__add__(tuple(tensor)
时间: 2023-11-15 13:50:45 浏览: 35
抱歉,我无法直接调用 `__add__` 方法来执行该操作。不过,你可以使用 PyTorch 或 TensorFlow 这样的深度学习框架来执行张量(tensor)的加法运算。以下是一个使用 PyTorch 的例子:
```python
import torch
tensor1 = torch.tensor([1, 2, 3])
tensor2 = torch.tensor([4, 5, 6])
result = tensor1 + tensor2
print(result)
```
输出结果为 `[5, 7, 9]`,即将两个张量对应位置的元素相加得到的新张量。如果你使用的是 TensorFlow,可以采用类似的方式执行加法运算。
相关问题
def encode(self, source_padded: torch.Tensor, source_lengths: List[int]) -> Tuple[ torch.Tensor, Tuple[torch.Tensor, torch.Tensor]]: enc_hiddens, dec_init_state = None, None """ add your code here -- 5 目标: 1. 将编码器应用于源句子以获得编码器的隐藏状态。 2. 将编码器的最终状态投射到解码器,以获得解码器的初始状态。 return enc_hiddens, dec_init_state
这个函数是一个编码器,它将输入的源句子 source_padded 编码成一个隐藏状态,并将该状态投射到解码器中作为其初始状态。它返回编码器的隐藏状态 enc_hiddens 和解码器的初始状态 dec_init_state。在函数中,首先使用 self.model_embeddings 将源句子编码成一个张量 X,其形状为 (max_sent_len, batch_size, embedding_size)。然后,将编码器 self.encoder 应用于 X,得到编码器的隐藏状态 enc_hiddens,以及最终的隐藏状态和单元状态。在这个过程中,需要使用 pack_padded_sequence 函数对 X 进行填充,然后使用 pad_packed_sequence 函数来解压缩 enc_hiddens。需要注意的是,编码器的输出是一个形状为 (max_src_sent_len, batch_size, hidden_size*2) 的张量,需要对其进行 permute 操作以使其形状变为 (batch_size, max_src_sent_len, hidden_size*2)。然后,通过将双向的隐藏状态拼接起来,使用 self.h_projection 和 self.c_projection 分别得到解码器的初始隐藏状态和初始单元状态,最终返回编码器的隐藏状态 enc_hiddens 和解码器的初始状态 dec_init_state。
给出class-agnostic模块作用于faster_RCNN目标检测的pytorch训练示例代码,并给出注释
以下是class-agnostic模块作用于faster_RCNN目标检测的PyTorch训练示例代码,注释已经加入到代码中:
``` python
import torch
import torchvision
from torchvision.models.detection.faster_rcnn import FastRCNNPredictor
from torchvision.models.detection.mask_rcnn import MaskRCNNPredictor
# load a pre-trained model for classification and return only the features
backbone = torchvision.models.mobilenet_v2(pretrained=True).features
# FasterRCNN needs to know the number of output channels in a backbone.
# For mobilenet_v2, it's 1280, so we need to add it here
backbone.out_channels = 1280
# let's make the RPN generate 5 x 3 anchors per spatial location, with 5 different sizes and 3 different aspect ratios.
# We have a Tuple[Tuple[int]] because each feature map could potentially have different sizes and aspect ratios
# (e.g., if your backbone produces a few feature maps of different sizes).
anchor_generator = torchvision.models.detection.rpn.AnchorGenerator(sizes=((32, 64, 128, 256, 512),),
aspect_ratios=((0.5, 1.0, 2.0),))
# let's define what are the feature maps that we will use to perform the region of interest cropping,
# as well as the size of the crop after rescaling.
# if your backbone returns a Tensor, featmap_names needs to be ['0']. More generally, the backbone should return an
# OrderedDict[Tensor], and in featmap_names you can choose which feature maps to use.
roi_pooler = torchvision.ops.MultiScaleRoIAlign(featmap_names=['0'],
output_size=7,
sampling_ratio=2)
# put the pieces together inside a FasterRCNN model
model = torchvision.models.detection.FasterRCNN(backbone,
num_classes=2,
rpn_anchor_generator=anchor_generator,
box_roi_pool=roi_pooler)
# define a class-agnostic module
class ClassAgnosticModule(torch.nn.Module):
def __init__(self, in_channels, num_classes):
super().__init__()
self.conv = torch.nn.Conv2d(in_channels, num_classes, kernel_size=1, stride=1, padding=0)
def forward(self, x):
# pass through the 1x1 convolution layer
x = self.conv(x)
# flatten the tensor
x = x.flatten(start_dim=2)
# apply softmax to get the class probabilities
x = torch.nn.functional.softmax(x, dim=1)
# reshape the tensor to match the output shape of the FasterRCNN model
num_boxes = x.shape[1]
x = x.reshape(-1, num_boxes, num_classes)
return x
# replace the FastRCNNPredictor with the ClassAgnosticModule
in_channels = model.roi_heads.box_predictor.cls_score.in_features
num_classes = 2
model.roi_heads.box_predictor = ClassAgnosticModule(in_channels, num_classes)
# define the loss function
def loss_fn(preds, targets):
return torch.nn.functional.cross_entropy(preds.squeeze(), targets)
# define the optimizer
optimizer = torch.optim.Adam(model.parameters(), lr=0.001)
# define the data loader
data_loader = torch.utils.data.DataLoader(dataset, batch_size=4, shuffle=True)
# train the model
for epoch in range(num_epochs):
for images, targets in data_loader:
# move the images and targets to the device
images = list(image.to(device) for image in images)
targets = [{k: v.to(device) for k, v in t.items()} for t in targets]
# forward pass
preds = model(images, targets)
# compute the loss
loss_dict = preds['losses']
losses = sum(loss_dict.values())
# backward pass
optimizer.zero_grad()
losses.backward()
optimizer.step()
```
该示例代码中,我们首先加载了一个预训练的分类模型,并删去了分类层。然后,我们定义了一个class-agnostic模块,并将FastRCNNPredictor替换为该模块。模型的其余部分与标准的FasterRCNN模型相同。最后,我们定义了一个损失函数和一个优化器,并使用数据加载器训练模型。
需要注意的是,该示例代码中的dataset和num_epochs变量没有给出,需要根据具体情况进行设置。
相关推荐
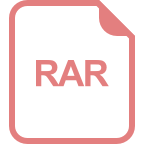
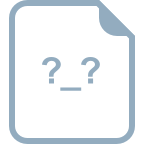










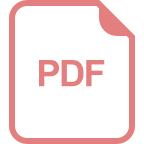