import tensorflow as tf a = tf.constant([[1,2,3,4,5], [2,3,4,5,6]], dtype=tf.float32) indices = tf.constant([[1, 0, 1, 0, 0], [0, 1, 0, 0, 0]], dtype = tf.int32) result = tf.where(tf.cast(indices, dtype=tf.bool), tf.nn.sigmoid(a), a) with tf.Session() as sess: print(sess.run(result))
时间: 2023-09-18 22:11:23 浏览: 81
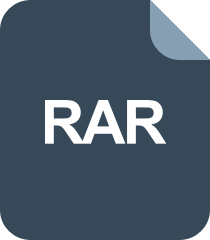
TensorFlow初学者教程和示例(支持 TF v1 和 v2).rar
这段代码使用 TensorFlow 定义了一个二维张量 `a` 和一个与之对应的二维索引张量 `indices`。然后,使用 TensorFlow 提供的 `tf.where` 方法,将 `a` 张量中对应 `indices` 张量中为 1 的位置的值应用 sigmoid 函数,其他位置的值不变,最终生成一个新的张量 `result`。
具体来说,`tf.cast(indices, dtype=tf.bool)` 将 `indices` 张量中的值转换为布尔类型,即将 1 转换为 True,将 0 转换为 False。`tf.where` 使用这个布尔类型的掩码来决定是否应用 sigmoid 函数。对于 `indices` 张量中为 1 的位置,`tf.where` 返回 `tf.nn.sigmoid(a)`,即对应位置的 `a` 张量的值应用 sigmoid 函数;对于 `indices` 张量中为 0 的位置,`tf.where` 返回 `a` 张量中对应的原始值。
最后,使用 TensorFlow Session 执行这个计算图,并打印 `result` 张量的值。
阅读全文
相关推荐
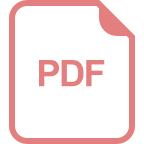
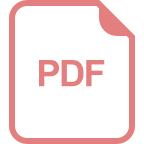














