为我提供TPR、FPR、K1、ROC、AUC、KS、GAIN、LIFT、GINI、KSI的函数代码
时间: 2024-06-17 09:05:40 浏览: 15
这些指标函数的代码可以在不同的编程语言中实现,下面是Python中的代码示例:
1. TPR (True Positive Rate) 和 FPR (False Positive Rate):
```python
from sklearn.metrics import confusion_matrix
def tpr_fpr(y_true, y_pred):
tn, fp, fn, tp = confusion_matrix(y_true, y_pred).ravel()
tpr = tp / (tp + fn)
fpr = fp / (fp + tn)
return tpr, fpr
```
2. K1 (Kappa):
```python
from sklearn.metrics import cohen_kappa_score
def kappa(y_true, y_pred):
return cohen_kappa_score(y_true, y_pred)
```
3. ROC (Receiver Operating Characteristic) 和 AUC (Area Under the Curve):
```python
from sklearn.metrics import roc_curve, auc
def roc_auc(y_true, y_pred_prob):
fpr, tpr, thresholds = roc_curve(y_true, y_pred_prob)
roc_auc = auc(fpr, tpr)
return roc_auc
```
4. KS (Kolmogorov-Smirnov):
```python
from scipy.stats import ks_2samp
def ks(y_true, y_pred_prob):
tpr_fpr_df = pd.DataFrame({'y_true': y_true, 'y_pred_prob': y_pred_prob})
p0 = tpr_fpr_df[tpr_fpr_df.y_true == 0].y_pred_prob
p1 = tpr_fpr_df[tpr_fpr_df.y_true == 1].y_pred_prob
ks_statistic, p_value = ks_2samp(p0, p1)
return ks_statistic
```
5. GAIN:
```python
import numpy as np
def gain(y_true, y_pred_prob, n_bins=10):
df = pd.DataFrame({'y_true': y_true, 'y_pred_prob': y_pred_prob})
df['y_true'] = df['y_true'].astype(int)
df['n'] = 1
df['decile'] = pd.qcut(df['y_pred_prob'], n_bins)
grouped = df.groupby('decile', as_index=False)
agg_df = grouped.agg({'y_true': np.sum, 'n': np.sum})
agg_df['pct_total'] = agg_df['n'] / agg_df['n'].sum()
agg_df['pct_pos'] = agg_df['y_true'] / agg_df['y_true'].sum()
agg_df['cum_pct_total'] = agg_df['pct_total'].cumsum()
agg_df['cum_pct_pos'] = agg_df['pct_pos'].cumsum()
agg_df['cum_pct_neg'] = agg_df['cum_pct_total'] - agg_df['cum_pct_pos']
agg_df['lift'] = agg_df['cum_pct_pos'] / agg_df['pct_total'].mean()
agg_df['gain'] = agg_df['cum_pct_pos'] / agg_df['cum_pct_pos'].max()
return agg_df[['decile', 'pct_total', 'pct_pos', 'cum_pct_pos', 'cum_pct_neg', 'lift', 'gain']]
```
6. LIFT:
```python
import numpy as np
def lift(y_true, y_pred_prob, n_bins=10):
df = pd.DataFrame({'y_true': y_true, 'y_pred_prob': y_pred_prob})
df['y_true'] = df['y_true'].astype(int)
df['n'] = 1
df['decile'] = pd.qcut(df['y_pred_prob'], n_bins)
grouped = df.groupby('decile', as_index=False)
agg_df = grouped.agg({'y_true': np.sum, 'n': np.sum})
agg_df['pct_total'] = agg_df['n'] / agg_df['n'].sum()
agg_df['pct_pos'] = agg_df['y_true'] / agg_df['y_true'].sum()
agg_df['cum_pct_total'] = agg_df['pct_total'].cumsum()
agg_df['cum_pct_pos'] = agg_df['pct_pos'].cumsum()
base_pos_rate = agg_df.y_true.sum() / len(df)
lift_series = agg_df.cum_pct_pos / (agg_df.cum_pct_total * base_pos_rate)
return lift_series
```
7. GINI:
```python
from sklearn.metrics import roc_curve
def gini(y_true, y_pred_prob):
fpr, tpr, thresholds = roc_curve(y_true, y_pred_prob)
auc_score = auc(fpr, tpr)
gini_coefficient = 2 * auc_score - 1
return gini_coefficient
```
8. KSI:
```python
from scipy.stats import norm
def ksi(y_true_train, y_pred_prob_train, y_true_test, y_pred_prob_test):
mu_train, std_train = norm.fit(y_pred_prob_train)
mu_test, std_test = norm.fit(y_pred_prob_test)
cdf_train_train = norm.cdf(y_pred_prob_train, loc=mu_train, scale=std_train)
cdf_train_test = norm.cdf(y_pred_prob_test, loc=mu_train, scale=std_train)
cdf_test_test = norm.cdf(y_pred_prob_test, loc=mu_test, scale=std_test)
ksi_train = np.abs((cdf_train_train - cdf_train_test).mean())
ksi_test = np.abs((cdf_test_test - cdf_train_test).mean())
return ksi_train, ksi_test
```
相关推荐
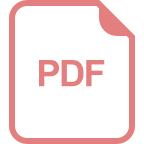
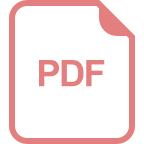
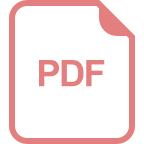











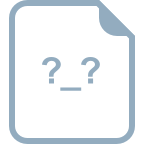
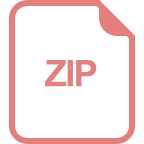
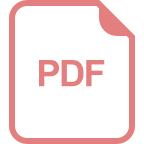
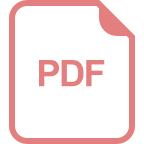