class MyBertModel(nn.Module): def __init__(self, config): super(MyBertModel, self).__init__() self.bert = BertModel.from_pretrained(config.bert_path) for param in self.bert.parameters(): param.requires_grad = True self.fc = nn.Linear(config.hidden_size, config.num_classes) def forward(self, x): context = x[0] # 输入的句子 mask = x[2] # 对padding部分进行mask,和句子一个size,padding部分用0表示,如:[1, 1, 1, 1, 0, 0] _, pooled = self.bert(context, attention_mask=mask, output_all_encoded_layers=False) out = self.fc(pooled) return out
时间: 2024-04-28 16:25:32 浏览: 120
这是一个 PyTorch 的模型类,名为 `MyBertModel`,继承自 `nn.Module`。这个模型使用了预训练的 BERT 模型,通过 `BertModel.from_pretrained(config.bert_path)` 加载预训练模型。然后,使用一个全连接层 `nn.Linear` 将 BERT 的输出映射到指定的类别个数上,即 `config.num_classes`。
在 forward 方法中,输入 `x` 是一个元组,包含三个张量:句子的 token id 序列 `context`,对应的 token 序列长度 `seq_len`,以及 padding 部分的掩码 `mask`。使用 BERT 模型对 `context` 序列进行编码,得到输出 `pooled`。最后,将 `pooled` 传入全连接层 `self.fc` 得到输出结果 `out`,并返回。
相关问题
class EntityRankerClassifier(nn.Module): def __init__(self, n_classes, PRE_TRAINED_MODEL_NAME): super(EntityRankerClassifier, self).__init__() self.bert = AutoModel.from_pretrained(PRE_TRAINED_MODEL_NAME) self.drop = nn.Dropout(p=0.3) self.out = nn.Linear(self.bert.config.hidden_size, n_classes) def forward(self, input_ids, attention_mask): _, pooled_output = self.bert( input_ids=input_ids, attention_mask=attention_mask, return_dict=False ) output = self.drop(pooled_output) return self.out(output)
这是一个使用预训练的BERT模型进行分类的PyTorch模型,具体来说,该模型包含以下几个部分:
1. 初始化函数:接受两个参数,一个是输出类别数n_classes,一个是预训练模型的名称PRE_TRAINED_MODEL_NAME,通过调用父类初始化函数来初始化模型。
2. 模型结构:该模型使用预训练的BERT模型作为编码器,通过AutoModel.from_pretrained函数加载预训练模型,并将输入的文本序列input_ids和注意力掩码attention_mask传入BERT模型中,得到BERT模型的输出。这里使用的是BERT模型的池化输出pooled_output,即将所有单词的输出取平均得到的一维向量,作为文本的表示。接着通过一个Dropout层进行正则化,最后通过一个全连接层进行分类。
3. 前向传播函数:接受两个参数,一个是文本序列input_ids,一个是注意力掩码attention_mask,将这两个参数传入模型结构中,得到模型的输出。
需要注意的是,在使用该模型进行分类时,需要将输入的文本序列转换为对应的input_ids和attention_mask,可以使用tokenizer将文本序列转换为模型所需的格式。在训练模型时,需要使用交叉熵损失函数和优化器对模型进行训练。
class Model(nn.Module): def __init__(self, config): super(Model, self).__init__() if config.embedding_pretrained is not None: self.embedding = nn.Embedding.from_pretrained(config.embedding_pretrained, freeze=False) else: self.embedding = nn.Embedding(config.n_vocab, config.embed, padding_idx=config.n_vocab - 1) self.lstm = nn.LSTM(config.embed, config.hidden_size, config.num_layers, bidirectional=True, batch_first=True, dropout=config.dropout) self.fc = nn.Linear(config.hidden_size * 2, config.num_classes) def forward(self, x): x, _ = x out = self.embedding(x) # [batch_size, seq_len, embeding]=[128, 32, 300] out, _ = self.lstm(out) out = self.fc(out[:, -1, :]) # 句子最后时刻的 hidden state return out
这段代码是一个基于 LSTM 的文本分类模型的实现,模型的输入是一个由文本数据构成的批次(batch),输出是这个批次中每个文本数据的分类结果。在该模型中,首先根据配置信息创建了一个 Embedding 层,用于将每个词汇映射为一个向量。如果配置信息中指定了预训练的 Embedding 权重,则使用预训练的权重;否则,随机初始化 Embedding 权重。接下来,将 Embedding 层的输出输入到一个多层 LSTM 网络中进行处理,得到每个文本数据的最后一个时刻上的 hidden state。最后,将这些 hidden state 输入到一个全连接层中进行分类,得到分类结果。在 forward 函数中,输入数据 x 是一个元组,其中第一个元素是输入数据,第二个元素是序列长度,但是在本模型中并没有用到序列长度,只用到了输入数据。在 forward 函数中,首先将输入数据输入到 Embedding 层中,得到词向量表达;然后将词向量表达输入到 LSTM 网络中进行处理,得到每个文本数据的 hidden state;最后,将 hidden state 输入到全连接层中进行分类,得到分类结果。
阅读全文
相关推荐









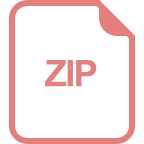
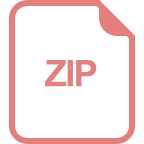