pytorchcnn猫狗分类代码和数据集
时间: 2023-10-28 07:02:52 浏览: 90
PyTorch是一种流行的深度学习框架,可以用于构建卷积神经网络(CNN)等模型。在猫狗分类任务中,我们可以使用PyTorch来训练一个CNN模型来对猫和狗的图像进行分类。
首先,我们需要准备一个猫狗分类的数据集。可以在网上找到已经标注好的猫狗图像数据集,例如Kaggle上的猫狗大战数据集。这个数据集包含了数千张猫和狗的图像,以及它们对应的标签。
接下来,我们需要导入必要的PyTorch库和模块,例如torch、torchvision等。
然后,我们需要定义一个CNN模型。可以使用PyTorch提供的nn模块来搭建一个简单的CNN网络,包括卷积层、池化层和全连接层等。可以根据具体任务的需求和网络结构进行调整。
在搭建好网络之后,我们需要定义损失函数和优化器。对于猫狗分类任务,可以使用交叉熵损失函数来衡量预测结果和真实标签的差异,并选择适当的优化器,如SGD、Adam等来更新模型的参数。
接下来,我们可以开始训练模型。将数据集分为训练集和测试集,使用训练集来迭代地更新模型参数,计算损失函数并通过反向传播算法更新模型。在每个epoch结束后,使用测试集来评估模型的性能,如准确率、精确率、召回率等。
最后,我们可以使用训练好的模型对新的猫狗图像进行分类预测。将图像传入模型中,得到对应的预测结果,即猫或狗的标签。
总结来说,PyTorch可以用于搭建CNN模型进行猫狗分类任务。需要准备好猫狗分类的数据集,在训练过程中使用损失函数和优化器来更新模型参数,并使用测试集来评估模型性能。最终可以使用训练好的模型对新的猫狗图像进行分类预测。
相关问题
pytorch实现猫狗分类代码
以下是使用 PyTorch 实现猫狗分类的代码:
```python
import torch
import torch.nn as nn
import torch.optim as optim
from torch.optim import lr_scheduler
import numpy as np
import torchvision
from torchvision import datasets, models, transforms
import matplotlib.pyplot as plt
import time
import os
import copy
# 设置数据路径
data_dir = "./data"
# 数据预处理
data_transforms = {
'train': transforms.Compose([
transforms.RandomResizedCrop(224),
transforms.RandomHorizontalFlip(),
transforms.ToTensor(),
transforms.Normalize([0.485, 0.456, 0.406], [0.229, 0.224, 0.225])
]),
'val': transforms.Compose([
transforms.Resize(256),
transforms.CenterCrop(224),
transforms.ToTensor(),
transforms.Normalize([0.485, 0.456, 0.406], [0.229, 0.224, 0.225])
]),
}
# 加载数据
image_datasets = {x: datasets.ImageFolder(os.path.join(data_dir, x), data_transforms[x]) for x in ['train', 'val']}
dataloaders = {x: torch.utils.data.DataLoader(image_datasets[x], batch_size=4, shuffle=True, num_workers=4) for x in ['train', 'val']}
dataset_sizes = {x: len(image_datasets[x]) for x in ['train', 'val']}
class_names = image_datasets['train'].classes
# GPU 是否可用
device = torch.device("cuda:0" if torch.cuda.is_available() else "cpu")
# 可视化部分图片
def imshow(inp, title=None):
inp = inp.numpy().transpose((1, 2, 0))
mean = np.array([0.485, 0.456, 0.406])
std = np.array([0.229, 0.224, 0.225])
inp = std * inp + mean
inp = np.clip(inp, 0, 1)
plt.imshow(inp)
if title is not None:
plt.title(title)
plt.pause(0.001)
# 获取一批训练数据
inputs, classes = next(iter(dataloaders['train']))
# 显示这批图片
out = torchvision.utils.make_grid(inputs)
imshow(out, title=[class_names[x] for x in classes])
# 定义训练模型的函数
def train_model(model, criterion, optimizer, scheduler, num_epochs=25):
since = time.time()
best_model_wts = copy.deepcopy(model.state_dict())
best_acc = 0.0
for epoch in range(num_epochs):
print('Epoch {}/{}'.format(epoch, num_epochs - 1))
print('-' * 10)
# 每个 epoch 都有训练和验证阶段
for phase in ['train', 'val']:
if phase == 'train':
model.train() # 训练模式
else:
model.eval() # 验证模式
running_loss = 0.0
running_corrects = 0
# 迭代数据
for inputs, labels in dataloaders[phase]:
inputs = inputs.to(device)
labels = labels.to(device)
# 零参数梯度
optimizer.zero_grad()
# 前向
# 训练阶段跟踪历史以进行反向传播
with torch.set_grad_enabled(phase == 'train'):
outputs = model(inputs)
_, preds = torch.max(outputs, 1)
loss = criterion(outputs, labels)
# 后向 + 仅在训练阶段进行优化
if phase == 'train':
loss.backward()
optimizer.step()
# 统计
running_loss += loss.item() * inputs.size(0)
running_corrects += torch.sum(preds == labels.data)
if phase == 'train':
scheduler.step()
# 计算训练和验证的损失和精度
epoch_loss = running_loss / dataset_sizes[phase]
epoch_acc = running_corrects.double() / dataset_sizes[phase]
print('{} Loss: {:.4f} Acc: {:.4f}'.format(phase, epoch_loss, epoch_acc))
# 深度复制模型
if phase == 'val' and epoch_acc > best_acc:
best_acc = epoch_acc
best_model_wts = copy.deepcopy(model.state_dict())
print()
time_elapsed = time.time() - since
print('Training complete in {:.0f}m {:.0f}s'.format(time_elapsed // 60, time_elapsed % 60))
print('Best val Acc: {:4f}'.format(best_acc))
# 加载最佳模型权重
model.load_state_dict(best_model_wts)
return model
# 定义模型
model_ft = models.resnet18(pretrained=True)
num_ftrs = model_ft.fc.in_features
model_ft.fc = nn.Linear(num_ftrs, 2)
model_ft = model_ft.to(device)
criterion = nn.CrossEntropyLoss()
# 优化器
optimizer_ft = optim.SGD(model_ft.parameters(), lr=0.001, momentum=0.9)
# 学习率衰减
exp_lr_scheduler = lr_scheduler.StepLR(optimizer_ft, step_size=7, gamma=0.1)
# 训练模型
model_ft = train_model(model_ft, criterion, optimizer_ft, exp_lr_scheduler, num_epochs=25)
# 可视化模型的预测结果
def visualize_model(model, num_images=6):
was_training = model.training
model.eval()
images_so_far = 0
fig = plt.figure()
with torch.no_grad():
for i, (inputs, labels) in enumerate(dataloaders['val']):
inputs = inputs.to(device)
labels = labels.to(device)
outputs = model(inputs)
_, preds = torch.max(outputs, 1)
for j in range(inputs.size()[0]):
images_so_far += 1
ax = plt.subplot(num_images // 2, 2, images_so_far)
ax.axis('off')
ax.set_title('predicted: {}'.format(class_names[preds[j]]))
imshow(inputs.cpu().data[j])
if images_so_far == num_images:
model.train(mode=was_training)
return
model.train(mode=was_training)
visualize_model(model_ft)
plt.show()
```
这个代码使用了 ResNet-18 模型,对数据进行了数据增强和标准化,使用了交叉熵损失函数和学习率衰减。可以根据实际情况进行修改。
基于pytorch实现猫狗分类测试代码
以下是使用PyTorch实现猫狗分类的测试代码:
```python
import torch
import torch.nn as nn
import torchvision.models as models
import torchvision.transforms as transforms
from PIL import Image
# 加载模型
model = models.resnet18(pretrained=True)
num_ftrs = model.fc.in_features
model.fc = nn.Linear(num_ftrs, 2)
model.load_state_dict(torch.load('model.pth'))
model.eval()
# 定义图像预处理函数
transform = transforms.Compose([
transforms.Resize(256),
transforms.CenterCrop(224),
transforms.ToTensor(),
transforms.Normalize(mean=[0.485, 0.456, 0.406],
std=[0.229, 0.224, 0.225])
])
# 加载测试图片
img_path = 'test.jpg'
img = Image.open(img_path)
# 预处理图片并转换为模型输入
img_tensor = transform(img)
img_tensor = img_tensor.unsqueeze(0)
# 使用模型进行预测
with torch.no_grad():
output = model(img_tensor)
_, predicted = torch.max(output, 1)
# 打印预测结果
if predicted.item() == 0:
print('猫')
else:
print('狗')
```
其中,`model.pth`是训练好的模型文件,`test.jpg`是待测试的图片文件。在运行代码前需要先安装PyTorch和Pillow库。
相关推荐
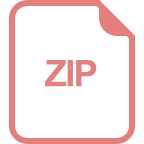












