将文本表示为一个词汇表中单词的计数向量。
- TF-IDF模型:词频-逆文档频率(Term Frequency-Inverse Document Frequency),旨在衡量一个单词在文档中的重要程度。
- Word2Vec模型:将单词转换为密集向量表示。
在Scikit-learn中,可以使用CountVectorizer和TfidfVectorizer进行特征提取。
```
from sklearn.feature_extraction.text import CountVectorizer, TfidfVectorizer
# 词袋模型
vectorizer = CountVectorizer()
X = vectorizer.fit_transform([d[0] for d in data])
# TF-IDF模型
vectorizer = TfidfVectorizer()
X = vectorizer.fit_transform([d[0] for d in data])
```
3. 模型训练
在特征提取后,可以使用Scikit-learn提供的机器学习算法进行模型训练。常用的分类算法有:
- 朴素贝叶斯(Naive Bayes)
- 决策树(Decision Tree)
- 支持向量机(Support Vector Machine)
- 随机森林(Random Forest)
以朴素贝叶斯分类器为例:
```
from sklearn.naive_bayes import MultinomialNB
from sklearn.model_selection import train_test_split
# 划分训练集和测试集
X_train, X_test, y_train, y_test = train_test_split(X, [d[1] for d in data], test_size=0.2)
# 训练朴素贝叶斯分类器
clf = MultinomialNB()
clf.fit(X_train, y_train)
```
4. 模型评估
训练完成后,需要对模型进行评估。常用的评估指标有:
- 准确率(Accuracy)
- 精确率(Precision)
- 召回率(Recall)
- F1-score
以准确率为例:
```
from sklearn.metrics import accuracy_score
# 在测试集上评估模型
y_pred = clf.predict(X_test)
accuracy = accuracy_score(y_test, y_pred)
print("Accuracy:", accuracy)
```
5. 新数据预测
最后,可以使用训练好的模型对新数据进行预测。
```
# 对新数据进行预测
new_data = ["I am not sure how I feel", "This sentence is neutral"]
X_new = vectorizer.transform(new_data)
y_pred = clf.predict(X_new)
print(y_pred)
```
以上就是使用Scikit-learn进行文本分类的详细解析。
相关推荐
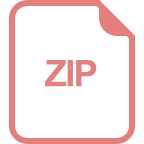













