python中要对csv文件中出现的np.inf和np.NAN如何删除,代码如何
时间: 2024-02-15 12:02:29 浏览: 28
你可以使用 pandas 库来读取 csv 文件,并通过 replace 和 dropna 方法删除 np.inf 和 np.nan。以下是代码示例:
```python
import pandas as pd
import numpy as np
# 读取 csv 文件
df = pd.read_csv('filename.csv')
# 将 np.inf 替换为 NaN
df.replace([np.inf, -np.inf], np.nan, inplace=True)
# 删除包含 NaN 的行
df.dropna(inplace=True)
# 保存结果
df.to_csv('new_filename.csv', index=False)
```
这段代码会将原始 csv 文件中的 np.inf 替换为 NaN,然后删除包含 NaN 的行,并将结果保存为一个新的 csv 文件。请将 `filename.csv` 替换为你自己的文件名,注意文件路径的正确性。
相关问题
python中要对数据中出现的np.inf和np.NAN如何删除,代码如何
可以使用NumPy库中的`numpy.isfinite()`函数来判断哪些元素是有限的,然后再使用布尔索引来删除含有`np.inf`和`np.NAN`的元素。
下面是一个示例代码:
```python
import numpy as np
# 创建一个包含np.inf和np.NAN的数组
arr = np.array([1, 2, np.inf, 4, np.NAN, 6])
# 判断哪些元素是有限的
mask = np.isfinite(arr)
# 使用布尔索引来删除含有np.inf和np.NAN的元素
filtered_arr = arr[mask]
print(filtered_arr)
```
输出结果为:
```
[1. 2. 4. 6.]
```
在上面的例子中,我们使用`numpy.isfinite()`函数来判断哪些元素是有限的,然后使用布尔索引来删除含有`np.inf`和`np.NAN`的元素,并将结果存储在`filtered_arr`中。
NaN和np.inf是一个概念嘛
NaN(Not a Number)和np.inf(无穷大)是数值计算中的特殊概念,但它们并不相同。
NaN 是一种特殊的数值,表示非数字或未定义的数值。它通常用于表示缺失数据、计算错误或无法表示的数值结果。在Python中,NaN 是通过浮点数类型的`np.nan`或`float('nan')`来表示。
而 np.inf 表示无穷大,它是一个正无穷大的特殊值。在数学中,无穷大表示一个值无限大,没有上界。在Python中,np.inf 是通过浮点数类型的`np.inf`或`float('inf')`来表示。
尽管 NaN 和 np.inf 都代表特殊的数值,但它们的含义和用法是不同的。NaN 表示非数字,而 np.inf 表示无穷大。在数值计算中,我们可以使用这些特殊值来处理缺失数据,异常情况或进行数值运算。
相关推荐
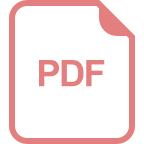
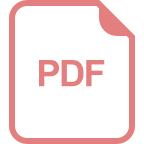












